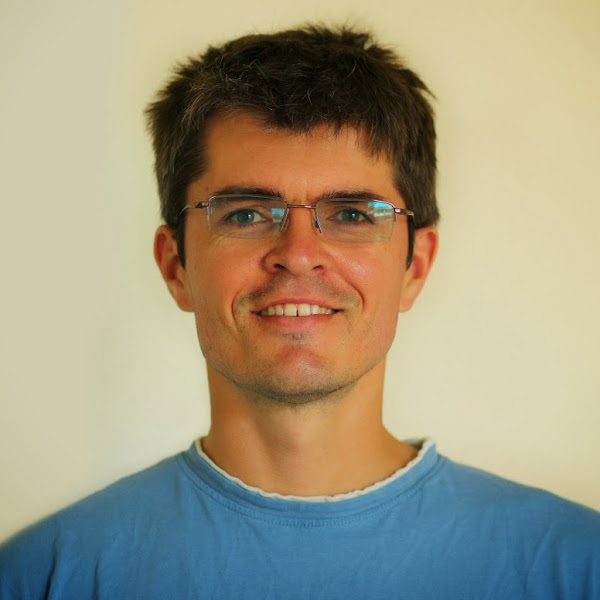
Martin Bokeloh
Martin Bokeloh is a software engineer at Google working on Cloud Robotics, focusing on 3D scene understanding and semantic segmentation. Before coming to Google Martin was a post-doctoral researcher at Stanford University and received his PhD from the Max Planck Institute in Saarbruecken.
Research Areas
Authored Publications
Sort By
3D-MPA: Multi Proposal Aggregation for 3D Semantic Instance Segmentation
Francis Engelmann
Bastian Leibe
Matthias Niessner
Proceedings of the IEEE Conference on Computer Vision and Pattern Recognition (CVPR) (2020)
Preview abstract
We present 3D-MPA, a method for instance segmentation on 3D point clouds.
Given an input point cloud, we propose an object-centric approach where each point votes for its object center.
We sample object proposals from the predicted object centers.
Then, we learn proposal features from grouped point features that voted for the same object center.
A graph convolutional network introduces inter-proposal relations, providing higher-level feature learning in addition to the lower-level point features.
Each proposal comprises a semantic label, a set of associated points over which we define a foreground-background mask, an objectness score and aggregation features.
Previous works usually perform non-maximum-suppression (NMS) over proposals to obtain the final object detections or semantic instances.
However, NMS can discard potentially correct predictions.
Instead, our approach keeps all proposals and groups them together based on the learned aggregation features.
We show that grouping proposals improves over NMS and outperforms previous state-of-the-art methods on the tasks of 3D object detection and semantic instance segmentation on the ScanNetV2 benchmark and the S3DIS dataset.
View details
Preview abstract
Augmented Reality aims at seamlessly blending virtual content into the real world. In this talk, I will showcase our recent work on 3D scene understanding. In particular, I will cover semantic segmentation and scan completion.
View details
ScanComplete: Large-Scale Scene Completion and Semantic Segmentation for 3D Scans
Angela Dai
Daniel Ritchie
Scott Reed
Matthias Nießner
Proc. Computer Vision and Pattern Recognition (CVPR), IEEE (2018)
Preview abstract
We introduce ScanComplete, a novel data-driven approach for taking an incomplete 3D scan of a scene as input and predicting a complete 3D model along with per-voxel semantic labels. The key contribution of our method is its ability to handle large scenes with varying spatial extent, managing the cubic growth in data size as scene size increases. To this end, we devise a fully-convolutional generative 3D CNN model whose filter kernels are invariant to the overall scene size. The model can be trained on scene subvolumes but deployed on arbitrarily large scenes at test time. In addition, we propose a coarse-to-fine inference strategy in order to produce high-resolution output while also leveraging large input context sizes. In an extensive series of experiments, we carefully evaluate different model design choices, considering both deterministic and probabilistic models for completion and semantic inference. Our results show that we outperform other methods not only in the size of the environments handled and processing efficiency, but also with regard to completion quality and semantic segmentation performance by a significant margin.
View details