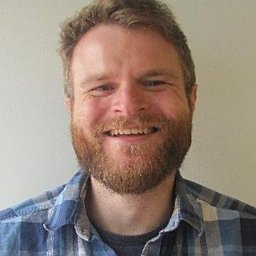
James Atwood
Research Areas
Authored Publications
Sort By
Towards A Scalable Solution for Improving Multi-Group Fairness in Compositional Classification
Tina Tian
Ben Packer
Meghana Deodhar
Alex Beutel
Ahmad Beirami
The Second Workshop on Spurious Correlations, Invariance and Stability @ ICML 2023 (2023)
Preview abstract
Despite the rich literature on machine learning fairness, relatively little attention has been paid to remediating complex systems, where the final prediction is the combination of multiple classifiers and where multiple groups are present. In this paper, we first show that natural baseline approaches for improving equal opportunity fairness scale linearly with the product of the number of remediated groups and the number of remediated prediction labels, rendering them impractical. We then introduce two simple techniques, called task-overconditioning and group-interleaving, to achieve a constant scaling in this multi-group multi-label setup. Our experimental results in academic and real-world environments demonstrate the effectiveness of our proposal at mitigation within this environment.
View details
The Inclusive Images Competition
D. Sculley
Igor Ivanov
Miha Skalic
Pallavi Baljekar
Pavel Ostyakov
Roman Solovyev
Weimin Wang
Yoni Halpern
Springer Series (2019)
Preview abstract
Popular large image classification datasets that are drawn from the web present Eurocentric and Americentric biases that negatively impact the generalizability of models trained on them . In order to encourage the development of modeling approaches that generalize well to images drawn from locations and cultural contexts that are unseen or poorly represented at the time of training, we organized the Inclusive Images competition in association with Kaggle and the NeurIPS 2018 Competition Track Workshop. In this chapter, we describe the motivation and design of the competition, present reports from the top three competitors, and provide high-level takeaways from the competition results.
View details
BriarPatches: Pixel-Space Interventions for Inducing Demographic Parity
Yoni Halpern
D. Sculley
Neural Information Processing Systems: Workshop on Ethical, Social and Governance Issues in AI (2018)
Preview abstract
We introduce the BriarPatch, a pixel-space intervention that obscures sensitive attributes from representations encoded in pre-trained classifiers. The patches encourage internal model representations not to encode sensitive information, which has the effect of pushing downstream predictors towards exhibiting demographic parity with respect to the sensitive information. The net result is that these BriarPatches provide an intervention mechanism available at user level, and complements prior research on fair representations that were previously only applicable by model developers and ML experts.
View details
No Classification without Representation: Assessing Geodiversity Issues in Open Data Sets for the Developing World
Shreya Shankar
Yoni Halpern
D. Sculley
NIPS 2017 workshop: Machine Learning for the Developing World
Preview abstract
Modern machine learning systems such as image classifers rely heavily on
large scale data sets for training. Such data sets are costly to create,
thus in practice a small number of freely available, open source data sets
are widely used. Such strategies may be particularly important for ML
applications in the developing world, where resources may be constrained
and the cost of creating suitable large scale data sets may be a
blocking factor. However, we suggest that examining the {\em geo-diversity}
of open data sets is critical before adopting a data set for such use
cases. In particular, we analyze two large, publicly available image
data sets to assess geo-diversity and find that these data sets appear
to exhibit a observable amerocentric and eurocentric representation bias.
Further, we perform targeted analysis on classifiers that use these data
sets as training data to assess the impact of these training distributions,
and find strong differences in the relative performance on images from
different locales. These results emphasize the need to ensure
geo-representation when constructing data sets for use in the developing
world.
View details