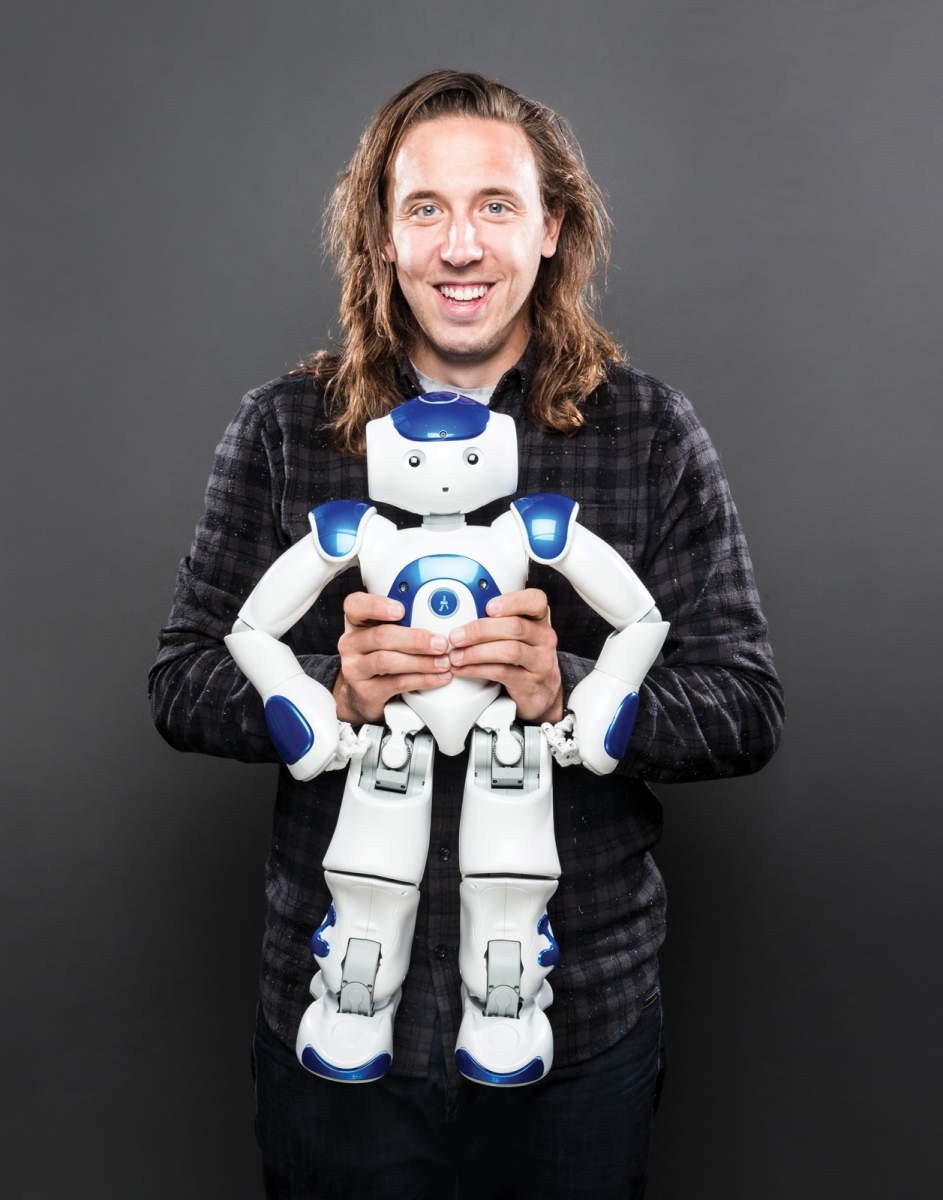
Kory Mathewson
Kory Mathewson is a Research Scientist with DeepMind and a Machine Learning Lab Scientist with the Creative Destruction Lab. He holds a Ph.D. in Computing Science from the University of Alberta with the Alberta Machine Intelligence Institute. His research interests include interactive machine learning, human-in-the-loop deep reinforcement learning, human-robot interfaces, prosthetic robotics, and conversational dialogue systems. Before his Ph.D., he completed his Bachelor’s degree in Electrical Engineering and his Master’s degree in Biomedical Engineering. Kory has interned at Twitter Cortex, at Google Brain Magenta, and at Apple Special Projects Group. Kory is an accomplished improvisational theatre performance artist with Rapid Fire Theatre. He fuses his interests by developing artificial intelligences to perform alongside.
