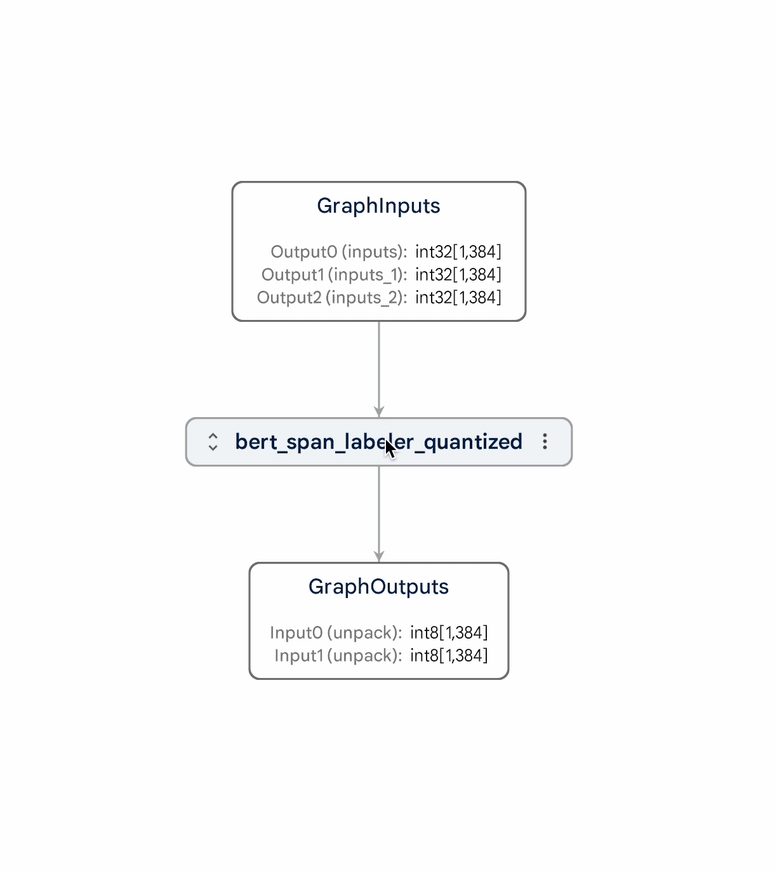
Blog test: Thumbnail gif
November 23, 2024
John Quinn, Software Engineer, Google Research
Update • sep 25, 2024
Lorem ipsum dolor sit amet, consectetur adipiscing elit, sed do eiusmod temporincididunt ut labore et dolore magnanostrud
exercitation ullamco laboris nisi ut aliquip ex ea commodo consequat.
Lorem ipsum dolor sitamet, consectetur adipiscing elit, sedminim veniam
, quis nostrud exercitation ullamco laboris nisi ut aliquip ex ea commodo consequat.
Quick links
This is an optional heading at the top of a RTE set as an H2
Lorem ipsum dolor sit amet, consectetur adipiscing elit, sed do eiusmod temporincididunt ut labore et dolore magna aliqua. Ut enimad minim veniam, quis nostrud
exercitation ullamco laboris nisi ut aliquip ex ea commodo consequat
This is a heading set to H2
By using WeightedSums as the inputs to other operators, it is possible to express rich combinatorial structures, while keeping models compact and the number of learnable weights small. As an example, we include the sum_of_products
model (illustrated in the figure below) which first creates a pairwise product of two WeightedSums
, and then a sum of the two products. By setting some of the weights to zero, we can create many different discrete structures. The total number of possible structures in this model is 216, since there are 216 base kernels that can be turned on or off. All these structures are explored implicitly by training just this one model.
This is a heading set to H3
Lorem ipsum dolor sit amet, consectetur adipiscing elit, sed do eiusmod temporincididunt ut labore et dolore magna aliqua. Ut enimad minim veniam, quis nostrud exercitation ullamco laboris nisi ut aliquip ex ea commodo consequat.
This is a heading set to H4
Lorem ipsum dolor sit amet, consectetur adipiscing elit, sed do eiusmod temporincididunt ut labore et dolore magna aliqua. Ut enimad minim veniam, quis nostrud exercitation ullamco laboris nisi ut aliquip ex ea commodo consequat.
