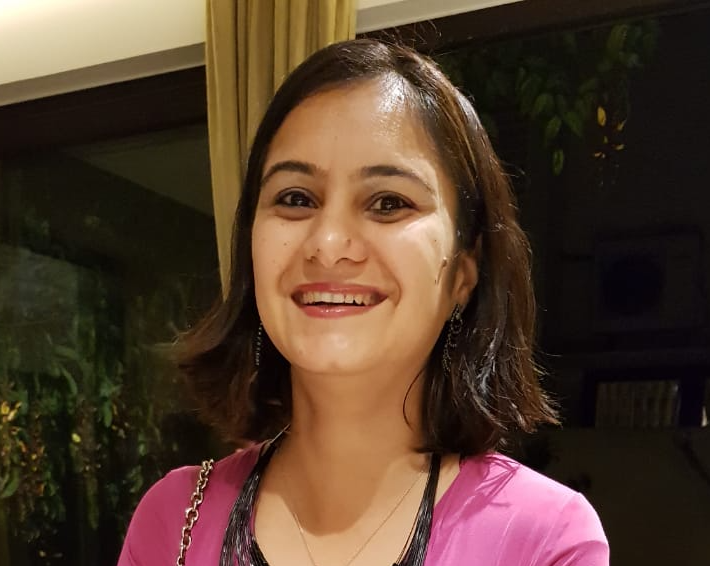
Aparna Taneja
I've been with Google since 2015. I am a researcher with the AI for social good team in Google Research India. My current research focus is to drive innovation in AI to achieve real world social impact. Our team collaborates with NGOs in public health, conservation and agriculture. One of our ongoing collaborations is with the NGO called ARMMAN with the mission to improve maternal and child health outcomes in underserved communities of India.
My earlier projects involved improving search quality on Google Maps, and using satellite imagery for applications in remote sensing. Prior to Google I was a Post Doc at Disney Research Zurich. I completed my PhD from the Computer Vision and Geometry Group in ETH Zurich and Bachelors in Computer Science from the Indian Institute of Technology, Delhi.
Research Areas
Authored Publications
Sort By
Scalable Decision-Focused Learning in Restless Multi-Armed Bandits with Application to Maternal and Child Care
Kai Wang
Shresth Verma
Aditya S. Mate
Sanket Shah
Neha Madhiwalla
Aparna Hegde
AAAI 2023 (to appear)
Preview abstract
This paper studies restless multi-armed bandit (RMAB) problems with unknown arm transition dynamics but with known correlated arm features. The goal is to learn a model to predict transition dynamics given features, where the Whittle index policy solves the RMAB problems using predicted transitions. However, prior works often learn the model by maximizing the predictive accuracy instead of final RMAB solution quality, causing a mismatch between training and evaluation objectives. To address this shortcoming, we propose a novel approach for decision-focused learning in RMAB that directly trains the predictive model to maximize the Whittle index solution quality. We present three key contributions: (i)
we establish differentiability of the Whittle index policy to support decision-focused learning; (ii) we significantly improve the scalability of decision-focused learning approaches in sequential problems, specifically RMAB problems; (iii) we apply our algorithm to a previously collected dataset of maternal and child health to demonstrate its performance. Indeed, our algorithm is the first for decision-focused learning in RMAB that scales to real-world problem sizes.
View details
Analyzing and Predicting Low-Listenership Trends in a Large-Scale Mobile Health Program: A Preliminary Investigation
Arshika Lalan
Shresth Verma
Kumar Madhu Sudan
Amrita Mahale
Aparna Hegde
The Workshop in Data Science for Social Good, KDD 2023 (2023)
Preview abstract
Mobile health programs are becoming an increasingly popular medium
for dissemination of health information among beneficiaries in less
privileged communities. Kilkari is one of the world’s largest mobile
health programs which delivers time sensitive audio-messages to
pregnant women and new mothers. We have been collaborating with
ARMMAN, a non-profit in India which operates the Kilkari program,
to identify bottlenecks to improve the efficiency of the program. In
particular, we provide an initial analysis of the trajectories of benefi-
ciaries’ interaction with the mHealth program and examine elements
of the program that can be potentially enhanced to boost its success.
We cluster the cohort into different buckets based on listenership
so as to analyze listenership patterns for each group that could help
boost program success . We also demonstrate preliminary results on
using historical data in a time-series prediction to identify benefi-
ciary dropouts and enable NGOs in devising timely interventions to
strengthen beneficiary retention.
