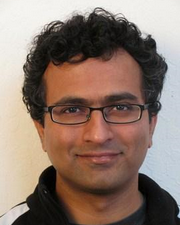
Hrishikesh Aradhye
Research Areas
Authored Publications
Sort By
Wide & Deep Learning for Recommender Systems
Levent Koc
Tal Shaked
Glen Anderson
Wei Chai
Mustafa Ispir
Rohan Anil
Zakaria Haque
Lichan Hong
Vihan Jain
Xiaobing Liu
Hemal Shah
arXiv:1606.07792 (2016)
Preview abstract
Generalized linear models with nonlinear feature transformations are widely used for large-scale regression and classification problems with sparse inputs. Memorization of feature interactions through a wide set of cross-product feature transformations are effective and interpretable, while generalization requires more feature engineering effort. With less feature engineering, deep neural networks can generalize better to unseen feature combinations through low-dimensional dense embeddings learned for the sparse features. However, deep neural networks with embeddings can over-generalize and recommend less relevant items when the user-item interactions are sparse and high-rank. In this paper, we present Wide & Deep learning---jointly trained wide linear models and deep neural networks---to combine the benefits of memorization and generalization for recommender systems. We productionized and evaluated the system on a commercial mobile app store with over one billion active users and over one million apps. Online experiment results show that Wide & Deep significantly increased app acquisitions compared with wide-only and deep-only models.
View details
Automatically Discovering Talented Musicians with Acoustic Analysis of YouTube Videos
Eric Nichols
Charles DuHadway
Proceedings of the 2012 IEEE 12th International Conference on Data Mining (ICDM), IEEE Computer Society, Washington, DC, USA, pp. 559-565
Preview abstract
Online video presents a great opportunity for up-and-coming singers and artists to be visible to a worldwide audience. However, the sheer quantity of video makes it difficult to discover promising musicians. We present a novel algorithm to automatically identify talented musicians using machine learning and acoustic analysis on a large set of "home singing" videos. We describe how candidate musician videos are identified and ranked by singing quality. To this end, we present new audio features specifically designed to directly capture singing quality. We evaluate these vis-a-vis a large set of generic audio features and demonstrate that the proposed features have good predictive performance. We also show that this algorithm performs well when videos are normalized for production quality.
View details
Boosting Video Classification Using Cross-Video Signals
Preview
Proceedings of the IEEE Conference on Acoustics, Speech, and Signal Processing (ICASSP) (2011) (to appear)
Preview abstract
This paper explores the problem of large-scale automatic video geolocation. A methodology is developed to infer the location at which videos from Anonymized.com were recorded using video content and various additional signals. Specifically, multiple binary Adaboost classifiers are trained to identify particular places based on learning decision stumps on sets of hundreds of thousands of sparse features. A one-vs-all classification strategy is then used to classify the location at which videos were recorded. Empirical validation is performed on an immense data set of 20 million labeled videos. Results demonstrate that high accuracy video geolocation is indeed possible for many videos and locations and interesting relationships exist between between videos and the places where they are recorded.
View details
Finding Meaning on YouTube: Tag Recommendation and Category Discovery
Marius Pasca
Computer Vision and Pattern Recognition, IEEE (2010)
Preview abstract
We present a system that automatically recommends tags for YouTube
videos solely based on their audiovisual content. We also propose a novel framework
for unsupervised discovery of video categories that exploits knowledge mined
from the World-Wide Web text documents/searches. First, video content to tag
association is learned by training classifiers that map audiovisual
content-based features from millions of videos on YouTube.com to existing
uploader-supplied tags for these videos. When a new video is uploaded, the
labels provided by these classifiers are used to automatically suggest tags
deemed relevant to the video. Our system has learned a vocabulary of over 20,000 tags.
Secondly, we mined large volumes of Web pages and search queries to discover a
set of possible text entity categories and a set of associated is-A
relationships that map individual text entities to categories. Finally, we
apply these is-A relationships mined from web text on the tags learned from
audiovisual content of videos to automatically synthesize a reliable set of
categories most relevant to videos -- along with a mechanism to predict these
categories for new uploads. We then present rigorous rating studies that
establish that: (a) the average relevance of tags automatically recommended by
our system matches the average relevance of the uploader-supplied tags at the
same or better coverage and (b) the average precision@K of video categories
discovered by our system is 70% with K=5.
View details
Preview abstract
This paper discusses a new method for automatic
discovery and organization of descriptive concepts (labels)
within large real-world corpora of user-uploaded multimedia,
such as YouTube.com. Conversely, it also provides validation
of existing labels, if any. While training, our method does not
assume any explicit manual annotation other than the weak
labels already available in the form of video title, descrip-
tion, and tags. Prior work related to such auto-annotation
assumed that a vocabulary of labels of interest (e.g., indoor,
outdoor, city, landscape) is specified a priori. In contrast,
the proposed method begins with an empty vocabulary. It
analyzes audiovisual features of 25 million YouTube.com videos
– nearly 150 years of video data – effectively searching for
consistent correlation between these features and text metadata.
It autonomously extends the label vocabulary as and when it
discovers concepts it can reliably identify, eventually leading
to a vocabulary with thousands of labels and growing. We
believe that this work significantly extends the state of the art
in multimedia data mining, discovery, and organization based
on the technical merit of the proposed ideas as well as the
enormous scale of the mining exercise in a very challenging,
unconstrained, noisy domain.
View details
Audiovisual Celebrity Recognition in Unconstrained Web Videos
Preview
Pedro Moreno
Proceedings of the IEEE Conference on Acoustics, Speech, and Signal Processing (ICASSP) (2009)
Adaptive, selective, automatic tonal enhancement of faces
Preview
ACM Multimedia, ACM, New York, NY, USA (2009), pp. 677-680