Heading test
August 9, 2024
Yun Zhu and Lijuan Liu, Software Engineers, Google Research
Quick links
asdfasdfasfd
hkjhkjhkjh
This video has Autoplay checked
This video has play inline unchecked. Help text says "Autoplay without controls and muted. Does not apply to embedded videos."
This video has Autoplay unchecked
ttest
test
Recent advancements in Large Language Models (LLMs) have laid the foundation for the development of highly capable multimodal LLMs (MLLMs) like Gemini. MLLMs can process additional modalities, such as images or videos, while retaining language understanding and generation capabilities. Despite the impressive performance of MLLMs across a variety of tasks, the issue of object hallucination presents a significant challenge to their widespread adoption. Object hallucination refers to generated language that includes descriptions of objects or their attributes that are not present in or cannot be verified by the given input.
Data augmented contrastive tuning
Our method consists of two key steps: (1) generative data augmentation and (2) contrastive tuning. For a given pair of vision-language instructions and a corresponding correct answer, generative data augmentation is applied to obtain hallucinated responses. We selectively alter ground-truth objects and object-related attributes from the correct answer to introduce hallucinated concepts that are not present in the input images.
A contrastive loss is then calculated between a pair of factual and hallucinated tokens. Our objective is to minimize the likelihood of generating hallucinated tokens and correspondingly, maximize the likelihood of generating factual tokens. We train the MLLM with a KL-divergence regularizer that ensures that the MLLM retains its original performance in general vision-language tasks by preventing it from diverging from the base model. We refer to MLLMs trained with the contrastive tuning framework as Hallucination Attenuated Language and Vision Assistants (HALVA).
Data augmented contrastive tuning
Our method consists of two key steps: (1) generative data augmentation and (2) contrastive tuning. For a given pair of vision-language instructions and a corresponding correct answer, generative data augmentation is applied to obtain hallucinated responses. We selectively alter ground-truth objects and object-related attributes from the correct answer to introduce hallucinated concepts that are not present in the input images.
A contrastive loss is then calculated between a pair of factual and hallucinated tokens. Our objective is to minimize the likelihood of generating hallucinated tokens and correspondingly, maximize the likelihood of generating factual tokens. We train the MLLM with a KL-divergence regularizer that ensures that the MLLM retains its original performance in general vision-language tasks by preventing it from diverging from the base model. We refer to MLLMs trained with the contrastive tuning framework as Hallucination Attenuated Language and Vision Assistants (HALVA).
Data augmented contrastive tuning
Our method consists of two key steps: (1) generative data augmentation and (2) contrastive tuning. For a given pair of vision-language instructions and a corresponding correct answer, generative data augmentation is applied to obtain hallucinated responses. We selectively alter ground-truth objects and object-related attributes from the correct answer to introduce hallucinated concepts that are not present in the input images.
A contrastive loss is then calculated between a pair of factual and hallucinated tokens. Our objective is to minimize the likelihood of generating hallucinated tokens and correspondingly, maximize the likelihood of generating factual tokens. We train the MLLM with a KL-divergence regularizer that ensures that the MLLM retains its original performance in general vision-language tasks by preventing it from diverging from the base model. We refer to MLLMs trained with the contrastive tuning framework as Hallucination Attenuated Language and Vision Assistants (HALVA).
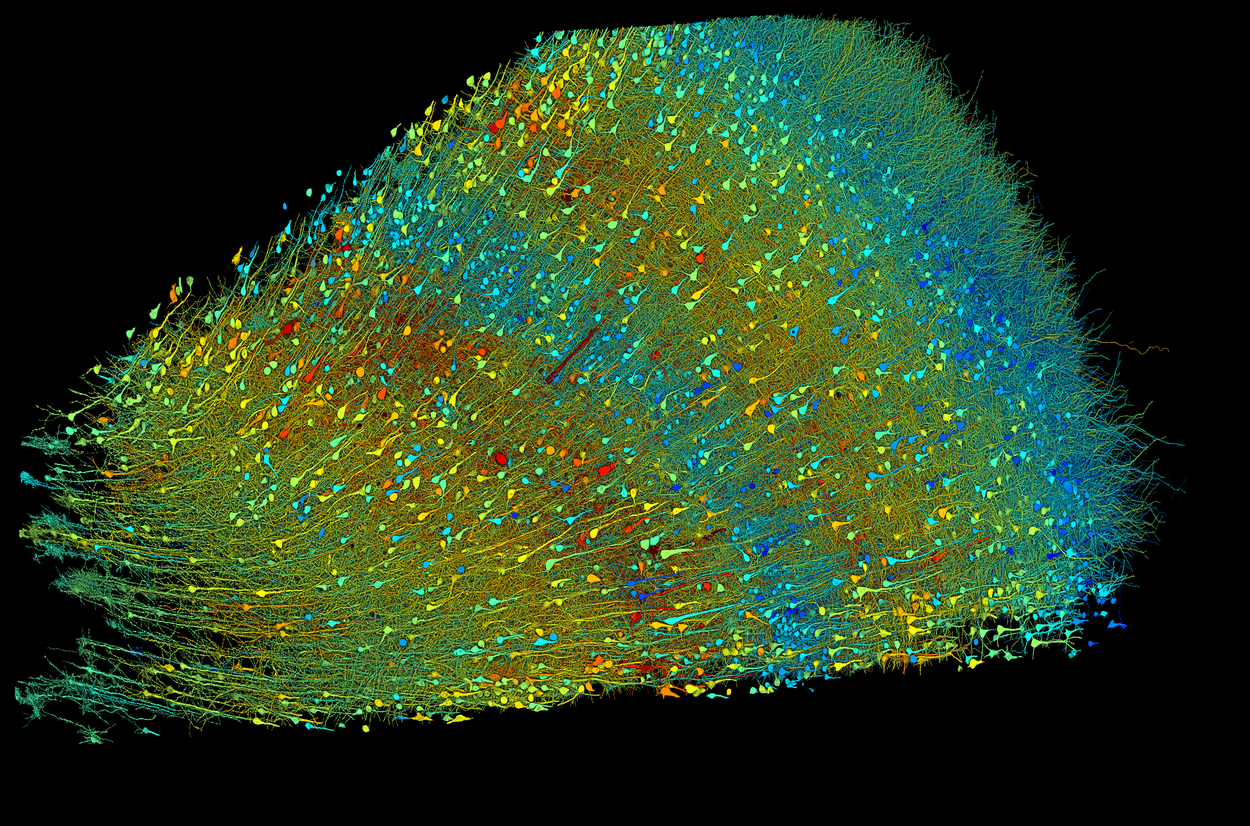
Vertical padding: top only