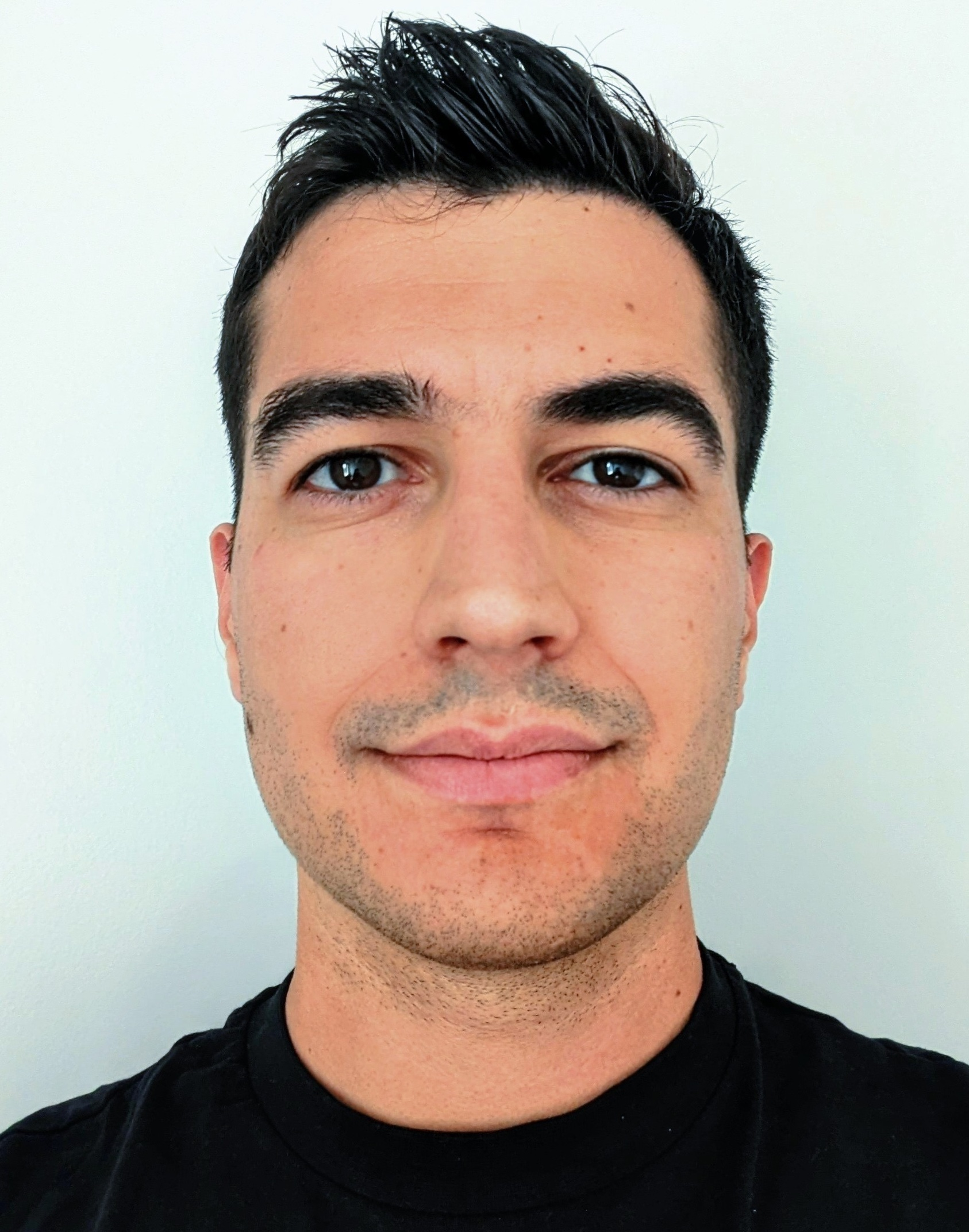
Efthymios Tzinis
Efthymios (Thymios) is a research scientist working on uni- and multi-modal sound separation and sound understanding in general. His research interests include the development of self-supervised audio and video perception machine learning models and their efficient deployment on low-energy mobile devices. He got his Ph.D. in Computer Science from the University of Illinois at Urbana-Champaign and also holds a diploma (B.Sc. + M.Eng.) in Electrical and Computer Engineering from the National Technical University of Athens. He has co-authored more than 30 papers in the field of audio and audio-visual processing and holds one US patent. Efthymios has also served as a reviewer to most of the top-notch journals and annual international conference-venues in the fields of deep learning and audio-visual processing and has also been recognized as an outstanding reviewer award 4 times. He has also been a past recipient of the Google Ph.D. fellowship for Machine Perception, Speech Technology and Computer Vision.
