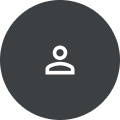
Ashish V. Thapliyal
I am a Software Engineer at Google Research, Mountain View, working in Radu Soricut's group. My current research interests are in Multilingual and Multimodal Machine Learning exploring learning from image, video, and text across a wide variety of languages/cultures. My previous research interests include Computer Security/Intrusion Detection and Quantum Information/Computation. I completed a PhD in Physics from the University of California at Santa Barbara for research in Quantum Information and Computation under the guidance of Dr. Charles H. Bennett (IBM Research) and Prof. David Awschalom. Concurrently with the PhD in Physics, I completed a MS in Computer Science (Systems) from the University of California at Santa Barbara.
Authored Publications
Sort By
MaXM: Towards Multilingual Visual Question Answering
Linting Xue
Michal Yarom
Findings of ACL: EMNLP (2023)
Preview abstract
Visual Question Answering (VQA) has been primarily studied through the lens of the English language. Yet, tackling VQA in other languages in the same manner would require a considerable amount of resources. In this paper, we propose scalable solutions to multilingual visual question answering (mVQA), on both data and modeling fronts. We first propose a translation-based framework to mVQA data generation that requires much less human annotation efforts than the conventional approach of directly collection questions and answers. Then, we apply our framework to the multilingual captions in the Crossmodal-3600 dataset and develop an efficient annotation protocol to create MaXM, a test-only VQA benchmark in 7 diverse languages. Finally, we develop a simple, lightweight, and effective approach as well as benchmark state-of-the-art English and multilingual VQA models. We hope that our benchmark encourages further research on mVQA.
View details
PaLI: A Jointly-Scaled Multilingual Language-Image Model
Piotr Padlewski
Daniel Salz
Sebastian Alexander Goodman
Basil Mustafa
Lucas Beyer
Alexander Kolesnikov
Keran Rong
Hassan Akbari
Linting Xue
James Bradbury
Chao Jia
Carlos Riquelme
Xiaohua Zhai
Neil Houlsby
International Conference on Learning Representations (ICLR) (2023)
Preview abstract
Effective scaling and a flexible task interface enable large-capacity language models to excel at many tasks. PaLI (Pathways Language and Image model) extends these ideas to the joint modeling of language and vision. PaLI is a model that generates text based on visual and textual inputs. Using this API, PaLI is able to perform many vision, language, and multimodal tasks, across many languages. We train PaLI with two main principles: reuse of pretrained unimodal components, and joint scaling of modalities. Using large-capacity pretrained language models and vision models allows us to capitalize on their existing capabilities, while leveraging the substantial cost of training them. We scale PaLI models across three axes:the language component, the vision component, and the training data that fuses them. For the vision component, we train the largest and best-performing VisionTransformer (ViT) to date. For the data, we build an image-text training set over10B images and covering over 100 languages.
PaLI inherits and enhances language-understanding capabilities, and achieves state-of-the-art in multiple vision and language tasks (image classification, image captioning, visual question-answering, scene-text understanding, etc.), based on a simple, modular, and reuse-friendly platform for modeling and scaling.
View details
Preview abstract
Research in massively multilingual image captioning has been severely hampered by a lack of
high-quality evaluation datasets. In this paper we present the Crossmodal-3600 dataset (XM3600 in short), a geographically-diverse set of 3600 images annotated with human-generated reference captions in 36 languages. The images were selected from across the world, covering regions where the 36 languages are spoken, and annotated with captions that achieve consistency in terms of style across all languages, while avoiding annotation artifacts due to direct translation. We apply this benchmark to model selection for massively multilingual image captioning models, and show strong correlation results with human evaluations when using XM3600 as golden references for automatic metrics.
View details