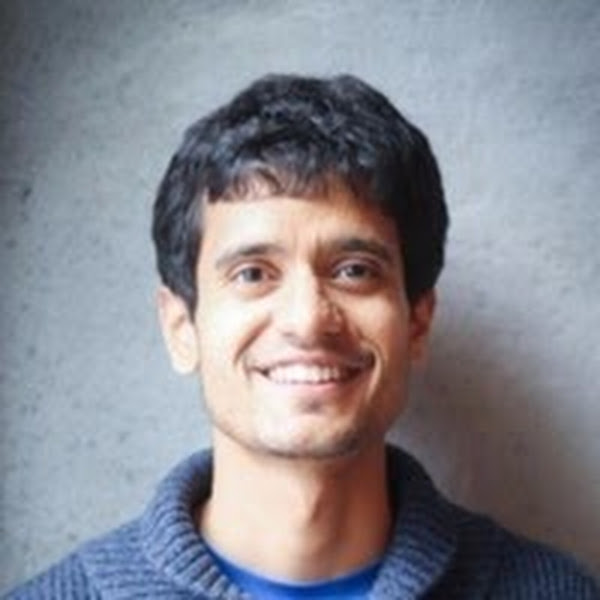
Achin (Ace) Kulshrestha
Ace is a Staff Software Engineer & Manager in Google's AR semantic perception team under Federico Tombari, focusing on privacy-aware ambient perception, On-Device LLMs, VLMs and 3D computer vision.
Beginning with a prestigious Microsoft award in 2009 to pursue privacy research, their contributions have extended to privacy, machine learning and anti-abuse roles at Facebook, UW-Madison, Yelp, and Google, spanning over 14+ years. They have a graduate degree in Computer Sciences from the University of Wisconsin-Madison.
Research Areas
Authored Publications
Sort By
Preview abstract
As large language models (LLMs) improve in their capacity to serve as personal AI assistants, their ability to output uniquely tailored, personalized responses that align with the soft preferences of their users is imperative for maximizing user satisfaction and retention. However, lay users are notoriously bad at prompt specification and often struggle with conveying their latent preferences to AI assistants. To resolve this, we demonstrate that activation steering, an inference-time method, can effectively control the response of the LLMs towards expressing different preferences. In contrast to memory-based personalization methods that require long user history, steering is extremely lightweight and easily-controllable via an interpretable linear strength factor. We further conduct a within-subjects user study (n=14) to investigate how end users personalize their conversations through three different steerable chatbot interfaces. The results demonstrate the effectiveness of preference-based steering for aligning real-world conversations with user preferences, and we discuss qualitative findings on how diverse values around control, transparency, and usability of personalization lead users to prefer different interfaces.
View details
Preview abstract
Understanding fine-grained temporal dynamics is crucial in egocentric videos, where continuous streams capture frequent, close-up interactions with objects. In this work, we bring to light that current egocentric video question-answering datasets often include questions that can be answered using only few frames or commonsense reasoning, without being necessarily grounded in the actual video. Our analysis shows that state-of-the-art Multi-Modal Large Language Models (MLLMs) on these benchmarks achieve remarkably high performance using just text or a single frame as input. To address these limitations, we introduce EgoTempo, a dataset specifically designed to evaluate temporal understanding in the egocentric domain. EgoTempo emphasizes tasks that require integrating information across the entire video, ensuring that models would need to rely on temporal patterns rather than static cues or pre-existing knowledge. Extensive experiments on EgoTempo show that current MLLMs still fall short in temporal reasoning on egocentric videos, and thus we hope EgoTempo will catalyze new research in the field and inspire models that better capture the complexity of temporal dynamics. Dataset and code are available at https://github.com/google-research-datasets/egotempo.git.
View details
I know what I don't know: improving model cascades through confidence tuning
Stephan Rabanser
Nathalie Rauschmayr
Petra Poklukar
Congchao Wang
2025
Preview abstract
Large-scale machine learning models deliver strong performance across a wide range of tasks but come with significant computational and resource constraints. To mitigate these challenges, local smaller models are often deployed alongside larger models, relying on routing and deferral mechanisms to offload complex tasks. However, existing approaches inadequately balance the capabilities of these models, often resulting in unnecessary deferrals or sub-optimal resource usage. In this work we introduce a novel loss function called Gatekeeper for calibrating smaller models in cascade setups. Our approach fine-tunes the smaller model to confidently handle tasks it can perform correctly while deferring complex tasks to the larger model. Moreover, it incorporates a mechanism for managing the trade-off between model performance and deferral accuracy, and is broadly applicable across various tasks and domains without any architectural changes. We evaluated our method on encoder-only, decoder-only, and encoder-decoder architectures. Experiments across image classification, language modeling, and vision-language tasks show that our approach substantially improves deferral performance.
View details
Preview abstract
Vision-language models (VLMs) are typically composed of a vision encoder, e.g. CLIP, and a language model (LM) that interprets the encoded features to solve downstream tasks. Despite remarkable progress, VLMs are subject to several shortcomings due to the limited capabilities of vision encoders, e.g. "blindness" to certain image features, visual hallucination, etc. To address these issues, we study broadening the visual encoding capabilities of VLMs. We first comprehensively benchmark several vision encoders with different inductive biases for solving VLM tasks. We observe that there is no single encoding configuration that consistently achieves top performance across different tasks, and encoders with different biases can perform surprisingly similarly. Motivated by this, we introduce a method, named BRAVE, that consolidates features from multiple frozen encoders into a more versatile representation that can be directly fed as the input to a frozen LM. BRAVE achieves state-of-the-art performance on a broad range of captioning and VQA benchmarks and significantly reduces the aforementioned issues of VLMs, while requiring a smaller number of trainable parameters than existing methods and having a more compressed representation. Our results highlight the potential of incorporating different visual biases for a more broad and contextualized visual understanding of VLMs.
View details