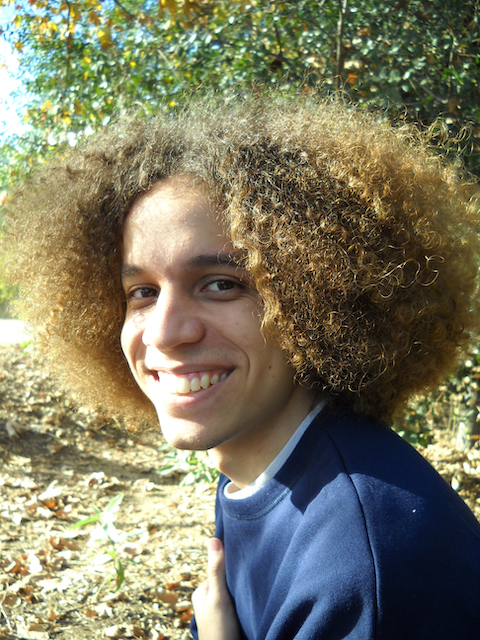
Jonas Kemp
Jonas is a research engineer in Google Health. He joined Google as an AI resident in 2017, investigating deep learning methods for modeling and understanding multimodal data in electronic health records. His research interests center on improving the quality, actionability, and reliability of clinical risk predictions, with a particular focus on natural language processing and representation learning methods. Jonas earned his BA in Human Biology and his MS in Computer Science from Stanford University.
Research Areas
Authored Publications
Sort By
Deciphering clinical abbreviations with a privacy protecting machine learning system
Alvin Rajkomar
Eric Loreaux
Yuchen Liu
Benny Li
Ming-Jun Chen
Yi Zhang
Afroz Mohiuddin
Juraj Gottweis
Nature Communications (2022)
Preview abstract
Physicians write clinical notes with abbreviations and shorthand that are difficult to decipher. Abbreviations can be clinical jargon (writing “HIT” for “heparin induced thrombocytopenia”), ambiguous terms that require expertise to disambiguate (using “MS” for “multiple sclerosis” or “mental status”), or domain-specific vernacular (“cb” for “complicated by”). Here we train machine learning models on public web data to decode such text by replacing abbreviations with their meanings. We report a single translation model that simultaneously detects and expands thousands of abbreviations in real clinical notes with accuracies ranging from 92.1%-97.1% on multiple external test datasets. The model equals or exceeds the performance of board-certified physicians (97.6% vs 88.7% total accuracy). Our results demonstrate a general method to contextually decipher abbreviations and shorthand that is built without any privacy-compromising data.
View details
Instability in clinical risk prediction models using deep learning
Daniel Lopez-Martinez
Alex Yakubovich
Martin Seneviratne
Akshit Tyagi
Ethan Steinberg
N. Lance Downing
Ron C. Li
Keith E. Morse
Nigam H. Shah
Ming-Jun Chen
Proceedings of the 2nd Machine Learning for Health symposium, PMLR (2022), pp. 552-565
Preview abstract
While it has been well known in the ML community that deep learning models suffer from instability, the consequences for healthcare deployments are under-characterised. We study the stability of different model architectures trained on electronic health records, using a set of outpatient prediction tasks as a case study.
We show that repeated training runs of the same deep learning model on the same training data can result in significantly different outcomes at a patient level even though global performance metrics remain stable.
We propose two stability metrics for measuring the effect of randomness of model training, as well as mitigation strategies for improving model stability.
View details
User-centred design for machine learning in health care: a case study from care management
Birju Patel
Daniel Lopez-martinez
Doris Wong
Eric Loreaux
Janjri Desai
Jonathan Chen
Lance Downing
Lutz Thomas Finger
Martin Gamunu Seneviratne
Ming-Jun Chen
Nigam Shah
Ron Li
BMJ Health & Care Informatics (2022)
Preview abstract
Objectives: Few machine learning (ML) models are successfully deployed in clinical practice. One of the common pitfalls across the field is inappropriate problem formulation: designing ML to fit the data rather than to address a real-world clinical pain point.
Methods: We introduce a practical toolkit for user-centred design consisting of four questions covering: (1) solvable pain points, (2) the unique value of ML (eg, automation and augmentation), (3) the actionability pathway and (4) the model’s reward function. This toolkit was implemented in a series of six participatory design workshops with care managers in an academic medical centre.
Results: Pain points amenable to ML solutions included outpatient risk stratification and risk factor identification. The endpoint definitions, triggering frequency and evaluation metrics of the proposed risk scoring model were directly influenced by care manager workflows and real-world constraints.
Conclusions: Integrating user-centred design early in the ML life cycle is key for configuring models in a clinically actionable way. This toolkit can guide problem selection and influence choices about the technical setup of the ML problem.
View details
Analyzing the Role of Model Uncertainty for Electronic Health Records
Edward Choi
Jeremy Nixon
Ghassen Jerfel
ACM Conference on Health, Inference, and Learning (ACM CHIL) (2020)
Preview abstract
In medicine, both ethical and monetary costs of incorrect predictions can be significant, and the complexity of the problems often necessitates increasingly complex models. Recent work has shown that changing just the random seed is enough for otherwise well-tuned deep neural networks to vary in their individual predicted probabilities. In light of this, we investigate the role of model uncertainty methods in the medical domain. Using RNN ensembles and various Bayesian RNNs, we show that population-level metrics, such as AUC-PR, AUC-ROC, log-likelihood, and calibration error, do not capture model uncertainty. Meanwhile, the presence of significant variability in patient-specific predictions and optimal decisions motivates the need for capturing model uncertainty. Understanding the uncertainty for individual patients is an area with clear clinical impact, such as determining when a model decision is likely to be brittle. We further show that RNNs with only Bayesian embeddings can be a more efficient way to capture model uncertainty compared to ensembles, and we analyze how model uncertainty is impacted across individual input features and patient subgroups.
View details
Preview abstract
Clinical notes in electronic health records contain highly heterogeneous writing styles, including non-standard terminology or abbreviations. Using these notes in predictive modeling has traditionally required preprocessing (e.g. taking frequent terms or topic modeling) that removes much of the richness of the source data. We propose a pretrained hierarchical recurrent neural network model that parses minimally processed clinical notes in an intuitive fashion, and show that it improves performance for discharge diagnosis classification tasks on the Medical Information Mart for Intensive Care III (MIMIC-III) dataset, compared to models that treat the notes as an unordered collection of terms or that conduct no pretraining. We also apply an attribution technique to examples to identify the words that the model uses to make its prediction, and show the importance of the words' nearby context.
View details