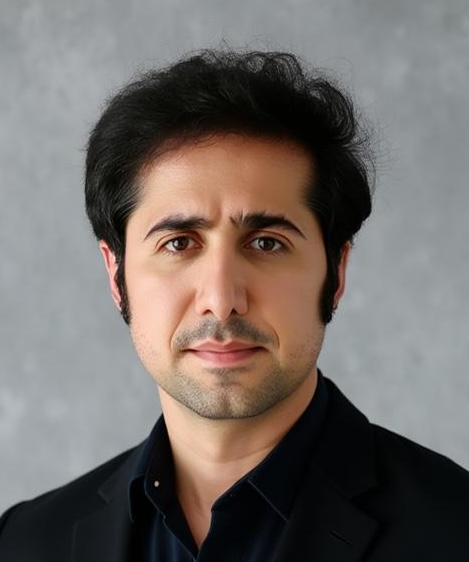
S. Mostafa Mousavi
S. Mostafa Mousavi is a research scientist whose work focuses on the scientific applications of artificial intelligence, particularly in observational seismology. His interests lie at the intersection of geophysics, statistics, and computer science. His research includes machine learning, signal processing, and pattern recognition in extensive sensor data to address challenging problems related to natural hazards and their impacts.
Research Areas
Authored Publications
Sort By
Gemini & Physical World: Large Language Models Can Estimate the Intensity of Earthquake Shaking from Multi-Modal Social Media Posts
Marc Stogaitis
Tajinder Gadh
Richard Allen
Alexei Barski
Robert Bosch
Patrick Robertson
Youngmin Cho
Nivetha Thiruverahan
Aman Raj
Geophysical Journal International (2025), ggae436
Preview abstract
This paper presents a novel approach for estimating the ground shaking intensity using real-time social media data and CCTV footage. Employing the Gemini 1.5 Pro’s (Reid et al. 2024) model, a multi-modal language model, we demonstrate the ability to extract relevant information from unstructured data utilizing generative AI and natural language processing. The model’s output, in the form of Modified Mercalli Intensity (MMI) values, align well with independent observational data. Furthermore, our results suggest that beyond its advanced visual and auditory understanding abilities, Gemini appears to utilize additional sources of knowledge, including a simplified understanding of the general relationship between earthquake magnitude, distance, and MMI intensity, which it presumably acquired during its training, in its reasoning and decision-making processes. These findings raise intriguing questions about the extent of Gemini's general understanding of the physical world and its phenomena. Gemini’s ability to generate results consistent with established scientific knowledge highlights the potential of LLMs like Gemini in augmenting our understanding of complex physical phenomena such as earthquakes. More specifically, the results of this study highlight the potential of LLMs like Gemini to revolutionize citizen seismology by enabling rapid, effective, and flexible analysis of crowdsourced data from eyewitness accounts for assessing earthquake impact and providing crisis situational awareness. This approach holds a great promise for improving early warning systems, disaster response, and overall resilience in earthquake-prone regions. This study provides a significant step toward harnessing the power of social media and AI for earthquake disaster mitigation.
View details
Machine Learning in Earthquake Seismology
Annual Review of Earth and Planetary Sciences , 51 (2023), pp. 105-129
Preview abstract
Machine learning (ML) is a collection of methods used to develop understanding and predictive capability by learning relationships embedded in data. ML methods are becoming the dominant approaches for many tasks in seismology. ML and data mining techniques can significantly improve our capability for seismic data processing. In this review we provide a comprehensive overview of ML applications in earthquake seismology, discuss progress and challenges, and offer suggestions for future work.
▪ Conceptual, algorithmic, and computational advances have enabled rapid progress in the development of machine learning approaches to earthquake seismology.
▪ The impact of that progress is most clearly evident in earthquake monitoring and is leading to a new generation of much more comprehensive earthquake catalogs.
▪ Application of unsupervised approaches for exploratory analysis of these high-dimensional catalogs may reveal new understanding of seismicity.
▪ Machine learning methods are proving to be effective across a broad range of other seismological tasks, but systematic benchmarking through open source frameworks and benchmark data sets are important to ensure continuing progress.
View details
Deep Learning Seismology
Science, 377 (2022), eabm4470
Preview abstract
Seismology is the study of seismic waves to understand their origin—most obviously, sudden fault slip in earthquakes, but also explosions, volcanic eruptions, glaciers, landslides, ocean waves, vehicular traffic, aircraft, trains, wind, air guns, and thunderstorms, for example. Seismology uses those same waves to infer the structure and properties of planetary interiors. Because sources can generate waves at any time, seismic ground motion is recorded continuously, at typical sampling rates of 100 points per second, for three components of motion, and on arrays that can include thousands of sensors. Although seismology is clearly a data-rich science, it often is a data-driven science as well, with new phenomena and unexpected behavior discovered with regularity. And for at least some tasks, the careful and painstaking work of seismic analysts over decades and around the world has also made seismology a data label–rich science. This facet makes it fertile ground for deep learning, which has entered almost every subfield of seismology and outperforms classical approaches, often dramatically, for many seismological tasks.
View details