This is an optional heading set to an H2 above Dynamic Media
Lorem ipsum dolor sit amet, consectetur adipiscing elit, sed do eiusmod temporincididunt ut labore et dolore magna aliqua. Ut enimad minim veniam, quis nostrud exercitation ullamco laboris nisi ut aliquip ex ea commodo consequat.
This is a caption alignment left.
This is an optional heading set to H3 above a media component
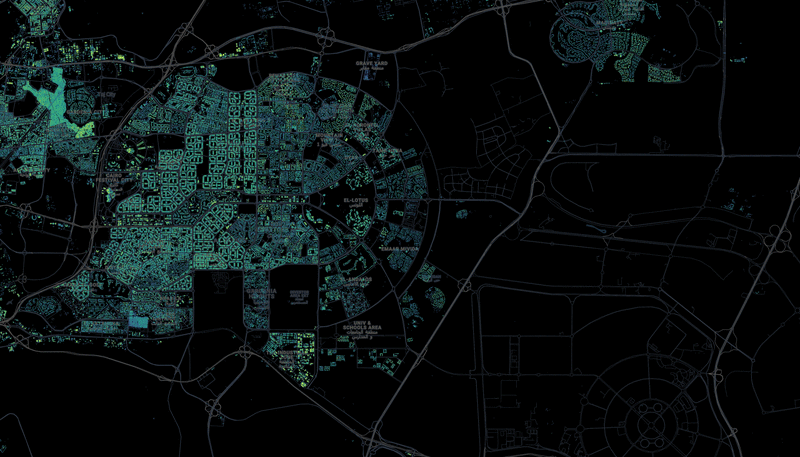
This is an optional setting above a sound player set to H4
Lorem ipsum dolor sit amet, consectetur adipiscing elit, sed do eiusmod temporincididunt ut labore et dolore magna aliqua. Ut enimad minim veniam, quis nostrud exercitation ullamco laboris nisi ut aliquip ex ea commodo consequat.
Optional caption test tests test test x2 x2 x2, set to center alignment
This is an optional header with no description.
Large language models (LLMs) are increasingly being example footnote
Retrieval augmented generation (RAG) recently emerged as a promising solution to mitigate these issues. RAG leverages an external knowledge base to retrieve documents with related information, and incorporates this information into its generated content. By retrieving timely and accurate information, RAG effectively reduces factual errors in knowledge-intensive tasks. While RAG improves the accuracy of LLM responses, longer documents require more complex reasoning and can significantly delay response times. Recent studies have explored paths to extend the context length limit of LLMs, yet achieving well-grounded reasoning over such extended contexts remains an open challenge. Consequently, striking a balance between efficiency and effectiveness in RAG has become a central research focus.
In “Speculative RAG: Enhancing Retrieval Augmented Generation through Drafting”, we propose a novel framework that offloads computational burden to a smaller specialist RAG drafter (a specialized LM fine-tuned for RAG), which serves as an efficient and robust RAG module for the existing generalist LM.
Speculative RAG follows the drafting approach described in speculative decoding, a method that accelerates auto-regressive LM inference by using a smaller model to concurrently and rapidly generate multiple subsequent tokens (e.g., words or word segments) verified in parallel with a base model, to improve the effectiveness and efficiency of RAG systems. We demonstrate that Speculative RAG yields significant improvements and achieves state-of-the-art performance in both accuracy and latency on the TriviaQA, MuSiQue, PubHealth, and ARC-Challenge benchmarks.
Speculative RAG
Speculative RAG consists of two components: (1) a specialist RAG drafter, and (2) a generalist RAG verifier. First, the base model’s knowledge retriever retrieves related documents from the knowledge base. Then, Speculative RAG offloads computational burden to the specialist RAG drafter, a small LM specialized in answering questions using retrieved documents and not expected to cope with general problems. This smaller module excels at reasoning over retrieved documents and can rapidly produce responses with their corresponding rationale. It serves as an efficient and robust RAG module for the generalist LM. The specialist drafter enables the generalist verifier to bypass the detailed review of potentially repetitive documents, focusing instead on validating the drafts and selecting the most accurate answer.
