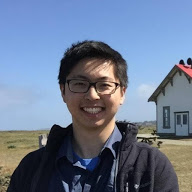
Ted Xiao
Research Areas
Authored Publications
Sort By
Deep RL at Scale: Sorting Waste in Office Buildings with a Fleet of Mobile Manipulators
Jarek Rettinghouse
Daniel Ho
Julian Ibarz
Sangeetha Ramesh
Matt Bennice
Alexander Herzog
Chuyuan Kelly Fu
Adrian Li
Kim Kleiven
Jeff Bingham
Yevgen Chebotar
David Rendleman
Wenlong Lu
Mohi Khansari
Mrinal Kalakrishnan
Ying Xu
Noah Brown
Khem Holden
Justin Vincent
Peter Pastor Sampedro
Jessica Lin
David Dovo
Daniel Kappler
Mengyuan Yan
Sergey Levine
Jessica Lam
Jonathan Weisz
Paul Wohlhart
Karol Hausman
Cameron Lee
Bob Wei
Yao Lu
Preview abstract
We describe a system for deep reinforcement learning of robotic manipulation skills applied to a large-scale real-world task: sorting recyclables and trash in office buildings. Real-world deployment of deep RL policies requires not only effective training algorithms, but the ability to bootstrap real-world training and enable broad generalization. To this end, our system combines scalable deep RL from real-world data with bootstrapping from training in simulation, and incorporates auxiliary inputs from existing computer vision systems as a way to boost generalization to novel objects, while retaining the benefits of end-to-end training. We analyze the tradeoffs of different design decisions in our system, and present a large-scale empirical validation that includes training on real-world data gathered over the course of 24 months of experimentation, across a fleet of 23 robots in three office buildings, with a total training set of 9527 hours of robotic experience. Our final validation also consists of 4800 evaluation trials across 240 waste station configurations, in order to evaluate in detail the impact of the design decisions in our system, the scaling effects of including more real-world data, and the performance of the method on novel objects.
View details
Robotic Skill Acquisition via Instruction Augmentation with Vision-Language Models
Harris Chan
Anthony Brohan
Karol Hausman
Sergey Levine
RSS 2023 (2023)
Preview abstract
In recent years, much progress has been made in learning robotic manipulation policies that can follow natural language instructions.
Common approaches involve learning methods that operate on offline datasets, such as task-specific teleoperated demonstrations or on hindsight labeled robotic experience.
Such methods work reasonably but rely strongly on the assumption of clean data: teleoperated demonstrations are collected with specific tasks in mind, while hindsight language descriptions rely on expensive human labeling.
Recently, large-scale pretrained language and vision-language models like CLIP have been applied to robotics in the form of learning representations and planners.
However, can these pretrained models also be used to cheaply impart internet-scale knowledge onto offline datasets, providing access to skills contained in the offline dataset that weren't necessarily reflected in ground truth labels?
We investigate fine-tuning a reward model on a small dataset of robot interactions with crowd-sourced natural language labels and using the model to relabel instructions of a large offline robot dataset.
The resulting dataset with diverse language skills is used to train imitation learning policies, which outperform prior methods by up to 30% when evaluated on a diverse set of novel language instructions that were not contained in the original dataset.
View details
PI-QT-Opt: Predictive Information Improves Multi-Task Robotic Reinforcement Learning at Scale
Adrian Li
Paul Wohlhart
Yao Lu
Conference on Robot Learning (CoRL) (2022)
Preview abstract
The predictive information, the mutual information between the past and future, has been shown to be a useful representation learning auxiliary loss for training reinforcement learning agents, as the ability to model what will happen next is critical to success on many control tasks. While existing studies are largely restricted to training specialist agents on single-task settings in simulation, in this work, we study modeling the predictive information for robotic agents and its importance for general-purpose agents that are trained to master a large repertoire of diverse skills from large amounts of data. Specifically, we introduce Predictive Information QT-Opt (PI-QT-Opt), a QT-Opt agent augmented with an auxiliary loss that learns representations of the predictive information to solve up to 297 vision-based robot manipulation tasks in simulation and the real world with a single set of parameters. We demonstrate that modeling the predictive information significantly improves success rates on the training tasks and leads to better zero-shot transfer to unseen novel tasks. Finally, we evaluate PI-QT-Opt on real robots, achieving substantial and consistent improvement over QT-Opt in multiple experimental settings of varying environments, skills, and multi-task configurations.