Research Areas
Authored Publications
Sort By
AudioScopeV2: Audio-Visual Attention Architectures for Calibrated Open-Domain On-Screen Sound Separation
Tal Remez
European Conference on Computer Vision (ECCV) (2022)
Preview abstract
We introduce AudioScopeV2, a state-of-the-art universal audio-visual on-screen sound separation system which is capable of learning to separate sounds and associate them with on-screen objects by looking at in-the-wild videos. We identify several limitations of previous work on audio-visual on-screen sound separation, including the coarse resolution of spatio-temporal attention, poor convergence of the audio separation model, limited variety in training and evaluation data, and failure to account for the trade off between preservation of on-screen sounds and suppression of off-screen sounds. We provide solutions to all of these issues. Our proposed cross-modal and self-attention network architectures capture audio-visual dependencies at a finer resolution over time, and we also propose efficient separable variants that are capable of scaling to longer videos without sacrificing much performance. We also find that pre-training the separation model only on audio greatly improves results. For training and evaluation, we collected new human annotations of onscreen sounds from a large database of in-the-wild videos (YFCC100M). This new dataset is more diverse and challenging. Finally, we propose a calibration procedure that allows exact tuning of on-screen reconstruction versus off-screen suppression, which greatly simplifies comparing performance between models with different operating points. Overall, our experimental results show marked improvements in on-screen separation performance under much more general conditions than previous methods with minimal additional computational complexity.
View details
Don’t Listen to What You Can’t See: The Importance of Negative Examples for Audio-Visual On-Screen Sound Separation
ECCV 2022 Workshop on AV4D: Visual Learning of Sounds in Spaces
Preview abstract
For the task of audio-visual on-screen sound separation, we illustrate the importance of using evaluation sets that includes not only positive examples (videos with on-screen sounds), but also negative examples (videos that only contain off-screen sounds). Given an evaluation set that includes such examples, we provide metrics and a calibration procedure to allow fair comparison of different models with a single metric, which is analogous to calibrating binary classifiers to achieve a desired false alarm rate. In addition, we propose a method of probing on-screen sound separation models by masking objects in input video frames. Using this method, we probe the sensitivity of our recently-proposed AudioScopeV2 model, and discover that its robustness to removing on-screen sound objects is improved by providing supervised examples in training.
View details
Into the Wild with AudioScope: Unsupervised Audio-Visual Separation of On-Screen Sounds
Tal Remez
International Conference on Learning Representations (ICLR) 2021
Preview abstract
Recent progress in deep learning has enabled many advances in sound separation and visual scene understanding. However, extracting sound sources which are apparent in natural videos remains an open problem. In this work, we present AudioScope, a novel audio-visual sound separation framework that can be trained without supervision to isolate on-screen sound sources from real in-the-wild videos. Prior audio-visual separation work assumed artificial limitations on the domain of sound classes (e.g., to speech or music), constrained the number of sources, and required strong sound separation or visual segmentation labels. AudioScope overcomes these limitations, operating on an open domain of sounds, with variable numbers of sources, and without labels or prior visual segmentation. The training procedure for AudioScope uses mixture invariant training (MixIT) to separate synthetic mixtures of mixtures (MoMs) into individual sources, where noisy labels for mixtures are provided by an unsupervised audio-visual coincidence model. Using the noisy labels, along with attention between video and audio features, AudioScope learns to identify audio-visual similarity and to suppress off-screen sounds. We demonstrate the effectiveness of our approach using a dataset of video clips extracted from open-domain YFCC100m video data. This dataset contains a wide diversity of sound classes recorded in unconstrained conditions, making the application of previous methods unsuitable. For evaluation and semi-supervised experiments, we collected human labels for presence of on-screen and off-screen sounds on a small subset of clips.
