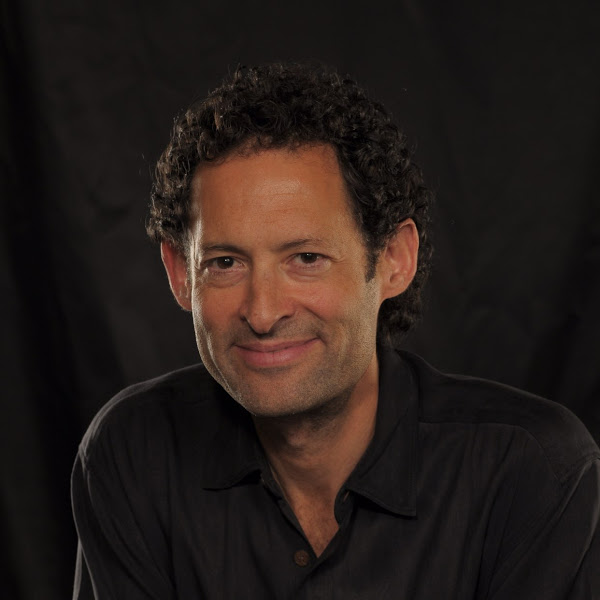
David H. Salesin
David Salesin leads the Capture, Creation & Interfaces team, whose mission is to Imagine and build the future of photography & videography, the creation of digital media, and the interfaces between humans & machines. David is also an Affiliate Professor in the Department of Computer Science & Engineering at the University of Washington, where he has been on the faculty since 1992. Prior to Google, he was Director of Research for Snap (2017-19), led the Creative Technologies Lab for Adobe Research as VP & Fellow (2005-17), and worked as a Senior Researcher at Microsoft Research (1999-2005). Earlier, he worked at Lucasfilm and Pixar, contributing computer animation to Academy Award-winning shorts and feature-length films (1983-87). He received his Sc.B. from Brown University in 1983, and his Ph.D. from Stanford University in 1991.
Authored Publications
Sort By
3D Moments from Near Duplicate Photos
Qianqian Wang
Zhengqi Li
Conference on Computer Vision and Pattern Recognition (CVPR) (2022)
Preview abstract
We introduce a new computational photography effect, starting from a pair of near duplicate photos that are prevalent in people's photostreams. Combining monocular depth synthesis and optical flow, we build a novel end-to-end system that can interpolate scene motion while simultaneously allowing independent control of the camera. We use our system to create short videos with scene motion and cinematic camera motion. We compare our method against two different baselines and demonstrate that our system outperforms them both qualitatively and quantitatively in publicly available benchmark datasets.
View details
Automatic Generation of Two-Level Hierarchical Tutorials from Instructional Makeup Videos
Anh Truong
Maneesh Agrawala
CHI 2021: ACM Conference on Human Factors in Computing Systems (2021)
Preview abstract
We present a multi-modal approach for automatically generating hierarchical tutorials from instructional makeup videos. Our approach is inspired by prior research in cognitive psychology, which suggests that people mentally segment procedural tasks into event hierarchies, where coarse-grained events focus on objects while fine-grained events focus on actions. In the instructional makeup domain, we find that objects correspond to facial parts while fine-grained steps correspond to actions on those facial parts. Given an input instructional makeup video, we apply a set of heuristics that combine computer vision techniques with transcript text analysis to automatically identify the fine-level action steps and group these steps by facial part to form the coarse-level events. We provide a voice-enabled, mixed-media UI to visualize the resulting hierarchy and allow users to efficiently navigate the tutorial (e.g., skip ahead, return to previous steps) at their own pace. Users can navigate the hierarchy at both the facial-part and action-step levels using click-based interactions and voice commands. We demonstrate the effectiveness of segmentation algorithms and the resulting mixed-media UI on a variety of input makeup videos. A user study shows that users prefer following instructional makeup videos in our mixed-media format to the standard video UI and that they find our format much easier to navigate.
View details
SLIDE: Single Image 3D Photography with Soft Layering and Depth-aware Inpainting
Varun Jampani*
Huiwen Chang*
Kyle Gregory Sargent
Abhishek Kar
Mike Krainin
Dominik Philemon Kaeser
Ce Liu
ICCV 2021 (2021)
Preview abstract
Single image 3D photography enables viewers to view a still image from novel viewpoints. Recent approaches for single-image view synthesis combine monocular depth network along with inpainting networks resulting in compelling novel view synthesis results. A drawback of these approaches is the use of hard layering making them not suitable to model intricate appearance effects such as matting. We present SLIDE, a modular and unified system for single image 3D photography that uses simple yet effective soft layering strategy to model appearance effects. In addition, we propose a novel depth-aware training of inpainting network suitable for 3D photography task. Extensive experimental analysis on 3 different view synthesis datasets in combination with user studies on in-the-wild image collections demonstrate the superior performance of our technique in comparison to existing strong baselines.
View details
Layered Neural Rendering for Retiming People in Video
Erika Lu
Weidi Xie
Andrew Zisserman
ACM Transactions on Graphics (Proc. SIGGRAPH Asia) (2020)
Preview abstract
We present a method for retiming people in an ordinary, natural video---manipulating and editing the time in which different motions of individuals in the video occur. We can temporally align different motions, change the speed of certain actions (speeding up/slowing down, or entirely "freezing" people), or "erase" selected people from the video altogether. We achieve these effects computationally via a dedicated learning-based layered video representation, where each frame in the video is decomposed into separate RGBA layers, representing the appearance of different people in the video. A key property of our model is that it not only disentangles the direct motions of each person in the input video, but also correlates each person automatically with the scene changes they generate---e.g., shadows, reflections, and motion of loose clothing. The layers can be individually retimed and recombined into a new video, allowing us to achieve realistic, high-quality renderings of retiming effects for real-world videos depicting complex actions and involving multiple individuals, including dancing, trampoline jumping, or group running.
View details
Monster Mash: A Single-View Approach to Casual 3D Modeling and Animation
Marek Dvoroznak
Olga Sorkine-Hornung
ACM Transactions on Graphics (TOG), ACM, New York, NY, USA (2020), pp. 1-12 (to appear)
Preview abstract
We present a new framework for sketch-based modeling and animation of 3D organic shapes that can work entirely in an intuitive 2D domain, enabling a playful, casual experience. Unlike previous sketch-based tools, our approach does not require a tedious part-based multi-view workflow with the explicit specification of an animation rig. Instead, we combine 3D inflation with a novel rigidity-preserving, layered deformation model, ARAP-L, to produce a smooth 3D mesh that is immediately ready for animation. Moreover, the resulting model can be animated from a single viewpoint — and without the need to handle unwanted inter-penetrations, as required by previous approaches. We demonstrate the benefit of our approach on a variety of examples produced by inexperienced users as well as professional animators. For less experienced users, our single-view approach offers a simpler modeling and animating experience than working in a 3D environment, while for professionals, it offers a quick and casual workspace for ideation.
View details