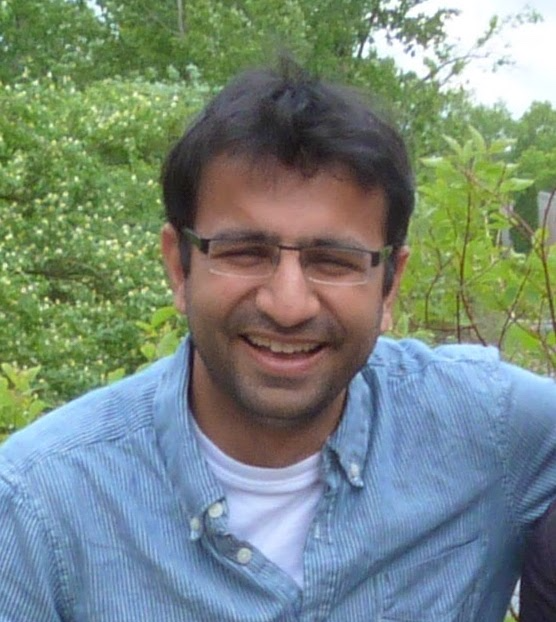
Varun Gulshan
I've been with Google since 2013, working in the areas of Machine Learning, Computer Vision and Health Research. I lead the Earth Observation Sciences team, which is part of the Climate and Energy efforts within Applied Sciences. Our team's mission is to use Machine Learning and Earth Observation imagery to solve Geoscience research questions, motivated by applications in climate mitigation and adaptation. Prior to this role, I was the engineering co-founder of the medical imaging team within Google research, where we developed and deployed models for detecting diabetic retinopathy from retina images. Prior to Google I was part of the 6 people team at Flutter, building systems for real time gesture recognition. I completed my PhD from the Visual Geometry Group in Oxford, UK and Bachelors in Computer Science from the Indian Institute of Technology (IIT), Delhi. Link to my personal website.
Research Areas
Authored Publications
Sort By
Satellite Sunroof: High-res Digital Surface Models and Roof Segmentation for Global Solar Mapping
Alex Wilson
Betty Peng
Carl Elkin
Umangi Jain
2024
Preview abstract
The transition to renewable energy sources such as solar is crucial for mitigating climate change. Google Maps Platform Solar API aims to accelerate this transition by providing accurate estimates of solar potential for buildings covered by aerial imagery. However, its impact is limited by geographical coverage and data availability. This paper presents an approach to expand the project's capabilities using satellite imagery, enabling global-scale solar potential assessment. We address challenges specific to satellite imagery, such as lower resolution and oblique views, by developing deep learning models for Digital Surface Model (DSM) estimation and roof plane segmentation. The models are trained and evaluated on datasets comprising of spatially aligned satellite and aerial imagery. Our results demonstrate the effectiveness of our approach in accurately predicting DSMs and roof segments from satellite imagery, paving the way for a significant expansion of the Solar API and impact in promoting solar adoption.
View details
Preview abstract
We develop a deep learning based convolutional-regression model that estimates the volumetric soil moisture content in the top ~5 cm. Input predictors include Sentinel-1 (active radar), Sentinel-2 (optical imagery), and SMAP (passive radar) as well as geophysical variables from SoilGrids and modelled soil moisture fields from GLDAS. The model was trained and evaluated on data from ~1300 in-situ sensors globally over the period 2015 - 2021 and obtained an average per-sensor correlation of 0.72 and ubRMSE of 0.0546. These results are benchmarked against 13 other soil moisture estimates at different locations, and an ablation study was used to identify important predictors.
View details
Cross-modal distillation for flood extent mapping
Shubhika Garg
Ben Feinstein
Shahar Timnat
Gideon Dror
Adi Gerzi Rosenthal
Environmental Data Science (2023)
Preview abstract
The increasing intensity and frequency of floods is one of the many consequences of our changing climate. In this work, we explore ML techniques that improve the flood detection module of an operational early flood warning system. Our method exploits an unlabelled dataset of paired multi-spectral and Synthetic Aperture Radar (SAR) imagery to reduce the labeling requirements of a purely supervised learning method. Prior works have used unlabelled data by creating weak labels out of them. However, from our experiments we noticed that such a model still ends up learning the label mistakes in those weak labels. Motivated by knowledge distillation and semi supervised learning, we explore the use of a teacher to train a student with the help of a small hand labelled dataset and a large unlabelled dataset. Unlike the conventional self distillation setup, we propose a cross modal distillation framework that transfers supervision from a teacher trained on richer modality (multi-spectral images) to a student model trained on SAR imagery. The trained models are then tested on the Sen1Floods11 dataset. Our model outperforms the Sen1Floods11 baseline model trained on the weak labeled SAR imagery by an absolute margin of 6.53% Intersection-over-Union (IoU) on the test split.