For example, when answering, “Which actress or singer starred as Doralee Rhodes in the 1980 film, Nine to Five?”, we retrieve a number of documents from the knowledge base with a retriever. We feed subsets of retrieved documents into the RAG drafter and generate multiple answer drafts with corresponding rationale in parallel. This guarantees a high processing speed of the large number of documents.
We determine that some retrieved documents are not relevant due to the limited capability of the knowledge retriever. In this example, the retrieved documents contain information about both the Nine to Five movie (1980) and the Nine to Five musical (2010). To determine the most accurate draft, the generalist RAG verifier, a general LLM, calculates the conditional generation probability of the answer drafts with rationales and outputs a confidence score. Since answer drafts based on the Nine to Five musical would be inaccurate, the generalist RAG verifier assigns those drafts lower scores and filters them out. Finally, the generalist verifier selects the answer draft with the highest confidence score, which is based on the Nine to Five movie, as the final answer.
AutoBNN is based on a line of research that over the past decade has yielded improved predictive accuracy by modeling time series using GPs with learned kernel structures. The kernel function of a GP encodes assumptions about the function being modeled, such as the presence of trends, periodicity or noise. With learned GP kernels, the kernel function is defined compositionally: it is either a base kernel (such as Linear
, Quadratic
, Periodic
, Matérn
or ExponentiatedQuadratic
) or a composite that combines two or more kernel functions using operators such as Addition
, Multiplication
, or ChangePoint
. This compositional kernel structure serves two related purposes. First, it is simple enough that a user who is an expert about their data, but not necessarily about GPs, can construct a reasonable prior for their time series. Second, techniques like Sequential Monte Carlo can be used for discrete searches over small structures and can output interpretable results.
AutoBNN improves upon these ideas, replacing the GP with Bayesian neural networks (BNNs) while retaining the compositional kernel structure. A BNN is a neural network with a probability distribution over weights rather than a fixed set of weights. This induces a distribution over outputs, capturing uncertainty in the predictions. BNNs bring the following advantages over GPs: First, training large GPs is computationally expensive, and traditional training algorithms scale as the cube of the number of data points in the time series. In contrast, for a fixed width, training a BNN will often be approximately linear in the number of data points. Second, BNNs lend themselves better to GPU and TPU hardware acceleration than GP training operations. Third, compositional BNNs can be easily combined with traditional deep BNNs, which have the ability to do feature discovery. One could imagine "hybrid" architectures, in which users specify a top-level structure of Add
(Linear
, Periodic
, Deep
), and the deep BNN is left to learn the contributions from potentially high-dimensional covariate information.
How might one translate a GP with compositional kernels into a BNN then? A single layer neural network will typically converge to a GP as the number of neurons (or "width") goes to infinity. More recently, researchers have discovered a correspondence in the other direction — many popular GP kernels (such as Matern
, ExponentiatedQuadratic
, Polynomial
or Periodic
) can be obtained as infinite-width BNNs with appropriately chosen activation functions and weight distributions. Furthermore, these BNNs remain close to the corresponding GP even when the width is very much less than infinite. For example, the figures below show the difference in the covariance between pairs of observations, and regression results of the true GPs and their corresponding width-10 neural network versions.
AutoBNN improves upon these ideas, replacing the GP with Bayesian neural networks (BNNs) while retaining the compositional kernel structure. A BNN is a neural network with a probability distribution over weights rather than a fixed set of weights. This induces a distribution over outputs, capturing uncertainty in the predictions. BNNs bring the following advantages over GPs: First, training large GPs is computationally expensive, and traditional training algorithms scale as the cube of the number of data points in the time series. In contrast, for a fixed width, training a BNN will often be approximately linear in the number of data points. Second, BNNs lend themselves better to GPU and TPU hardware acceleration than GP training operations. Third, compositional BNNs can be easily combined with traditional deep BNNs, which have the ability to do feature discovery. One could imagine "hybrid" architectures, in which users specify a top-level structure of Add
(Linear
, Periodic
, Deep
), and the deep BNN is left to learn the contributions from potentially high-dimensional covariate information.
test caption
test caption
test caption