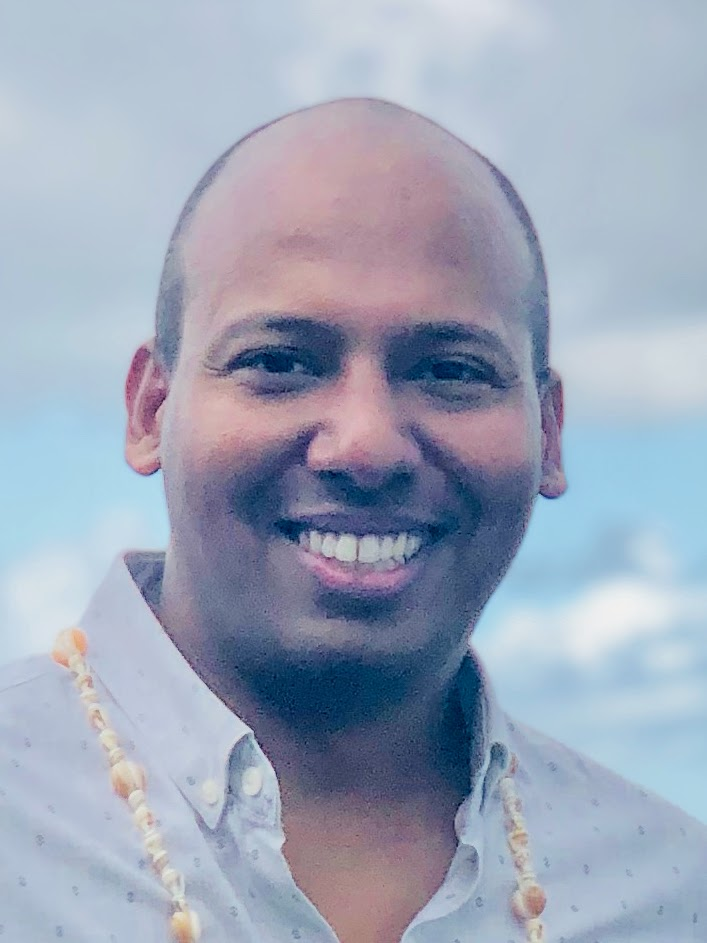
Animesh Srivastava
Animesh Srivastava is a member of Applied Privacy Research group at Google. He received his PhD in Computer Science from Duke University, USA in 2017 where he focused on building access mechanisms for mobile operating system to prevent inadvertent visual leaks and protect users' privacy. His wider research interests include applications of natural language processing and machine learning to privacy, modelling user behavior by leveraging large scale data to understand users' privacy and security concerns, and building usable privacy and security solutions.
Research Areas
Authored Publications
Sort By
A Decade of Privacy-Relevant Android App Reviews: Large Scale Trends
Omer Akgul
Michelle Mazurek
Benoit Seguin
Preview abstract
We present an analysis of 12 million instances of privacy-relevant reviews publicly visible on the Google Play Store that span a 10 year period. By leveraging state of the art NLP techniques, we examine what users have been writing about privacy along multiple dimensions: time, countries, app types, diverse privacy topics, and even across a spectrum of emotions. We find consistent growth of privacy-relevant reviews, and explore topics that are trending (such as Data Deletion and Data Theft), as well as those on the decline (such as privacy-relevant reviews on sensitive permissions). We find that although privacy reviews come from more than 200 countries, 33 countries provide 90% of privacy reviews. We conduct a comparison across countries by examining the distribution of privacy topics a country’s users write about, and find that geographic proximity is not a reliable indicator that nearby countries have similar privacy perspectives. We uncover some countries with unique patterns and explore those herein. Surprisingly, we uncover that it is not uncommon for reviews that discuss privacy to be positive (32%); many users express pleasure about privacy features within apps or privacy-focused apps. We also uncover some unexpected behaviors, such as the use of reviews to deliver privacy disclaimers to developers. Finally, we demonstrate the value of analyzing app reviews with our approach as a complement to existing methods for understanding users' perspectives about privacy.