View details
Preview abstract
We consider the task of effect estimation of resource allocation algorithms through clinical trials. Such algorithms are tasked with optimally
utilizing severely limited intervention resources,
with the goal of maximizing their overall benefits
derived. Evaluation of such algorithms through
clinical trials proves difficult, notwithstanding the
scale of the trial, because the agents’ outcomes are
inextricably linked through the budget constraint
controlling the intervention decisions. Towards
building more powerful estimators with improved
statistical significance estimates, we propose a
novel concept involving retrospective reshuffling
of participants across experimental arms at the
end of a clinical trial. We identify conditions
under which such reassignments are permissible
and can be leveraged to construct counterfactual
clinical trials, whose outcomes can be accurately
‘observed’ without uncertainty, for free. We prove
theoretically that such an estimator is more accurate than common estimators based on sample
means — we show that it returns an unbiased estimate and simultaneously reduces variance. We
demonstrate the value of our approach through
empirical experiments on both, real case studies
as well as synthetic and realistic data sets and
show improved estimation accuracy across the
board.
View details
Adherence Bandits
Jackson A. Killian*
Arshika Lalan*
Aditya Mate*
Manish Jain
The Workshop on Artificial Intelligence for Social Good at AAAI 2023 (2023)
Preview abstract
We define a new subclass of the restless multi-armed bandit framework, that we name Adherence Bandits, designed to capture the dynamics prevalent in many public health intervention problems. We discuss key properties of Adherence Bandits, their real-world motivations, how structures lead to both technical and computational advantages, and natural extensions that have been or can be made to the subclass. We summarise key research works that have contributed to the growing sub-area and finish by highlighting future directions of research
View details
Preview abstract
Restless multi-armed bandits (RMABs) are an extension of multi-armed bandits (MABs) with state information associated with arms, where the states evolve restlessly with different transition probabilities depending on whether the arms are pulled. The additional state information in RMABs captures broader applications with state dependency, including digital marketing and healthcare recommendation. However, solving RMABs requires information on transition dynamics, which is often not available upfront. This paper considers learning the transition probabilities in an RMAB setting while maintaining small regret. We use the confidence bounds of transition probabilities to define an optimistic Whittle index policy to solve the RMAB problem while maintaining sub-linear regret compared to the benchmark. Our algorithm, UCWhittle, leverages the structure of RMABs and the Whittle index policy solution to achieve better performance than other online learning baselines without structural information. We evaluate UCWhittle on real-world healthcare data to help reduce maternal mortality.
View details
Flexible Budgets in Restless Bandits: A Primal-Dual Algorithm for Efficient Budget Allocation
Jackson Killian
Lily Xu
Paula Rodriguez Diaz
AAAI 2023 (to appear)
Preview abstract
Restless Multi-Armed Bandits (RMABs) are an important model that enable optimizing allocation of limited resources in sequential decision-making settings. Typical RMABs assume the budget --- the number of arms pulled --- per round to be fixed for each step in the planning horizon. However, when planning in real-world settings, resources are not necessarily limited at each planning step; we may be able to distribute surplus resources in one round to an earlier or later round. Often this flexibility in budget is constrained to within a subset of consecutive planning steps. In this paper we define a general class of RMABs with flexible budget, which we term F-RMABs, and provide an algorithm to optimally solve for them. Additionally, we provide heuristics that tradeoff solution quality for efficiency and present experimental comparisons of different F-RMAB solution approaches.
View details
Robust Planning over Restless Groups: Engagement Interventions for a Large-Scale Maternal Telehealth Program
Jackson Killian
Lily Xu
Arpita Biswas
Shresth Verma
Vineet Nair
Aparna Hegde
Neha Madhiwalla
Paula Rodriguez Diaz
Sonja Johnson-Yu
AAAI 2023 (to appear)
Preview abstract
In 2020, maternal mortality in India was estimated to be as high as 130 deaths per 100K live births, nearly twice the UN’s target. To improve health outcomes, the non-profit ARMMAN sends automated voice messages to expecting and new mothers across India. However, 38% of mothers stop listening to these calls, missing critical preventative care information. To improve engagement, ARMMAN employs health workers to intervene by making service calls, but workers can only call a fraction of the 100K enrolled mothers. Partnering with ARMMAN, we model the problem of allocating limited interventions across mothers as a restless multi-armed bandit (RMAB), where the realities of large scale and model uncertainty present key new technical challenges. We address these with GROUPS, a double oracle–based algorithm for robust planning in RMABs with scalable grouped arms. Robustness over grouped arms requires several methodological advances. First, to adversarially select stochastic group dynamics, we develop a new method to optimize Whittle indices over transition probability intervals. Second, to learn group level RMAB policy best responses to these adversarial environments, we introduce a weighted index heuristic. Third, we prove a key theoretical result that planning over grouped arms achieves the same minimax regret–optimal strategy as planning over individual arms, under a technical condition. Finally, using real world data from ARMMAN, we show that GROUPS produces robust policies that reduce minimax regret by up to 50%, halving the number of preventable missed voice messages to connect more mothers with life saving maternal health information.
