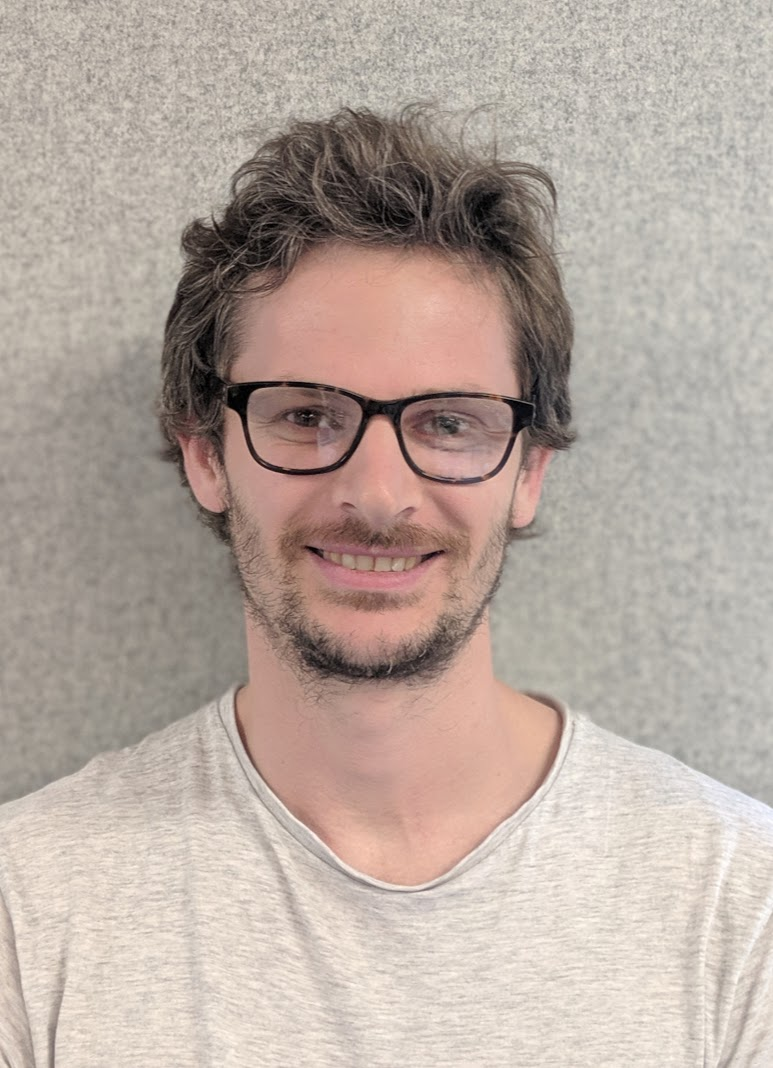
Sandy Ritchie
I work on internationalization for speech technology at Google. My research interests are in how we can scale language technology to a more diverse range of languages all around the world. Before joining Google, I received a Ph.D. and conducted postdoctoral research at SOAS, University of London.
Research Areas
Authored Publications
Sort By
LinguaMeta: Unified Metadata for Thousands of Languages
Uche Okonkwo
Emily Drummond
Proceedings of the 2024 Joint International Conference on Computational Linguistics, Language Resources and Evaluation (LREC-COLING 2024)
Preview abstract
We introduce LinguaMeta, a unified resource for language metadata for thousands of languages, including language codes, names, number of speakers, writing systems, countries, official status, coordinates, and language varieties. The resources are drawn from various existing repositories and supplemented with our own research. Each data point is tagged for its origin, allowing us to easily trace back to and improve existing resources with more up-to-date and complete metadata. The resource is intended for use by researchers and organizations who aim to extend technology to thousands of languages.
View details
Connecting Language Technologies with Rich, Diverse Data Sources Covering Thousands of Languages
Sebastian Ruder
Julia Kreutzer
Clara Rivera
Ishank Saxena
Proceedings of the 2024 Joint International Conference on Computational Linguistics, Language Resources and Evaluation (LREC-COLING 2024)
Preview abstract
Contrary to common belief, there are rich and diverse data sources available for many thousands of languages, which can be used to develop technologies for these languages. In this paper, we provide an overview of some of the major online data sources, the types of data that they provide access to, potential applications of this data, and the number of languages that they cover. Even this covers only a small fraction of the data that exists; for example, printed books are published in many languages but few online aggregators exist.
View details
Multimodal Modeling for Spoken Language Identification
Shikhar Bharadwaj
Sriram (Sri) Ganapathy
Sid Dalmia
Wei Han
Yu Zhang
Proceedings of 2024 IEEE International Conference on Acoustics, Speech and Signal Processing (ICASSP 2024) (2024)
Preview abstract
Spoken language identification refers to the task of automatically predicting the spoken language in a given utterance. Conventionally, it is modeled as a speech-based language identification task. Prior techniques have been constrained to a single modality; however in the case of video data there is a wealth of other metadata that may be beneficial for this task. In this work, we propose MuSeLI, a Multimodal Spoken Language Identification method, which delves into the use of various metadata sources to enhance language identification. Our study reveals that metadata such as video title, description and geographic location provide substantial information to identify the spoken language of the multimedia recording. We conduct experiments using two diverse public datasets of YouTube videos, and obtain state-of-the-art results on the language identification task. We additionally conduct an ablation study that describes the distinct contribution of each modality for language recognition.
