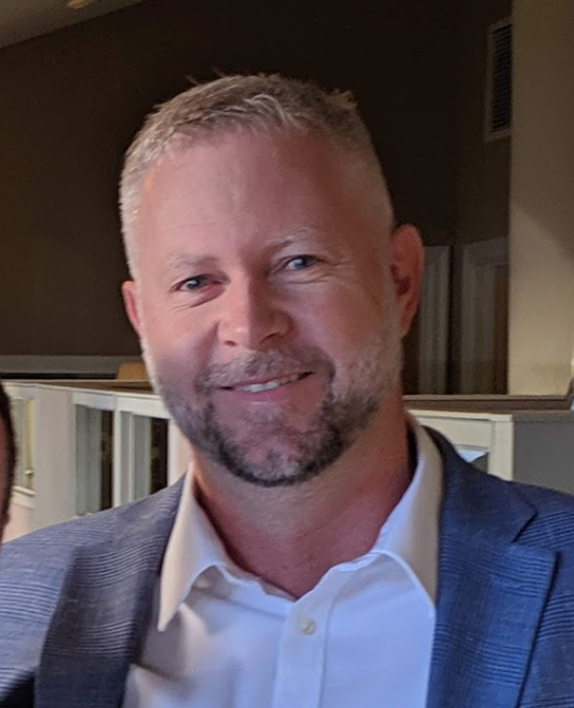
Ben Hutchinson
Ben Hutchinson is a Research Scientist in Google's Research, in Google's Responsible AI and Human-Centered Technology team. His research includes learning from various disciplines to inform the ethical development of AI. Prior to joining Google Research, he spent ten years working on a variety of products such as Google Wave, Google Maps, Knowledge Graph, Google Search, Social Impact, and others. He now uses this experience to work closely with product teams as a consultant on responsible practices and the development of responsible data sets machine learning models. He has a PhD in Natural Language Processing from the University of Edinburgh, and undergraduate degrees in linguistics and mathematics.
Authored Publications
Sort By
Preview abstract
Testing, within the machine learning (ML) community, has been predominantly about assessing a learned model's predictive performance measured against a test dataset. This test dataset is often a held-out subset of the dataset used to train the model, and hence expected to follow the same data distribution as the training dataset. While recent work on robustness testing within ML has pointed to the importance of testing against distributional shifts, these efforts also focus on estimating the likelihood of the model making an error against a reference dataset/distribution. In this paper, we argue that this view of testing actively discourages researchers and developers from looking into many other sources of robustness failures, for instance corner cases. We draw parallels with decades of work within software engineering testing focused on assessing a software system against various stress conditions, including corner cases, as opposed to solely focusing on average-case behaviour. Finally, we put forth a set of recommendations to broaden the view of machine learning testing to a rigorous practice.
View details
Preview abstract
In order to build trust that a machine learned model is appropriate and responsible within a systems context involving technical and
human components, a broad range of factors typically need to be considered. However in practice model evaluations frequently focus
on only a narrow range of expected predictive behaviours. This paper examines the critical evaluation gap between the idealized
breadth of concerns and the observed narrow focus of actual evaluations. In doing so, we demonstrate which values are centered—and
which are marginalized—within the machine learning community. Through an empirical study of machine learning papers from
recent high profile conferences, we demonstrate the discipline’s general focus on a small set of evaluation methods. By considering the
mathematical formulations of evaluation metrics and the test datasets over which they are calculated, we draw attention to which
properties of models are centered in the field. This analysis also reveals an important gap: the properties of models which are frequently
neglected or sidelined during evaluation. By studying the structure of this gap, we demonstrate the machine learning discipline’s
implicit assumption of a range of commitments which have normative impacts; these include commitments to consequentialism,
abstractability from context, the quantifiability of impacts, the irrelevance of non-predictive features, and the equivalence of different
failure modes. Shedding light on these assumptions and commitments enables us to question their appropriateness for different ML
system contexts, and points the way towards more diverse and contextualized evaluation methodologies which can be used to more
robustly examine the trustworthiness of ML models.
