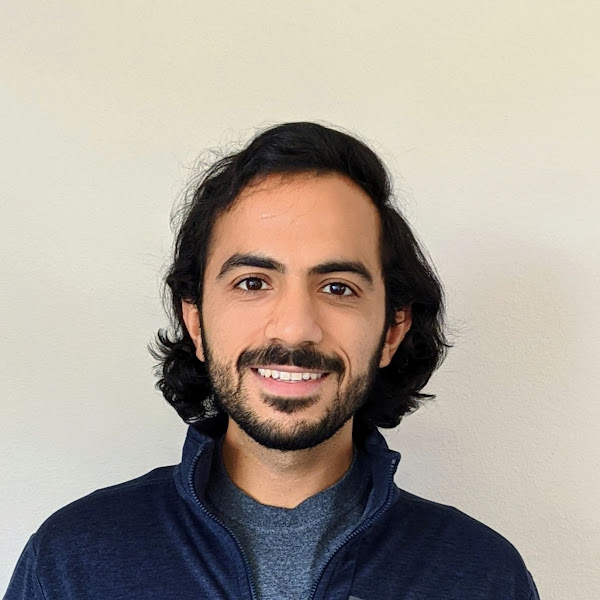
Nikhil Khani
Nikhil Khani is a seasoned technologist with a demonstrated history of leading impactful Machine Learning initiatives within the tech industry. Currently serving as a Staff Software Engineer at Google, Nikhil has landed critical projects related improving video recommendation quality at YouTube. He's the lead author on multiple patents and his expertise extends beyond recommendations. In his previous role at VMware (now Broadcom) Nikhil worked on improving cloud infrastructure using graphical neural networks as a Senior Machine Learning Engineer.
In addition to his professional endeavors, Nikhil actively contributes to the broader tech community. He serves as a program chair and peer reviewer for prestigious AI and ML conferences, shaping the landscape of technological discourse. His commitment to excellence and innovation has garnered him numerous accolades, including the Code Excellence Awards and multiple other awards for high quality improvements to YouTube Recommendations.
In addition to his professional endeavors, Nikhil actively contributes to the broader tech community. He serves as a program chair and peer reviewer for prestigious AI and ML conferences, shaping the landscape of technological discourse. His commitment to excellence and innovation has garnered him numerous accolades, including the Code Excellence Awards and multiple other awards for high quality improvements to YouTube Recommendations.
Authored Publications
Sort By
Bridging the Gap: Unpacking the Hidden Challenges in Knowledge Distillation for Online Ranking Systems
Shuo Yang
Aniruddh Nath
Yang Liu
Li Wei
Shawn Andrews
Maciej Kula
Jarrod Kahn
Zhe Zhao
Lichan Hong
2024
Preview abstract
Knowledge Distillation (KD) is a powerful approach for compressing large models into smaller, more efficient models, particularly beneficial for latency-sensitive applications like recommender systems. However, current KD research predominantly focuses on Computer Vision (CV) and NLP tasks, overlooking unique data characteristics and challenges inherent to recommender systems. This paper addresses these overlooked challenges, specifically: (1) mitigating data distribution shifts between teacher and student models, (2) efficiently identifying optimal teacher configurations within time and budgetary constraints, and (3) enabling computationally efficient and rapid sharing of teacher labels to support multiple students. We present a robust KD system developed and rigorously evaluated on multiple large-scale personalized video recommendation systems within Google. Our live experiment results demonstrate significant improvements in student model performance while ensuring the consistent and reliable generation of high-quality teacher labels from continuous data streams.
View details