View details
Unveiling Privacy Perspectives about Mobile Health Apps on a Large Scale
PETS workshop: Privacy, Safety and Trust for Mobile Health Apps (2024)
Preview abstract
In this paper we study users' opinions about the privacy of their mobile health apps. We look at what they write in app reviews in the 'Health & Fitness' category on the Google Play store. We identified 2832 apps in this category (based on 1K minimum installs). Using NLP/LLM analyses, we find that 76% of these apps have at least some privacy reviews. In total this yields over 164,000 reviews about privacy, from over 150 countries and in 25 languages. Our analyses identifies top themes and offers an approximation of how widespread these issues are around the world. We show that the top 4 themes - Data Sharing and Exposure, Permission Requests, Location Tracking and Data Collection - are issues of concern in over 70 countries. Our automatically generated thematic summaries reveal interesting aspects that deserve further research around user suspicions (unneeded data collection), user requests (more fine-grained control over data collection and data access), as well as user behavior (uninstalling apps).
View details
Preview abstract
In this paper we study users' opinions about the privacy of their mobile health apps. We look at what they write in app reviews in the 'Health & Fitness' category on the Google Play store. We identified 2832 apps in this category (based on 1K minimum installs). Using NLP/LLM analyses, we find that 76% of these apps have at least some privacy reviews. In total this yields over 164,000 reviews about privacy, from over 150 countries and in 25 languages. Our analyses identifies top themes and offers an approximation of how widespread these issues are around the world. We show that the top 4 themes - Data Sharing and Exposure, Permission Requests, Location Tracking and Data Collection - are issues of concern in over 70 countries. Our automatically generated thematic summaries reveal interesting aspects that deserve further research around user suspicions (unneeded data collection), user requests (more fine-grained control over data collection and data access), as well as user behavior (uninstalling apps).
View details
Preview abstract
In this paper we study users' opinions about the privacy of their mobile health apps. We look at what they write in app reviews in the 'Health & Fitness' category on the Google Play store. We identified 2832 apps in this category (based on 1K minimum installs). Using NLP/LLM analyses, we find that 76% of these apps have at least some privacy reviews. In total this yields over 164,000 reviews about privacy, from over 150 countries and in 25 languages. Our analyses identifies top themes and offers an approximation of how widespread these issues are around the world. We show that the top 4 themes - Data Sharing and Exposure, Permission Requests, Location Tracking and Data Collection - are issues of concern in over 70 countries. Our automatically generated thematic summaries reveal interesting aspects that deserve further research around user suspicions (unneeded data collection), user requests (more fine-grained control over data collection and data access), as well as user behavior (uninstalling apps).
View details
Hark: A Deep Learning System for Navigating Privacy Feedback at Scale
Rishabh Khandelwal
2022 IEEE Symposium on Security and Privacy (SP)
Preview abstract
Integrating user feedback is one of the pillars for building successful products. However, this feedback is generally collected in an unstructured free-text form, which is challenging to understand at scale. This is particularly demanding in the privacy domain due to the nuances associated with the concept and the limited existing solutions. In this work, we present Hark, a system for discovering and summarizing privacy-related feedback at scale. Hark automates the entire process of summarizing privacy feedback, starting from unstructured text and resulting in a hierarchy of high-level privacy themes and fine-grained issues within each theme, along with representative reviews for each issue. At the core of Hark is a set of new deep learning models trained on different tasks, such as privacy feedback classification, privacy issues generation, and high-level theme creation. We illustrate Hark’s efficacy on a corpus of 626M Google Play reviews. Out of this corpus, our privacy feedback classifier extracts 6M privacy-related reviews (with an AUC-ROC of 0.92). With three annotation studies, we show that Hark’s generated issues are of high accuracy and coverage and that the theme titles are of high quality. We illustrate Hark’s capabilities by presenting high-level insights from 1.3M Android apps.
View details