View details
Self-Supervised Audio-Visual Separation of On-Screen Sounds from Unlabeled Videos
Tal Remez
NeurIPS 2020 Workshop on Self-Supervised Learning for Speech and Audio Processing
Preview abstract
Recent progress in deep learning has enabled many advances in sound separation and visual scene understanding. However, extracting sound sources which are apparent in natural videos remains an open problem. In this work, we present AudioScope, a novel audio-visual sound separation framework that can be trained without supervision to isolate on-screen sound sources from real in-the-wild videos. Prior audio-visual separation work assumed artificial limitations on the domain of sound classes (e.g., to speech or music), constrained the number of sources, and required strong sound separation or visual segmentation labels. AudioScope overcomes these limitations, operating on an open domain of sounds, with variable numbers of sources, and without labels or prior visual segmentation. The training procedure for AudioScope uses mixture invariant training (MixIT) to separate synthetic mixtures of mixtures (MoMs) into individual sources, where noisy labels for mixtures are provided by an unsupervised audio-visual coincidence model. Using the noisy labels, along with attention between video and audio features, AudioScope learns to identify audio-visual similarity and to suppress off-screen sounds. We demonstrate the effectiveness of our approach using a dataset of video clips extracted from open-domain YFCC100m video data. This dataset contains a wide diversity of sound classes recorded in unconstrained conditions, making the application of previous methods unsuitable. For evaluation and semi-supervised experiments, we collected human labels for presence of on-screen and off-screen sounds on a small subset of clips.
View details
Preview abstract
Deep learning approaches have recently achieved impressive performance on both audio source separation and sound classification. Most audio source separation approaches focus only on separating sources belonging to a restricted domain of source classes, such as speech and music. However, recent work has demonstrated the possibility of "universal sound separation", which aims to separate acoustic sources from an open domain, regardless of their class. In this paper, we utilize the semantic information learned by sound classifier networks trained on a vast amount of diverse sounds to improve universal sound separation. In particular, we show that semantic embeddings extracted from a sound classifier can be used to condition a separation network, providing it with useful additional information. This approach is especially useful in an iterative setup, where source estimates from an initial separation stage and their corresponding classifier-derived embeddings are fed to a second separation network. By performing a thorough hyperparameter search consisting of over a thousand experiments, we find that classifier embeddings from oracle clean sources provide nearly one dB of SNR gain, and our best iterative models achieve a significant fraction of this oracle performance, establishing a new state-of-the-art for universal sound separation.
View details
Preview abstract
In recent years, rapid progress has been made on the problem of single-channel sound separation using supervised training of deep neural networks. In such supervised approaches, a model is trained to predict the component sources from synthetic mixtures created by adding up isolated ground-truth sources. Reliance on this synthetic training data is problematic because good performance depends upon the degree of match between the training data and real-world audio, especially in terms of the acoustic conditions and distribution of sources. The acoustic properties can be challenging to accurately simulate, and the distribution of sound types may be hard to replicate. In this paper, we propose a completely unsupervised method, mixture invariant training (MixIT), that requires only single-channel acoustic mixtures. In MixIT, training examples are constructed by mixing together existing mixtures, and the model separates them into a variable number of latent sources, such that the separated sources can be remixed to approximate the original mixtures. We show that MixIT can achieve competitive performance compared to supervised methods on speech separation. Using MixIT in a semi-supervised learning setting enables unsupervised domain adaptation and learning from large amounts of real world data without ground-truth source waveforms. In particular, we significantly improve reverberant speech separation performance by incorporating reverberant mixtures, train a speech enhancement system from noisy mixtures, and improve universal sound separation by incorporating a large amount of in-the-wild data.
View details
Unsupervised Speech Separation Using Mixtures of Mixtures
ICML 2020 Workshop on Self-Supervision for Audio and Speech
Preview abstract
Supervised approaches to single-channel speech separation rely on synthetic mixtures, so that the individual sources can be used as targets. Good performance depends upon how well the synthetic mixture data match real mixtures. However, matching synthetic data to the acoustic properties and distribution of sounds in a target domain can be challenging. Instead, we propose an unsupervised method that requires only singlechannel acoustic mixtures, without ground-truth source signals. In this method, existing mixtures are mixed together to form a mixture of mixtures, which the model separates into latent sources. We propose a novel loss that allows the latent sources
to be remixed to approximate the original mixtures. Experiments show that this method can achieve competitive performance on speech separation compared to supervised methods. In a semisupervised learning setting, our method enables domain adaptation by incorporating unsupervised mixtures from a matched domain. In particular, we demonstrate that significant improvement to reverberant speech separation performance can be achieved by incorporating reverberant mixtures.
View details