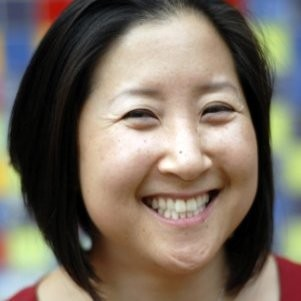
Beverly Freeman
https://www.linkedin.com/in/beverlyfreeman/
Research Areas
Authored Publications
Sort By
Zoom in, Zoom out, Reframe: Domain Experts’ Strategies for Addressing Non-Experts’ Complex Questions
Roma Ruparel
Proceedings of the 2025 CHI Conference on Human Factors in Computing Systems (CHI) (2025)
Preview abstract
Consumers rely on the Internet for expert information in domains such as healthcare and law. Large Language Models (LLMs) have the potential to increase access to expert knowledge. However, past research has not addressed how to handle certain aspects of complex questions that commonly occur in expert-layperson interactions. We conducted in-depth interviews with 26 experts across multiple domains to understand how they experience and respond to challenges associated with non-experts’ questions. Results from a thematic analysis reveal three recurring strategies that experts across domains employ when fielding complex questions. Experts zoom in to clarify details of a broad information request, zoom out to address overly narrow questions or assumptions, and reframe when the underlying need is unstated or poorly represented. We discuss implications for the design of LLM-based experiences that facilitate access to expert information.
View details
Iterative quality control strategies for expert medical image labeling
Sonia Phene
Abigail Huang
Rebecca Ackermann
Olga Kanzheleva
Caitlin Taggart
Proceedings of the AAAI Conference on Human Computation and Crowdsourcing (2021)
Preview abstract
Data quality is a key concern for artificial intelligence (AI) efforts that rely upon crowdsourced data collection. In the domain of medicine in particular, labeled data must meet higher quality standards, or the resulting AI may lead to patient harm, and/or perpetuate biases. What are the challenges involved in expert medical labeling? What processes do such teams employ? In this study, we interviewed members of teams developing AI for medical imaging across 4 subdomains (ophthalmology, radiology, pathology, and dermatology). We identify a set of common practices for ensuring data quality. We describe one instance of low-quality labeling caught by post-launch monitoring. However, the more common pattern is to involve experts in an iterative process of defining, testing, and iterating tasks and instructions. Teams invest in these upstream efforts in order to mitigate downstream quality issues during large-scale labeling.
View details