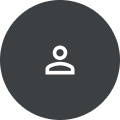
Konstantin Voevodski
Authored Publications
Sort By
Large Scale K-Clustering
ACM Transactions on Knowledge Discovery from Data (2024)
Preview abstract
Large-scale learning algorithms are essential for modern data collections that may have billions of data points. Here we study the design of parallel $k$-clustering algorithms, which include the $k$-median, $k$-medoids, and $k$-means clustering problems. We design efficient parallel algorithms for these problems and prove that they still compute constant-factor approximations to the optimal solution for stable clustering instances. In addition to our theoretic results we present computational experiments that show that our $k$-median and $k$-means algorithms work well in practice - we are able to find better clusterings than state-of-the-art coreset constructions using samples of the same size.
View details
Large Scale K-Median Clustering for Stable Clustering Instances
AISTATS (2021), pp. 2890-2898
Preview abstract
We study the problem of computing a good k-median clustering in a parallel computing environment. We design an efficient algorithm that gives a constant-factor approximation to the optimal solution for stable clustering instances. The notion of stability that we consider is resilience to perturbations of the distances between the points. Our computational experiments show that our algorithm works well in practice - we are able to find better clusterings than Lloyd’s algorithm and a centralized coreset construction using samples of the same size.
View details
Semi-Supervised Max-Sum Clustering
CIKM (2020), pp. 1495-1504
Preview abstract
We study max-sum clustering in a semi-supervised setting. Our objective function maximizes the pairwise within-cluster similarity with respect to some null hypothesis regarding the similarity. This is a natural objective that does not require any additional parameters, and is a generalization of the well-known modularity objective function. We show that for such an objective function in a semi-supervised setting we can compute an additive approximation of the optimal solution in the general case, and a constant-factor approximation when the optimal objective value is large. The supervision that we consider is in the form of cluster assignment queries and same-cluster queries; we also study the setting where the query responses are noisy. Our algorithm also generalizes to the min-sum objective function, for which we can achieve similar performance guarantees. We present experiments to show that our framework is effective for clustering text data - we are able to find clusterings that are close to the queried clustering and have a good objective value.
View details
Local Algorithms for Interactive Clustering
Pranjal Awasthi
Maria Florina Balcan
Journal of Machine Learning Research, 18 (2017), pp. 1-35
Preview abstract
We study the design of interactive semi-supervised clustering algorithms. The user supervision that we consider is in the form of cluster split/merge requests; such feedback is easy for users to provide because it only requires a high-level understanding of the clusters. Our algorithms start with any initial clustering and only make local changes in each step; both are desirable properties in many applications. Local changes are desirable because in practice edits of other parts of the clustering are considered churn - changes that are perceived as quality-neutral or quality-negative. We show that in this framework we can still design provably correct algorithms given that our data satisfies natural separability properties. We also show that our framework works well in practice.
View details
Monotonic Calibrated Interpolated Look-Up Tables
Maya Gupta
Andrew Cotter
Jan Pfeifer
Alexander Mangylov
Wojciech Moczydlowski
Alexander van Esbroeck
Journal Machine Learning Research (JMLR) (2016)
Preview abstract
Real-world machine learning applications may require functions to be interpretable and fast to evaluate, in addition to accurate. In particular, guaranteed monotonicity of the learned function can be critical to user trust. We propose meeting these three goals for low-dimensional machine learning problems by learning flexible, monotonic functions using calibrated interpolated look-up tables. We extend the structural risk minimization framework
of lattice regression to train monotonic look-up tables by solving a convex prob-
lem with appropriate linear inequality constraints. In addition, we propose jointly learning interpretable calibrations of each feature to normalize continuous features and handle categorical or missing data, though this changes the optimization problem to be non-convex. We address large-scale learning through parallelization, mini-batching, and propose random sampling of additive regularizer terms. Experiments on seven real-world problems with five to sixteen features and thousands to millions of training samples show the proposed monotonic functions can achieve state-of-the-art accuracy on practical problems while providing greater transparency to users.
View details