Authored Publications
Sort By
Preview abstract
What are dimensions of human intent, and how do writing tools shape and augment these expressions? From papyrus to auto-complete, a major turning point was when Alan Turing famously asked, “Can Machines Think?” If so, should we offload aspects of our thinking to machines, and what impact do they have in enabling the intentions we have? This paper adapts the Authorial Leverage framework, from the Intelligent Narrative Technologies literature, for evaluating recent generative model advancements. With increased widespread access to Large Language Models (LLMs), the evolution of our evaluative frameworks follow suit. To do this, we discuss previous expert studies of deep generative models for fiction writers and playwrights, and propose two future directions, (1) author-focused and (2) audience-focused, for furthering our understanding of Authorial Leverage of LLMs, particularly in the domain of comedy writing.
View details
Story Centaur: Large Language Model Few Shot Learning as a Creative Writing Tool
Ben Pietrzak
Ben Swanson
Monica Dinculescu
EACL (European Association of Computational Linguistics) (2021)
Preview abstract
Few shot learning with large language models has the potential to give people without formal machine learning training the access to a wide range of text to text models. We consider how this applies to creative writers and present \textsc{Story Centaur}, a user interface for prototyping few shot models and a set of recombinable web components that deploy them. \textsc{Story Centaur}'s goal is to expose creative writers to few shot learning with a simple but powerful interface that lets these writers compose their own co-creation tools that further their own unique artistic directions. We build out several examples of this goal, and in the process probe the boundaries and issues surrounding generation with large language models.
View details
Preview abstract
A majority of games keep to discrete inputs and have not easily realized the expressivity of spoken language interfaces. Furthermore, natural language processing systems had limitations understanding language intent. For this paper, we define a type of language interface, Semantic Chat, and the challenges of achieving this functionality for interactive fiction and multiplayer games. In the past, games accepted text chat, through a keyboard, or voice chat, through a microphone; however, the inputs were often read verbatim and, at most, pattern matched to a desired intent. With recent advancements in deep learning, language models are able to more effectively derive the semantic meaning behind the textual input, and machine learning models have become increasingly better at transcribing voice. Even so, Semantic Chat is still rarely found in games. In practice, the application of these neural language models is an open problem, with non-trivial challenges in deployment. Using techniques like transfer learning, we discuss the obstacles in realizing believable voice avatars.
View details
Preview abstract
Our objective is to create an expressive language interface that allows human participants to have agency in narrative-driven virtual worlds. Text to Dialog (TTD) gives narrative designers an opportunity to paint audience participants into a story universe utilizing semantic similarity. To do this, we apply the Universal Sentence Encoder by using embedding vectors that specifically target transfer learning to story-dialog related NLP tasks. We conclude that building expressive tools like TTD could enable new artistic experiences through (1) Semantic Dialect Matching, where human-generated textual statements are semantically matched with a pre-scripted list of dialog (from an avatar's dialect, voice, or way of speaking), and (2) Semantic Dialog Selection, where natural language can maneuver decision points through semantic matching. We reference two case-studies to demonstrate each use-case.
View details
Shaping the Narrative Arc: Information-Theoretic Collaborative Dialogue
George Foster
Marc G. Bellemare
International Conference on Computational Creativity (2020)
Preview abstract
We consider the challenge of designing an artificial agent capable of interacting with humans in collaborative dialogue to produce creative, engaging narratives. Collaborative dialogue is distinct from chit-chat in that it is knowledge building, each utterance provides just enough information to add specificity and reduce ambiguity without limiting the conversation. We use concepts from information theory to define a narrative arc function which models dialogue progression. We demonstrate that this function can be used to modulate a generative conversation model and make it produce more interesting dialogues, compared to baseline outputs. We focus on two antithetical modes of modulation: reveal and conceal. Empirically, we show how the narrative arc function can model existing dialogues and shape conversation models towards either mode. We conclude with quantitative evidence suggesting that these modulated models provide interesting and engaging dialogue partners for improvisational theatre performers.
View details