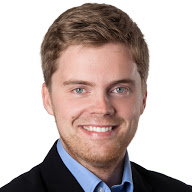
Tomas Pfister
Tomas Pfister is the Head of Cloud AI Research. He came to Google from Apple where he cofounded Apple's central AI research group and published Apple’s first research paper that won the Best Paper Award at CVPR’17. Tomas’ key scientific achievements have been proposing a method to improve the realism of synthetic images; developing the first automated method to detect facial micro-expressions; and inventing a new way for neural networks to exploit spatiotemporal structure. He is currently exploring learning from small amount of labeled data (using techniques such as generative models, few-shot learning, transfer learning) and explainability/interpretability of deep learning models, and is particularly excited about the potential of AI in healthcare & education. His research has laid the foundation for several applications such as Face ID in iPhone X, autonomous driving, human pose estimation, detecting facial micro-expressions & translating sign language. Tomas did his PhD in deep learning with Prof Andrew Zisserman at Oxford University and bachelor’s degree in computer science at Cambridge University. He is the recipient of the Forbes 30 Under 30 award, and has received over 40 research awards, including 3 best paper awards, with numerous publications in top AI research venues. His work has been frequently featured in mainstream media, including Forbes, BusinessInsider & Wired.
Authored Publications
Sort By
Found in the middle: Calibrating Positional Attention Bias Improves Long Context Utilization
Cheng-Yu Hsieh
Yung-Sung Chuang
Chun-Liang Li
Abhishek Kumar
James Glass
Alexander Ratner
Ranjay Krishna
2024
Preview abstract
Large language models (LLMs), even when specifically trained to process long input contexts, struggle to capture relevant information located in the middle of their input. This phenomenon has been known as the lost-in-the-middle problem. In this work, we make three contributions. First, we set out to understand the factors that cause this phenomenon. In doing so, we establish a connection between lost-in-the-middle to LLMs' intrinsic attention bias: LLMs exhibit a U-shaped attention bias where the tokens at the beginning and at the end of its input receive higher attention, regardless of their relevance. Second, we mitigate this positional bias through a calibration mechanism, found-in-the-middle, that allows the model to attend to contexts faithfully according to their relevance, even though when they are in the middle. Third, we show found-in-the-middle not only achieves better performance in locating relevant information within a long context, but also eventually leads to improved retrieval-augmented generation (RAG) performance across various tasks, outperforming existing methods by up to 15 percentage points. These findings open up future directions in understanding LLM attention bias and its potential consequences.
View details
ASPEST: Bridging the Gap Between Active Learning and Selective Prediction
Somesh Jha
Transactions on Machine Learning Research (TMLR) (2024)
Preview abstract
Selective prediction aims to learn a reliable model that abstains from making predictions when uncertain. These predictions can then be deferred to humans for further evaluation. As an everlasting challenge for machine learning, in many real-world scenarios, the distribution of test data is different from the training data. This results in more inaccurate predictions, and often increased dependence on humans, which can be difficult and expensive. Active learning aims to lower the overall labeling effort, and hence human dependence, by querying the most informative examples. Selective prediction and active learning have been approached from different angles, with the connection between them missing. In this work, we introduce a new learning paradigm, active selective prediction, which aims to query more informative samples from the shifted target domain while increasing accuracy and coverage. For this new paradigm, we propose a simple yet effective approach, ASPEST, that utilizes ensembles of model snapshots with self-training with their aggregated outputs as pseudo labels. Extensive experiments on numerous image, text and structured datasets, which suffer from domain shifts, demonstrate that ASPEST can significantly outperform prior work on selective prediction and active learning (e.g. on the MNIST→SVHN benchmark with the labeling budget of 100, ASPEST improves the AUACC metric from 79.36% to 88.84%) and achieves more optimal utilization of humans in the loop.