View details
LaMDA: Language Models for Dialog Applications
Aaron Daniel Cohen
Alena Butryna
Alicia Jin
Apoorv Kulshreshtha
Ben Zevenbergen
Chung-ching Chang
Cosmo Du
Daniel De Freitas Adiwardana
Dehao Chen
Dmitry (Dima) Lepikhin
Erin Hoffman-John
Igor Krivokon
James Qin
Jamie Hall
Joe Fenton
Johnny Soraker
Kathy Meier-Hellstern
Maarten Paul Bosma
Marc Joseph Pickett
Marcelo Amorim Menegali
Marian Croak
Maxim Krikun
Noam Shazeer
Rachel Bernstein
Ravi Rajakumar
Ray Kurzweil
Romal Thoppilan
Steven Zheng
Taylor Bos
Toju Duke
Tulsee Doshi
Vincent Y. Zhao
Will Rusch
Yuanzhong Xu
Zhifeng Chen
arXiv (2022)
Preview abstract
We present LaMDA: Language Models for Dialog Applications. LaMDA is a family of Transformer-based neural language models specialized for dialog, which have up to 137B parameters and arepre-trained on 1.56T words of public dialog data and web text. While model scaling alone canimprove quality, it shows less improvements on safety and factual grounding. We demonstrate thatfine-tuning with annotated data and enabling the model to consult external knowledge sources canlead to significant improvements towards the two key challenges of safety and factual grounding.The first challenge, safety, involves ensuring that the model’s responses are consistent with a set ofhuman values, such as preventing harmful suggestions and unfair bias. We quantify safety using ametric based on an illustrative set of values, and we find that filtering candidate responses using aLaMDA classifier fine-tuned with a small amount of crowdworker-annotated data offers a promisingapproach to improving model safety. The second challenge, factual grounding, involves enabling themodel to consult external knowledge sources, such as an information retrieval system, a languagetranslator, and a calculator. We quantify factuality using a groundedness metric, and we find that ourapproach enables the model to generate responses grounded in known sources, rather than responsesthat merely sound plausible. Finally, we explore the use of LaMDA in the domains of education andcontent recommendations, and analyze their helpfulness and role consistency.
View details
Preview abstract
Questions regarding implicitness, ambiguity and underspecification are crucial for multimodal image+text systems, but have received little attention to date. This paper maps out a conceptual framework to address this gap for systems which generate images from text inputs, specifically for systems which generate images depicting scenes from descriptions of those scenes. In doing so, we account for how texts and images convey different forms of meaning. We then outline a set of core challenges concerning textual and visual ambiguity and specificity tasks, as well as risks that may arise from improper handling of ambiguous and underspecified elements. We propose and discuss two strategies for addressing these challenges: a) generating a visually ambiguous output image, and b) generating a set of diverse output images.
View details
PaLM: Scaling Language Modeling with Pathways
Aakanksha Chowdhery
Sharan Narang
Jacob Devlin
Maarten Bosma
Hyung Won Chung
Sebastian Gehrmann
Parker Schuh
Sasha Tsvyashchenko
Abhishek Rao
Yi Tay
Noam Shazeer
Nan Du
Reiner Pope
James Bradbury
Guy Gur-Ari
Toju Duke
Henryk Michalewski
Xavier Garcia
Liam Fedus
David Luan
Barret Zoph
Ryan Sepassi
David Dohan
Shivani Agrawal
Mark Omernick
Marie Pellat
Aitor Lewkowycz
Erica Moreira
Rewon Child
Oleksandr Polozov
Zongwei Zhou
Brennan Saeta
Michele Catasta
Jason Wei
Kathy Meier-Hellstern
arxiv:2204.02311 (2022)
Preview abstract
Large language models have been shown to achieve remarkable performance across a variety of natural language tasks using few-shot learning, which drastically reduces the number of task-specific training examples needed to adapt the model to a particular application. To further our understanding of the impact of scale on few-shot learning, we trained a 540-billion parameter, densely activated, Transformer language model, which we call Pathways Language Model PaLM. We trained PaLM on 6144 TPU v4 chips using Pathways, a new ML system which enables highly efficient training across multiple TPU Pods. We demonstrate continued benefits of scaling by achieving state-of-the-art few-shot learning results on hundreds of language understanding and generation benchmarks. On a number of these tasks, PaLM 540B achieves breakthrough performance, outperforming the finetuned state-of-the-art on a suite of multi-step reasoning tasks, and outperforming average human performance on the recently released BIG-bench benchmark. A significant number of BIG-bench tasks showed discontinuous improvements from model scale, meaning that performance steeply increased as we scaled to our largest model. PaLM also has strong capabilities in multilingual tasks and source code generation, which we demonstrate on a wide array of benchmarks. We additionally provide a comprehensive analysis on bias and toxicity, and study the extent of training data memorization with respect to model scale. Finally, we discuss the ethical considerations related to large language models and discuss potential mitigation strategies.
