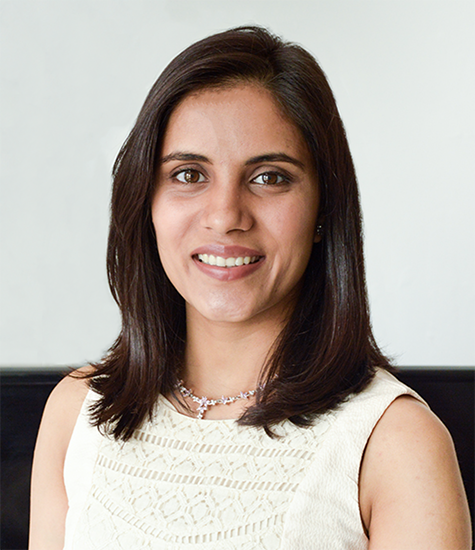
Mahima Pushkarna
I am a UX Designer at Google AI, where I design novel tools and frameworks for understanding machine learning models and interpreting decisions made by algorithms.
I tend to wear many hats – UI/UX, visual design, strategy and design research - to create better human-ai partnerships, such as helping doctors diagnose cancer using AI. The products and processes that I have designed, such as the What-If Tool and Facets, have been widely used to advance better practices in machine learning in industry and academia. As a part of Google's People + AI Research Initiative, I study the impact of design on AI, and vice-versa.
I hold an MFA in Information Design & Data Visualization from Northeastern University, (Boston, MA) and previously studied design at Srishti School of Art, Design & Technology (Bangalore, India) and the University of Michigan, Ann Arbor.
I tend to wear many hats – UI/UX, visual design, strategy and design research - to create better human-ai partnerships, such as helping doctors diagnose cancer using AI. The products and processes that I have designed, such as the What-If Tool and Facets, have been widely used to advance better practices in machine learning in industry and academia. As a part of Google's People + AI Research Initiative, I study the impact of design on AI, and vice-versa.
I hold an MFA in Information Design & Data Visualization from Northeastern University, (Boston, MA) and previously studied design at Srishti School of Art, Design & Technology (Bangalore, India) and the University of Michigan, Ann Arbor.
Authored Publications
Sort By
LLM Comparator: Visual Analytics for Side-by-Side Evaluation of Large Language Models
Minsuk Kahng
Michael Xieyang Liu
Krystal Kallarackal
Extended Abstracts of the CHI Conference on Human Factors in Computing Systems (CHI EA '24), ACM (2024)
Preview abstract
Automatic side-by-side evaluation has emerged as a promising approach to evaluating the quality of responses from large language models (LLMs). However, analyzing the results from this evaluation approach raises scalability and interpretability challenges. In this paper, we present LLM Comparator, a novel visual analytics tool for interactively analyzing results from automatic side-by-side evaluation. The tool supports interactive workflows for users to understand when and why a model performs better or worse than a baseline model, and how the responses from two models are qualitatively different. We iteratively designed and developed the tool by closely working with researchers and engineers at Google. This paper details the user challenges we identified, the design and development of the tool, and an observational study with participants who regularly evaluate their models.
View details
Believing Anthropomorphism: Examining the Role of Anthropomorphic Cues on User Trust in Large Language Models
Michelle Cohn
Femi Olanubi
Zion Mengesha
Daniel Padgett
CM (Association of Computing Machinery) CHI conference on Human Factors in Computing Systems 2024 (2024)
Preview abstract
People now regularly interface with Large Language Models (LLMs) via speech and text (e.g., Bard) interfaces. However, little is known about the relationship between how users anthropomorphize an LLM system (i.e., ascribe human-like characteristics to a system) and how they trust the information the system provides. Participants (n=2,165; ranging in age from 18-90 from the United States) completed an online experiment, where they interacted with a pseudo-LLM that varied in modality (text only, speech + text) and grammatical person (“I” vs. “the system”) in its responses. Results showed that the “speech + text” condition led to higher anthropomorphism of the system overall, as well as higher ratings of accuracy of the information the system provides. Additionally, the first-person pronoun (“I”) led to higher information accuracy and reduced risk ratings, but only in one context. We discuss these findings for their implications for the design of responsible, human–generative AI experiences.
View details
Data Cards: Purposeful and Transparent Dataset Documentation for Responsible AI
Association for Computing MachineryNew YorkNYUnited States (2022)
Preview abstract
As we move towards large-scale models capable of numerous downstream tasks, the complexity of understanding multi-modal datasets that give nuance to models rapidly increases. A clear and thorough understanding of a dataset's origins, development, intent, ethical considerations and evolution becomes a necessary step for the responsible and informed deployment of models, especially those in people-facing contexts and high-risk domains. However, the burden of this understanding often falls on the intelligibility, conciseness, and comprehensiveness of its documentation. However, the burden of this understanding often falls on the intelligibility, conciseness, and comprehensiveness of the documentation, and consistency and comparability across the documentation of all datasets involved, and as such documentation must be treated as a user-centric product in and of itself. In this paper, we propose Data Cards for fostering transparent, purposeful and human-centered documentation of datasets within the practical contexts of industry and research. Data Cards are structured summaries of essential facts about various aspects of ML datasets needed by stakeholders across a dataset's lifecycle for responsible AI development. These summaries provide explanations of processes and rationales that shape the data and consequently the models—such as upstream sources, data collection and annotation methods; training and evaluation methods, intended use, or decisions affecting model performance. We also present evaluative frameworks that ground Data Cards in real-world utility and human-centricity. Using two case studies, we report on desirable characteristics that support adoption across domains, organizational structures, and audience groups. Finally, we present lessons learned from deploying over twenty Data Cards.