View details
Model Swarms: Collaborative Search of Adapted LLM Experts via Swarm Intelligence
Shangbin Feng
Yike Wang
Nathalie Rauschmayr
Yejin Choi
Yulia Tsvetkov
2024
Preview abstract
We propose Model Swarms, a collaborative search algorithm to adapt LLM experts via swarm intelligence. Specifically, Model Swarms starts with a pool of LLM experts and a utility function. Guided by the best-found checkpoints across models, diverse LLM experts collaboratively move in the weight space and search for adapted models that optimize the utility function. Compared to existing model composition approaches, Model Swarms offers modularity, works in low-data regimes, and doesn't need assumptions about existing experts and how they should be composed. Extensive experiments demonstrate that Model Swarms could flexibly adapt LLM experts to a single dataset, multi-dataset domains, reward models, as well as diverse human preferences. Further analysis reveals that LLM experts discover previously unseen capabilities in the search process and that Model Swarms enable the weak-to-strong transition of experts through the collaborative search process.
View details
Preview abstract
Large language models (LLMs) have achieved remarkable advancements in natural language understanding, generation, and manipulation of text-based data. However, one major issue towards their widespread deployment in the real world is that they can generate "hallucinated" answers that are not factual. Towards this end, this paper focuses on improving grounding from a holistic perspective with a novel framework, AGREE. We start with the design of a test time adaptation capability that takes into account the support information generated in self-grounded responses. To effectively enable this capability, we propose that the model tuning needs to be redesigned with a novel tuning objective mimicking the test time adaptation setup for grounding. This tuning on top of the pre-trained LLMs requires small amount of data that need to be constructed in a particular way to learn the grounding information, for which we introduce a data construction method. Our results show that AGREE pushes the state-of-the-art in grounding, demonstrated across many datasets.
View details
Preview abstract
With development of Large Language Models (LLMs), collaboration between LLMs to solve complex tasks has attracted more and more attention. An important challenging task is reasoning from long text that cannot be input into LLMs. Thus far, limited research has explored how to solve long context tasks via pure multi-agent collaboration.
In this paper, we propose Chain-of-Agents (CoA), a novel framework that leverages the multi-agent collaboration via natural language to solve complex tasks. In CoA, the long text is split into chunks to be processed by agents repeatedly with appending the information from preceding agents. A manager model is finally employed to obtain the final answer utilizing the output of the last agent.
On wide range of datasets for long context question answering, summarization, and code completion and with many LLMs (including PaLM 2, Claude, and Gemini), we show that CoA framework outperforms strong baselines, including the commonly-used retrieval augmented generation (RAG) systems, by a large margin. For instance, text-bison obtains 13.30\% performance gain on NarrativeQA, and 10.22\% on MuSiQue dataset.
View details
CodecLM: Aligning Language Models with Tailored Synthetic Data
Chun-Liang Li
Jin Miao
NAACL 2024
Preview abstract
Instruction tuning has emerged as the key in aligning large language models (LLMs) with specific task instructions, thereby mitigating the discrepancy between the next-token prediction objective and users' actual goals. To reduce the labor and time cost to collect or annotate data by humans, researchers start to explore the use of LLMs to generate instruction-aligned synthetic data. Recent works focus on generating diverse instructions and applying LLM to increase instruction complexity, often neglecting downstream use cases. It remains unclear how to tailor high-quality data to elicit better instruction-following abilities in different target instruction distributions and LLMs. To this end, we introduce CodecLM, a general framework for adaptively generating high-quality synthetic data for LLM alignment with different downstream instruction distributions and LLMs. Drawing on the Encode-Decode principles, we use LLMs as codecs to guide the data generation process. We first encode seed instructions into metadata, which are concise keywords generated on-the-fly to capture the target instruction distribution, and then decode metadata to create tailored instructions. We also introduce Self-Rubrics and Contrastive Filtering during decoding to tailor data-efficient samples. Extensive experiments on four open-domain instruction following benchmarks validate the effectiveness of CodecLM over the current state-of-the-arts.