View details
Deployed SAHELI: Field Optimization of Intelligent RMAB for Maternal and Child Care
Shresth Verma
Aditya S. Mate
Paritosh Verma
Sruthi Gorantala
Neha Madhiwalla
Aparna Hegde
Manish Jain
Innovative Applications of Artificial Intelligence (IAAI) (2023) (to appear)
Preview abstract
Underserved communities face critical health challenges due to lack of access to timely and reliable information. Non-governmental organizations are leveraging the widespread use of cellphones to combat these healthcare challenges and spread preventative awareness. The health workers at these organizations reach out individually to beneficiaries; however such programs still suffer from declining engagement. We have deployed SAHELI, a system to efficiently utilize the limited availability of health workers for improving maternal and child health in India. SAHELI uses the Restless Multi-armed Bandit (RMAB) framework to identify beneficiaries for outreach. It is the first deployed application for RMABs
in public health, and is already in continuous use by our partner NGO, ARMMAN. We have already reached ∼ 100K beneficiaries with SAHELI, and are on track to serve 1 million beneficiaries by the end of 2023. This scale and impact has been achieved through multiple innovations in the RMAB model and its development, in preparation of real world data, and in deployment practices; and through careful consideration of responsible AI practices. Specifically, in this paper, we describe our approach to learn from past data to improve the performance of SAHELI’s RMAB model, the real-world challenges faced during deployment and adoption of SAHELI, and the end-to-end pipeline
View details
Facilitating Human-Wildlife Cohabitation through Conflict Prediction
Susobhan Ghosh
Pradeep Varakantham
Aniket Bhatkhande
Tamanna Ahmad
Anish Andheria
Wenjun Li
IAAI Technical Track on Emerging Applications of AI (2022)
Preview abstract
With increasing world population and expanded use of forests as cohabited regions, interactions and conflicts with wildlife are increasing, leading to large scale loss of lives (animal and human) and livelihoods (economic). While community knowledge is valuable, forest officials and conservation organisations can greatly benefit from predictive analysis of human-wildlife conflict, leading to targeted interventions that can potentially help save lives and livelihoods. However, the problem of prediction is a complex socio-technical problem in the context of limited data in low-resource regions. Identifying the right features to make accurate predictions of conflicts at the required spatial granularity using a sparse conflict training dataset is the key challenge that we address in this paper. Specifically, we do an illustrative case study on human-wildlife conflicts in the Bramhapuri Forest Division in Chandrapur, Maharashtra, India. Most existing work has considered human wildlife conflicts in protected areas and to the best of our knowledge, this is the first effort at prediction of human-wildlife conflicts in unprotected areas and using those predictions for deploying interventions on the ground.
View details
Field Study in Deploying Restless Multi-Armed Bandits: Assisting Non-Profits in Improving Maternal and Child Health
Aditya Mate
Lovish Madaan
Neha Madhiwalla
Shresth Verma
Aparna Hegde
Pradeep Varakantham
AAAI Conference on Artificial Intelligence (2022) (to appear)
Preview abstract
The widespread availability of cell phones has enabled non-profits to deliver critical health information to their beneficiaries in a timely manner. This paper describes our work to assist non-profits that employ automated messaging programs to deliver timely preventive care information to beneficiaries (new and expecting mothers) during pregnancy and after delivery. Unfortunately, a key challenge in such information delivery programs is that a significant fraction of beneficiaries drop out of the program. Yet, non-profits often have limited health-worker resources (time) to place crucial service calls for live interaction with beneficiaries to prevent such engagement drops. To assist non-profits in optimizing this limited resource, we developed a Restless Multi-Armed Bandits (RMABs) system. One key technical contribution in this system is a novel clustering method of offline historical data to infer unknown RMAB parameters. Our second major contribution is evaluation of our RMAB system in collaboration with an NGO, via a real-world service quality improvement study. The study compared strategies for optimizing service calls to 23003 participants over a period of 7 weeks to reduce engagement drops. We show that the RMAB group provides statistically significant improvement over other comparison groups, reducing 30% engagement drops. To the best of our knowledge, this is the first study demonstrating the utility of RMABs in real world public health settings. We are transitioning our RMAB system to the NGO for real-world use.
View details