View details
Preview abstract
Conventional algorithmic fairness is West-centric, as seen in its sub-groups, values, and optimisations. In this paper, we de-center algorithmic fairness and analyse AI power in India. Based on 36 qualitative interviews and a discourse analysis of algorithmic deployments in India, we find that several assumptions of algorithmic fairness are challenged in India. We find that data is not always reliable due to socio-economic factors, users are given third world treatment by ML makers, and AI signifies unquestioning aspiration. We contend that localising model fairness alone can be window dressing in India, where the distance between models and oppressed communities is large. Instead, we re-imagine algorithmic fairness in India and provide a roadmap to re-contextualise data and models, empower oppressed communities, and enable Fair-ML ecosystems.
View details
Towards Accountability for Machine Learning Datasets
Alex Hanna
Christina Greer
Margaret Mitchell
Proceedings of FAccT 2021 (2021) (to appear)
Preview abstract
Rising concern for the societal implications of artificial intelligence systems has inspired demands for greater transparency and accountability. However the datasets which empower machine learning are often used, shared and re-used with little visibility into the processes of deliberation which led to their creation. Which stakeholder groups had their perspectives included when the dataset was conceived? Which domain experts were consulted regarding how to model subgroups and other phenomena? How were questions of representational biases measured and addressed? Who labeled the data? In this paper, we introduce a rigorous framework for dataset development transparency which supports decision-making and accountability. The framework uses the cyclical, infrastructural and engineering nature of dataset development to draw on best practices from the software development lifecycle. Each stage of the data development lifecycle yields a set of documents that facilitate improved communication and decision-making, as well as drawing attention the value and necessity of careful data work. The proposed framework is intended to contribute to closing the accountability gap in artificial intelligence systems, by making visible the often overlooked work that goes into dataset creation.
View details
Preview abstract
In this paper we argue that standard calls for explainability that focus on the epistemic inscrutability of black-box machine learning models may be misplaced. If we presume, for the sake of this paper, that machine learning can be a source of knowledge, then it makes sense to wonder what kind of justification it involves. How do we rationalize on the one hand the seeming justificatory black box with the observed widespread adoption of machine learning? We argue that, in general, people implicitly adopt reliabilism regarding machine learning. Reliabilism is an epistemological theory of epistemic justification according to which a belief is warranted if it has been produced by a reliable process or method. We argue that, in cases where model deployments require moral justification, reliabilism is not sufficient, and instead justifying deployment requires establishing robust human processes as a moral “wrapper” around machine outputs. We then suggest that, in certain high-stakes domains with moral consequences, reliabilism does not provide another kind of necessary justification—moral justification. Finally, we offer cautions relevant to the (implicit or explicit)adoption of the reliabilist interpretation of machine learning.
View details
Social Biases in NLP Models as Barriers for Persons with Disabilities
Yu Zhong
Stephen Craig Denuyl
Proceedings of ACL 2020, ACL (to appear)
Preview abstract
Building equitable and inclusive technologies
demands paying attention to how social attitudes towards persons with disabilities are
represented within technology. Representations perpetuated by NLP models often inadvertently encode undesirable social biases
from the data on which they are trained. In this
paper, first we present evidence of such undesirable biases towards mentions of disability in
two different NLP models: toxicity prediction
and sentiment analysis. Next, we demonstrate
that neural embeddings that are critical first
steps in most NLP pipelines also contain undesirable biases towards mentions of disabilities.
We then expose the topical biases in the social
discourse about some disabilities which may
explain such biases in the models; for instance,
terms related to gun violence, homelessness,
and drug addiction are over-represented in discussions about mental illness.
View details
Closing the AI accountability gap: defining an end-to-end framework for internal algorithmic auditin
Becky White
Inioluwa Deborah Raji
Margaret Mitchell
Timnit Gebru
FAT* Barcelona, 2020, ACM Conference on Fairness, Accountability, and Transparency (ACM FAT* (2020)
Preview abstract
Rising concern for the societal implications of artificial intelligencesystems has inspired a wave of academic and journalistic literaturein which deployed systems are audited for harm by investigatorsfrom outside the organizations deploying the algorithms. However,it remains challenging for practitioners to identify the harmfulrepercussions of their own systems prior to deployment, and, oncedeployed, emergent issues can become difficult or impossible totrace back to their source.In this paper, we introduce a framework for algorithmic auditingthat supports artificial intelligence system development end-to-end,to be applied throughout the internal organization development life-cycle. Each stage of the audit yields a set of documents that togetherform an overall audit report, drawing on an organization’s valuesor principles to assess the fit of decisions made throughout the pro-cess. The proposed auditing framework is intended to contribute toclosing theaccountability gapin the development and deploymentof large-scale artificial intelligence systems by embedding a robustprocess to ensure audit integrity.
View details