View details
Value Function Spaces: Skill-Centric State Abstractions for Long-Horizon Reasoning
Alexander Toshkov Toshev
Brian Andrew Ichter
Dhruv Shah
Peng Xu
Sergey Levine
Yao Lu
ICLR (2022)
Preview abstract
Reinforcement learning can train policies that effectively perform complex tasks. However, the performance of these methods degrades as the horizon increases, and performing long-horizon tasks often requires reasoning over and composing multiple lower-level skills. Hierarchical reinforcement learning aims to enable this, by providing a bank of low-level skills as action abstractions, in the form of primitives or options.
However, an effective hierarchy should exhibit abstraction both in the space of actions and states. We posit that a suitable state abstraction for the higher-level policy should depend on the capabilities of the available lower-level policies, and we propose an approach that produces such a representation by using the value functions corresponding to each lower-level skill to capture the affordances for these skills.
Empirical evaluations for maze-solving and robotic manipulation tasks demonstrate that our approach improves long-horizon performance and enables better zero-shot generalization than popular model-free and model-based methods by constructing a compact state abstraction that represents the affordances of the scene and is robust to distractors.
We implement our approach in two domains: a long-horizon maze solving task, and a complex image-based robotic manipulation simulator. In both settings, we show empirically that, when provided with a suitable bank of skills, our approach enables more effective long-horizon control as compared to alternative state representation learning methods.
View details
Jump-Start Reinforcement Learning
Ike Uchendu
Yao Lu
Banghua Zhu
Mengyuan Yan
Joséphine Simon
Matt Bennice
Chuyuan Kelly Fu
Cong Ma
Jiantao Jiao
Sergey Levine
Karol Hausman
NeurIPS 2021 Robot Learning Workshop, RSS 2022 Scaling Robot Learning Workshop
Preview abstract
Reinforcement learning (RL) provides a theoretical framework for continuously improving an agent's behavior via trial and error. However, efficiently learning policies from scratch can be very difficult, particularly for tasks that present exploration challenges. In such settings, it might be desirable to initialize RL with an existing policy, offline data, or demonstrations. However, naively performing such initialization in RL often works poorly, especially for value-based methods.
In this paper, we present a meta algorithm that can use offline data, demonstrations, or a pre-existing policy to initialize an RL policy, and is compatible with any RL approach.
In particular, we propose Jump-Start Reinforcement Learning (JSRL), an algorithm that employs two policies to solve tasks: a guide-policy, and an exploration-policy.
By using the guide-policy to form a curriculum of starting states for the exploration-policy, we are able to efficiently improve performance on a set of simulated robotic tasks.
In addition, we provide an upper bound on the sample complexity of JSRL and show that it is able to significantly outperform existing imitation and reinforcement learning algorithms, particularly in the small-data regime.
View details
InnerMonologue: Embodied Reasoning through Planning with Language Models
Wenlong Huang
Harris Chan
Jacky Liang
Pete Florence
Andy Zeng
Igor Mordatch
Yevgen Chebotar
Noah Brown
Tomas Jackson
Linda Luu
Sergey Levine
Karol Hausman
Brian Andrew Ichter
Conference on Robot Learning (2022) (to appear)
Preview abstract
Recent works have shown the capabilities of large language models to perform tasks requiring reasoning and to be applied to applications beyond natural language processing, such as planning and interaction for embodied robots.These embodied problems require an agent to understand the repertoire of skills available to a robot and the order in which they should be applied. They also require an agent to understand and ground itself within the environment.
In this work we investigate to what extent LLMs can reason over sources of feedback provided through natural language. We propose an inner monologue as a way for an LLM to think through this process and plan. We investigate a variety of sources of feedback, such as success detectors and object detectors, as well as human interaction. The proposed method is validated in a simulation domain and on real robotic. We show that Innerlogue can successfully replan around failures, and generate new plans to accommodate human intent.