View details
SQL-PaLM: Improved Large Language Model Adaptation for Text-to-SQL
Satya Gundabathula
Hanjun Dai
TMLR (2024)
Preview abstract
Text-to-SQL, the process of translating natural language into Structured Query Language
(SQL), represents a transformative application of large language models (LLMs), potentially
revolutionizing how humans interact with data. This paper introduces the SQL-PaLM
framework, a comprehensive solution for understanding and enhancing Text-to-SQL using
LLMs, using in the learning regimes of few-shot prompting and instruction fine-tuning. With
few-shot prompting, we explore the effectiveness of consistency decoding with execution-based error filtering. With instruction fine-tuning, we delve deep in understanding the critical
paradigms that influence the performance of tuned LLMs. In particular, we investigate
how performance can be improved through expanded training data coverage and diversity,
synthetic data augmentation, and integrating query-specific database content. We propose
a test-time selection method to further refine accuracy by integrating SQL outputs from
multiple paradigms with execution feedback as guidance. Additionally, we tackle the
practical challenge of navigating intricate databases with a significant number of tables and
columns, proposing efficient techniques for accurately selecting relevant database elements to
enhance Text-to-SQL performance. Our holistic approach yields substantial advancements
in Text-to-SQL, as demonstrated on two key public benchmarks, Spider and BIRD. Through
comprehensive ablations and error analyses, we shed light on the strengths and weaknesses
of our framework, offering valuable insights into Text-to-SQL’s future work.
View details
Chain-of-Table: Evolves Tables in the LLM Reasoning Chain for Table Understanding
Zilong Wang
Hao Zhang
Chun-Liang Li
Jingbo Shang
ICLR (2024)
Preview abstract
Table-based reasoning with large language models (LLMs) is a promising direction to tackle many table understanding tasks, such as table-based question answering and fact verification. Compared with generic reasoning, table-based reasoning requires the extraction of underlying semantics from both free-form questions and semi-structured tabular data. Chain-of-Thought and its similar approaches incorporate the reasoning chain in the form of textual context, but it is still an open question how to effectively leverage tabular data in the reasoning chain. We propose the Chain-of-Table framework, where tabular data is explicitly used in the reasoning chain as a proxy for intermediate thoughts. Specifically, we guide LLMs using in-context learning to iteratively generate operations and update the table to represent a tabular reasoning chain. LLMs can therefore dynamically plan the next operation based on the results of the previous ones. This continuous evolution of the table forms a chain, showing the reasoning process for a given tabular problem. The chain carries structured information of the intermediate results, enabling more accurate and reliable predictions. Chain-of-Table achieves new state-of-the-art performance on WikiTQ, FeTaQA, and TabFact benchmarks across multiple LLM choices.
View details
Preview abstract
Grounded generation aims to equip language models (LMs) with the ability to produce more credible and accountable responses by accurately citing verifiable sources. However, existing methods, by either feeding LMs with raw or preprocessed materials, remain prone to errors. To address this, we introduce CaLM, a novel verification framework. CaLM leverages the insight that a robust grounded response should be consistent with information derived solely from its cited sources. Our framework empowers smaller LMs, which rely less on parametric memory and excel at processing relevant information given a query, to validate the output of larger LMs. Larger LM responses that closely align with the smaller LMs' output, which relies exclusively on cited documents, are verified. Responses showing discrepancies are iteratively refined through a feedback loop. Experiments on three open-domain question-answering datasets demonstrate significant performance gains of 1.5% to 7% absolute average without any required model fine-tuning.
View details
Preview abstract
While remarkable progress has been made in imbalanced supervised learning, less attention has been given to the setting of imbalanced semi-supervised learning (SSL) where not only are few labeled data provided, but the underlying data distribution can be severely imbalanced. Recent work requires both complicated sampling strategies of pseudo-labeled unlabeled data and distribution alignment of the pseudo-label distribution to accommodate this imbalance. We present a novel approach that relies only on a form of a distribution alignment but no sampling strategy where rather than aligning the pseudo-labels during inference, we move the distribution alignment component into the respective cross entropy loss computations for both the supervised and unsupervised losses. This alignment compensates for both imbalance in the data and the eventual distributional shift present during evaluation. Altogether, this provides a unified strategy that offers both significantly reduced training requirements and improved performance across both low and richly labeled regimes and over varying degrees of imbalance. In experiments, we validate the efficacy of our method on SSL variants of CIFAR10-LT, CIFAR100-LT, and ImageNet-127. On ImageNet-127, our method shows 1.6% accuracy improvement over CReST with an 80% training time reduction and is competitive with other SOTA methods.
View details