View details
Cross Modal Distillation for Flood Extent Mapping
Shubhika Garg
Ben Feinstein
Shahar Timnat
Gideon Dror
Adi Gerzi Rosenthal
Tackling Climate Change with Machine Learning, NeurIPS 2022 Workshop
Preview abstract
The increasing intensity and frequency of floods is one of the many consequences of our changing climate. In this work, we explore ML techniques that improve the flood detection module of an operational early flood warning system. Our method exploits an unlabelled dataset of paired multi-spectral and Synthetic Aperture Radar (SAR) imagery to reduce the labeling requirements of a purely supervised learning method. Past attempts have used such unlabelled data by creating weak labels out of them, but end up learning the label mistakes in those weak labels. Motivated by knowledge distillation and semi supervised learning, we explore the use of a teacher to train a student with the help of a small hand labeled dataset and a large unlabelled dataset. Unlike the conventional self distillation setup, we propose a cross modal distillation framework that transfers supervision from a teacher trained on richer modality (multi-spectral images) to a student model trained on SAR imagery. The trained models are then tested on the Sen1Floods11 dataset. Our model outperforms the Sen1Floods11 SAR baselines by an absolute margin of 4.15% pixel wise Intersection-over-Union (IoU) on the test split.
View details
Multimodal contrastive learning for remote sensing tasks
Umangi Jain
Alex Wilson
Self-Supervised Learning - Theory and Practice, NeurIPS 2022 Workshop
Preview abstract
Self-supervised methods have shown tremendous success in the field of computer vision, including subfields like remote sensing and medical imaging. Most popular contrastive-loss based methods like SimCLR, MoCo, MoCo-v2 use multiple views of the same image by applying contrived augmentations on the image to create positive pairs and contrast them with negative examples. Although these techniques work well, most of these techniques have been tuned on ImageNet (and similar computer vision datasets). While there have been some attempts to capture a richer set of deformations in the positive samples, in this work, we explore a promising alternative to generating positive examples for remote sensing data within the contrastive learning framework. Images captured from different sensors at the same location and nearby timestamps can be thought of as strongly augmented instances of the same scene, thus removing the need to explore and tune a set of hand crafted strong augmentations. In this paper, we propose a simple dual-encoder framework, which is pre-trained on a large unlabeled dataset (~1M) of Sentinel-1 and Sentinel-2 image pairs. We test the embeddings on two remote sensing downstream tasks: flood segmentation and land cover mapping, and empirically show that embeddings learnt from this technique outperforms the conventional technique of collecting positive examples via aggressive data augmentations.
View details
Preview abstract
We perform a rigorous evaluation of recent self and semi-supervised ML techniques that leverage unlabeled data for improving downstream task performance, on three remote sensing tasks of riverbed segmentation, land cover mapping and flood mapping. These methods are especially valuable for remote sensing tasks since there is easy access to unlabeled imagery and getting ground truth labels can often be expensive. We quantify performance improvements one can expect on these remote sensing segmentation tasks when unlabeled imagery (outside of the labeled dataset) is made available for training. We also design experiments to test the effectiveness of these techniques when the test set has a domain shift relative to the training and validation sets.
View details
Inundation Modeling in Data Scarce Regions
Zvika Ben-Haim
Vova Anisimov
Yusef Shafi
Sella Nevo
Artificial Intelligence for Humanitarian Assistance and Disaster Response Workshop (2019)
Preview abstract
Flood forecasts are crucial for effective individual and governmental protective action. The vast majority of flood-related casualties occur in developing countries, where providing spatially accurate forecasts is a challenge due to scarcity of data and lack of funding. This paper describes an operational system providing flood extent forecast maps covering several flood-prone regions in India, with the goal of being sufficiently scalable and cost-efficient to facilitate the establishment of effective flood forecasting systems globally.
View details
Performance of a Deep-Learning Algorithm vs Manual Grading for Detecting Diabetic Retinopathy in India
Renu P. Rajan
Derek Wu
Peter Wubbels
Tyler Rhodes
Kira Whitehouse
Ramasamy Kim
Rajiv Raman
Lily Peng
JAMA Ophthalmology (2019)
Preview abstract
Importance More than 60 million people in India have diabetes and are at risk for diabetic retinopathy (DR), a vision-threatening disease. Automated interpretation of retinal fundus photographs can help support and scale a robust screening program to detect DR.
Objective To prospectively validate the performance of an automated DR system across 2 sites in India.