View details
Do As I Can, Not As I Say: Grounding Language in Robotic Affordances
Alexander Herzog
Alexander Toshkov Toshev
Andy Zeng
Anthony Brohan
Brian Andrew Ichter
Byron David
Chelsea Finn
Clayton Tan
Diego Reyes
Dmitry Kalashnikov
Eric Victor Jang
Jarek Liam Rettinghouse
Jornell Lacanlale Quiambao
Julian Ibarz
Karol Hausman
Kyle Alan Jeffrey
Linda Luu
Mengyuan Yan
Michael Soogil Ahn
Nicolas Sievers
Noah Brown
Omar Eduardo Escareno Cortes
Peng Xu
Peter Pastor Sampedro
Rosario Jauregui Ruano
Sally Augusta Jesmonth
Sergey Levine
Steve Xu
Yao Lu
Yevgen Chebotar
Yuheng Kuang
Conference on Robot Learning (CoRL) (2022)
Preview abstract
Large language models can encode a wealth of semantic knowledge about the world. Such knowledge could in principle be extremely useful to robots aiming to act upon high-level, temporally extended instructions expressed in natural language.
However, a significant weakness of language models is that they lack contextual grounding, which makes it difficult to leverage them for decision making within a given real-world context.
For example, asking a language model to describe how to clean a spill might result in a reasonable narrative, but it may not be applicable to a particular agent, such as a robot, that needs to perform this task in a particular environment.
We propose to provide this grounding by means of pretrained behaviors, which are used to condition the model to propose natural language actions that are both feasible and contextually appropriate.
The robot can act as the language model’s “hands and eyes,” while the language model supplies high-level semantic knowledge about the task.
We show how low-level tasks can be combined with large language models so that the language model provides high-level knowledge about the procedures for performing complex and temporally extended instructions, while value functions associated with these tasks provide the grounding necessary to connect this knowledge to a particular physical environment.
We evaluate our method on a number of real-world robotic tasks, where we show that this approach is capable of executing long-horizon, abstract, natural-language tasks on a mobile manipulator.
The project's website and the video can be found at \url{say-can.github.io}.
View details
Actionable Models: Unsupervised Offline Learning of Robotic Skills
Benjamin Eysenbach
Chelsea Finn
Dmitry Kalashnikov
Jake Varley
Karol Hausman
Sergey Levine
Yao Lu
Yevgen Chebotar
International Conference on Machine Learning 2021 (2021)
Preview abstract
We consider the problem of learning useful robotic skills from previously collected offline data without access to manually specified rewards or additional online exploration, a setting that is becoming increasingly important for scaling robot learning by reusing past robotic data. In particular, we propose the objective of learning a functional understanding of the environment by learning to reach any goal state in a given dataset. We employ goal-conditioned Q-learning with hindsight relabeling and develop several techniques that enable training in a particularly challenging offline setting. We find that our method can operate on high-dimensional camera images and learn a variety of skills on real robots that generalize to previously unseen scenes and objects. We also show that our method can learn to reach long-horizon goals across multiple episodes, and learn rich representations that can help with downstream tasks through pre-training or auxiliary objectives.
View details
AW-Opt: Learning Robotic Skills with Imitationand Reinforcement at Scale
Yao Lu
Karol Hausman
Yevgen Chebotar
Mengyuan Yan
Eric Victor Jang
Alexander Herzog
Mohi Khansari
Dmitry Kalashnikov
Sergey Levine
Conference on Robot Learning 2021 (2021)
Preview abstract
This paper proposes a new algorithm "AW-Opt" to combine Imitation Learning (IL) and Reinforcement Learning (RL). Prior methods face significant difficulty with sparse reward, image based input robotics tasks. By carefully designing sample filtering strategy, exploration strategy, and bellman equation, AW-Opt outperforms existing SOTA algorithms. Experimental results in both simulation and with real robots show that AW-Opt can achieve reasonable success rate from initial demonstrations, maintain low inference time, fine tune to reach SOTA success rate and use much less samples than existing algorithms.
View details
Thinking While Moving: Deep Reinforcement Learning with Concurrent Control
Eric Victor Jang
Dmitry Kalashnikov
Sergey Levine
Julian Ibarz
Karol Hausman
Alexander Herzog
ICLR 2020
Preview abstract
We study reinforcement learning in settings where sampling an action from the policy must be done concurrently with the time evolution of the controlled system, such as when a robot must decide on the next action while still performing the previous action. Much like a person or an animal, the robot must think and move at the same time, deciding on its next action before the previous one has completed. In order to develop an algorithmic framework for such concurrent control problems, we start with a continuous-time formulation of the Bellman equations, and then discretize them in a way that is aware of system delays. We instantiate this new class of approximate dynamic programming methods via a simple architectural extension to existing value-based deep reinforcement learning algorithms. We evaluate our methods on simulated benchmark tasks and a large-scale robotic grasping task where the robot must "think while moving.''
View details