View details
LaMPost: Evaluation of an AI-assisted Writing Email Editor Prototype for Adults with Dyslexia
Steven Goodman
Erin Buehler
Patrick Clary
Andy Coenen
Aaron Michael Donsbach
Tiffanie Horne
Bob MacDonald
Rain Breaw Michaels
Ajit Narayanan
Joel Christopher Riley
Alex Santana
Rachel Sweeney
Phil Weaver
Ann Yuan
Proceedings of ASSETS 2022, ACM (2022) (to appear)
Preview abstract
Prior work has explored the writing challenges experienced by people with dyslexia, and the potential for new spelling, grammar, and word retrieval technologies to address these challenges. However, the capabilities for natural language generation demonstrated by the latest class of large language models (LLMs) highlight an opportunity to explore new forms of human-AI writing support tools. In this paper, we introduce LaMPost, a prototype email-writing interface that explores the potential for LLMs to power writing support tools that address the varied needs of people with dyslexia. LaMPost draws from our understanding of these needs and introduces novel AI-powered features for email-writing, including: outlining main ideas, generating a subject line, suggesting changes, rewriting a selection. We evaluated LaMPost with 19 adults with dyslexia, identifying many promising routes for further exploration (including the popularity of the “rewrite” and “subject line” features), but also finding that the current generation of LLMs may not surpass the accuracy and quality thresholds required to meet the needs of writers with dyslexia. Surprisingly, we found that participants’ awareness of the AI had no effect on their perception of the system, nor on their feelings of autonomy, expression, and self-efficacy when writing emails. Our findings yield further insight into the benefits and drawbacks of using LLMs as writing support for adults with dyslexia and provide a foundation to build upon in future research.
View details
Healthsheet: development of a transparency artifact for health datasets
Diana Mincu
Lauren Wilcox
Jessica Schrouff
Razvan Adrian Amironesei
Nyalleng Moorosi
ACM FAccT Conference 2022, ACM (2022)
Preview abstract
Machine learning (ML) approaches have demonstrated promising results in a wide range of healthcare applications. Data plays a crucial role in developing ML-based healthcare systems that directly affect people’s lives. Many of the ethical issues surrounding the use of ML in healthcare stem from structural inequalities underlying the way we collect, use, and handle data. Developing guidelines to improve documentation practices regarding the creation, use, and maintenance of ML healthcare datasets is therefore of critical importance. In this work, we introduce Healthsheet, a contextualized adaptation of the original datasheet questionnaire for health-specific applications. Through a series of semi-structured interviews, we adapt the datasheets for healthcare data documentation. As part of the Healthsheet development process and to understand the obstacles researchers face in creating datasheets, we worked with three publicly-available healthcare datasets as our case studies, each with different types of structured data: Electronic health Records (EHR), clinical trial study data, and smartphone-based performance outcome measures. Our findings from the interviewee study and case studies show 1) that datasheets should be contextualized for healthcare, 2) that despite incentives to adopt accountability practices such as datasheets, there is a lack of consistency in the broader use of these practices 3) how the ML for health community views datasheets and particularly Healthsheets as diagnostic tool to surface the limitations and strength of datasets and 4) the relative importance of different fields in the datasheet to healthcare concerns.
View details
The Language Interpretability Tool: Extensible, Interactive Visualizations and Analysis for NLP Models
Andy Coenen
Sebastian Gehrmann
Ellen Jiang
Carey Radebaugh
Ann Yuan
Proceedings of the 2020 Conference on Empirical Methods in Natural Language Processing: System Demonstrations, Association for Computational Linguistics (to appear)
Preview abstract
We present the Language Interpretability Tool (LIT), an open-source platform for visualization and understanding of NLP models. We focus on core questions about model behavior: Why did my model make this prediction? When does it perform poorly? What happens under a controlled change in the input? LIT integrates local explanations, aggregate analysis, and counterfactual generation into a streamlined, browser-based interface to enable rapid exploration and error analysis. We include case studies for a diverse set of workflows, including exploring counterfactuals for sentiment analysis, measuring gender bias in coreference systems, and exploring local behavior in text generation. LIT supports a wide range of models--including classification, seq2seq, and structured prediction--and is highly extensible through a declarative, framework-agnostic API. LIT is under active development, with code and full documentation available at https://github.com/pair-code/lit.
View details
Preview abstract
A key challenge in developing and deploying Machine Learning (ML) systems is understanding their performance across a wide range of inputs. To address this challenge, we created the What-If Tool, an open-source application that allows practitioners to probe, visualize, and analyze ML systems, with minimal coding. The What-If Tool lets practitioners test performance in hypothetical situations, analyze the importance of different data features, and visualize model behavior across multiple models and subsets of input data. It also lets practitioners measure systems according to multiple ML fairness metrics. We describe the design of the tool, and report on real-life usage at different organizations.
View details