Design, Setting, and Participants This prospective observational study was conducted at 2 eye care centers in India (Aravind Eye Hospital and Sankara Nethralaya) and included 3049 patients with diabetes. Data collection and patient enrollment took place between April 2016 and July 2016 at Aravind and May 2016 and April 2017 at Sankara Nethralaya. The model was trained and fixed in March 2016.
Interventions Automated DR grading system compared with manual grading by 1 trained grader and 1 retina specialist from each site. Adjudication by a panel of 3 retinal specialists served as the reference standard in the cases of disagreement.
Main Outcomes and Measures Sensitivity and specificity for moderate or worse DR or referable diabetic macula edema.
Results Of 3049 patients, 1091 (35.8%) were women and the mean (SD) age for patients at Aravind and Sankara Nethralaya was 56.6 (9.0) years and 56.0 (10.0) years, respectively. For moderate or worse DR, the sensitivity and specificity for manual grading by individual nonadjudicator graders ranged from 73.4% to 89.8% and from 83.5% to 98.7%, respectively. The automated DR system’s performance was equal to or exceeded manual grading, with an 88.9% sensitivity (95% CI, 85.8-91.5), 92.2% specificity (95% CI, 90.3-93.8), and an area under the curve of 0.963 on the data set from Aravind Eye Hospital and 92.1% sensitivity (95% CI, 90.1-93.8), 95.2% specificity (95% CI, 94.2-96.1), and an area under the curve of 0.980 on the data set from Sankara Nethralaya.
Conclusions and Relevance This study shows that the automated DR system generalizes to this population of Indian patients in a prospective setting and demonstrates the feasibility of using an automated DR grading system to expand screening programs.
View details
Grader variability and the importance of reference standards for evaluating machine learning models for diabetic retinopathy
Ehsan Rahimy
Peter Karth
Lily Peng
Ophthalmology (2018)
Preview abstract
Purpose
Use adjudication to quantify errors in diabetic retinopathy (DR) grading based on individual graders and majority decision, and to train an improved automated algorithm for DR grading.
Design
Retrospective analysis.
Participants
Retinal fundus images from DR screening programs.
Methods
Images were each graded by the algorithm, U.S. board-certified ophthalmologists, and retinal specialists. The adjudicated consensus of the retinal specialists served as the reference standard.
Main Outcome Measures
For agreement between different graders as well as between the graders and the algorithm, we measured the (quadratic-weighted) kappa score. To compare the performance of different forms of manual grading and the algorithm for various DR severity cutoffs (e.g., mild or worse DR, moderate or worse DR), we measured area under the curve (AUC), sensitivity, and specificity.
Results
Of the 193 discrepancies between adjudication by retinal specialists and majority decision of ophthalmologists, the most common were missing microaneurysm (MAs) (36%), artifacts (20%), and misclassified hemorrhages (16%). Relative to the reference standard, the kappa for individual retinal specialists, ophthalmologists, and algorithm ranged from 0.82 to 0.91, 0.80 to 0.84, and 0.84, respectively. For moderate or worse DR, the majority decision of ophthalmologists had a sensitivity of 0.838 and specificity of 0.981. The algorithm had a sensitivity of 0.971, specificity of 0.923, and AUC of 0.986. For mild or worse DR, the algorithm had a sensitivity of 0.970, specificity of 0.917, and AUC of 0.986. By using a small number of adjudicated consensus grades as a tuning dataset and higher-resolution images as input, the algorithm improved in AUC from 0.934 to 0.986 for moderate or worse DR.
Conclusions
Adjudication reduces the errors in DR grading. A small set of adjudicated DR grades allows substantial improvements in algorithm performance. The resulting algorithm's performance was on par with that of individual U.S. Board-Certified ophthalmologists and retinal specialists.
View details
Preview abstract
Data are often labelled by many different experts with each expert only labeling a small fraction of the data and each data point being labelled by several experts. This reduces the workload on individual experts and also gives a better estimate of the unobserved ground truth. When experts disagree, the standard approaches are to treat the majority opinion as the correct label or to model the correct label as a distribution. These approaches, however, do not make any use of potentially valuable information about which expert produced which label. To make use of this extra information, we propose modeling the experts individually and then learning mixing proportions for combining them in sample-specific ways. This allows us to give more weight to more reliable experts and makes it possible to take advantage of the unique strengths of individual experts at classifying certain types of data. Here we show that our approach leads to improved computer-aided diagnosis of diabetic retinopathy, where the experts are human doctors and the data are retinal images. We compare our method against those of Welinder and Perona, and Mnih and Hinton. Our work offers an innovative approach for dealing with the myriad real-world settings that lack ground truth labels.
View details