View details
Chimane-Mosetén
Jeanette Sakel
Amazonian Languages: An International Handbook, De Gruyter Mouton (2023)
Preview abstract
Chimane-Mosetén (also known as Mosetenan; ISO 639–3: cas; Glottocode: mose1249) is a dialect continuum spoken by 13,500–16,000 people in the Amazonian region of northern Bolivia. It has not been convincingly shown to be related to any other language. Its status as an isolate makes it unique in many respects, not least in its combination of features typical of both Amazonian and Andean languages. Like its closer geographical neighbors in Amazonian Bolivia, including Movima, Tacana, Reyesano, and Cavineña, it exhibits contrastive nasality in the vowel system and is head marking and predominantly agglutinative. Bound pronominal forms marking arguments in the clause have the same form as bound pronominals marking possessors. Subordinate clauses typically involve nominalized verbs. Unlike most of its Amazonian neighbors, on the other hand, it does not have a semantically-based classifier or gender system but instead features arbitrarily assigned masculine or feminine gender. It also does not feature any incorporation of nouns, adverbs, or adpositions. It has an extensive oblique case-marking system, though core case-marking does not occur. More similar to Quechua and other Andean languages, it features a complex predicate-argument agreement system in which one or more agreement suffixes cross-reference the subject and object arguments of a transitive verb. It also has a large class of lexical numbers following a decimal numeral system.
View details
Preview abstract
Almost none of the 2,000+ languages spoken in Africa have widely available automatic speech recognition systems, and the required data is also only available for a few languages. We have experimented with two techniques which may provide pathways to large vocabulary speech recognition for African languages: multilingual modeling and self-supervised learning. We gathered available open source data and collected data for 15 languages, and trained experimental models using these techniques. Our results show that pooling the small amounts of data available in multilingual end-to-end models, and pre-training on unsupervised data can help improve speech recognition quality for many African languages.
View details
XTREME-S: Evaluating Cross-lingual Speech Representations
Clara E. Rivera
Mihir Sanjay Kale
Sebastian Ruder
Simran Khanuja
Ye Jia
Yu Zhang
Proc. Interspeech 2022
Preview abstract
We introduce \xtremes, a new benchmark to evaluate universal cross-lingual speech representations in many languages. XTREME-S covers four task families: speech recognition, classification, retrieval and speech-to-text translation. Covering 102 languages from 10+ language families, 3 different domains and 4 task families, XTREME-S aims to simplify multilingual speech representation evaluation, as well as catalyze research in ``universal'' speech representation learning. This paper describes the new benchmark and establishes the first speech-only and speech-text baselines using XLS-R and mSLAM on all downstream tasks. We motivate the design choices and detail how to use the benchmark. The code and pre-processing scripts will be made publicly available.\footnote{\small\url{https://huggingface.co/datasets/google/xtreme_s}}
View details
Preview abstract
Pronunciation modeling is a key task for building speech technology in new languages, and while solid grapheme-to-phoneme (G2P) mapping systems exist, language coverage can stand to be improved. The information needed to build G2P models for many more languages can easily be found on Wikipedia, but unfortunately, it is stored in disparate formats. We report on a system we built to mine a pronunciation data set in 819 languages from loosely structured tables within Wikipedia. The data includes phoneme inventories, and for 63 low-resource languages, also includes the grapheme-to-phoneme (G2P) mapping. 54 of these languages do not have easily findable G2P mappings online otherwise. We turned the information from Wikipedia into a structured, machine-readable TSV format, and make the resulting data set publicly available so it can be improved further and used in a variety of applications involving low-resource languages.
View details
Preview abstract
Training data for machine learning models can come from many different sources, which can be of dubious quality. For resource-rich languages like English, there is a lot of data available, so we can afford to throw out the dubious data. For low-resource languages where there is much less data available, we can't necessarily afford to throw out the dubious data, in case we end up with a training set which is too small to train a model. In this study, we examine the effects of text normalization and data set quality for a set of low-resource languages of Africa -- Afrikaans, Amharic, Hausa, Igbo, Malagasy, Somali, Swahili, and Zulu. We describe our text normalizer which we built in the Pynini framework, a Python library for finite state transducers, and our experiments in training language models for African languages using the Natural Language Toolkit (NLTK), an open-source Python library for NLP.
View details
Data-Driven Parametric Text Normalization: Rapidly Scaling Finite-State Transduction Verbalizers to New Languages
Kim Anne Heiligenstein
Nikos Bampounis
Christian Schallhart
Jonas Fromseier Mortensen
Proceedings of the 1st Joint SLTU and CCURL Workshop (SLTU-CCURL 2020), Language Resources and Evaluation Conference (LREC 2020), Marseille, 218–225
Preview abstract
This paper presents a methodology for rapidly generating FST-based verbalizers for ASR and TTS systems by efficiently sourcing language-specific data. We describe a questionnaire which collects the necessary data to bootstrap the number grammar induction system and parameterize the verbalizer templates described in Ritchie et al. (2019), and a machine-readable data store which allows the data collected through the questionnaire to be supplemented by additional data from other sources. We also discuss the benefits of this system for low-resource languages.
View details
Preview abstract
When building automatic speech recognition (ASR) systems, typically some amount of audio and text data in the target language is needed. While text data can be obtained relatively easily across many languages, transcribed audio data is challenging to obtain. This presents a barrier to making voice technologies available in more languages of the world. In this paper, we present a way to build an ASR system for a language even in the absence of any audio training data in that language at all. We do this by simply re-using an existing acoustic model from a phonologically similar language, without any kind of modification or adaptation towards the target language. The basic insight is that, if two languages are sufficiently similar in terms of their phonological system, an acoustic model should hold up relatively well when used for another language. We describe how we tailor our pronunciation models to enable such re-use, and show experimental results across a number of languages from various language families. We also provide a theoretical analysis of situations in which this approach is likely to work. Our results show that is possible to achieve less than 20% word error rate (WER) using this method.
View details