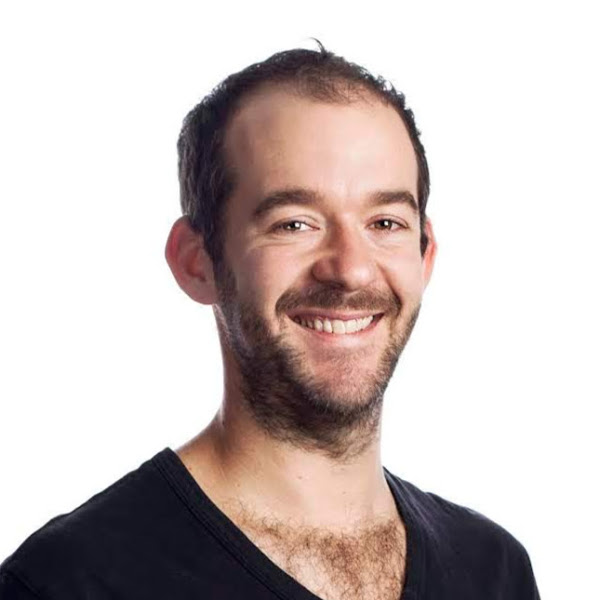
Marc van Zee
AI researcher interested in commonsense reasoning, natural language understanding, and compositional generalization.
Research Areas
Authored Publications
Sort By
Measuring Compositional Generalization: A Comprehensive Method on Realistic Data
Nathanael Schärli
Nathan Scales
Hylke Buisman
Daniel Furrer
Nikola Momchev
Danila Sinopalnikov
Lukasz Stafiniak
Tibor Tihon
Dmitry Tsarkov
ICLR (2020)
Preview abstract
State-of-the-art machine learning methods exhibit limited compositional generalization. At the same time, there is a lack of realistic benchmarks that comprehensively measure this ability, which makes it challenging to find and evaluate improvements. We introduce a novel method to systematically construct such benchmarks by maximizing compound divergence while guaranteeing a small atom divergence between train and test sets, and we quantitatively compare this method to other approaches for creating compositional generalization benchmarks. We present a large and realistic natural language question answering dataset that is constructed according to this method, and we use it to analyze the compositional generalization ability of three machine learning architectures. We find that they fail to generalize compositionally and that there is a surprisingly strong negative correlation between compound divergence and accuracy. We also demonstrate how our method can be used to create new compositionality benchmarks on top of the existing SCAN dataset, which confirms these findings.
View details
Preview abstract
Goal-oriented requirements modeling approaches aim at capturing intentions of various stakeholders involved in the development of an information system and refining them into low level goals and tasks. The process of constructing a goal model usually involves discussions between a requirements engineer and a group of stakeholders. While it is possible to capture part of this discussion process in a goal model, by specifying alternative solutions for a goal, not all of the arguments can be found back in the resulting model. For instance, the discussion on whether to accept or reject a certain goal and the ultimate rationale for acceptance or rejection cannot be captured in current goal modeling languages. In this paper, we apply argumentation techniques from artificial intelligence to a goal modeling approach by using a coding analysis in which stakeholders discuss requirements for a Traffic Simulator. We combine a simplified version of a traditional goal modeling approach, the Goal-oriented Requirements Language (GRL), with a formal Practical Reasoning Argument Scheme (PRAS) for reasoning about goals into a new framework (RationalGRL). RationalGRL provides a formal semantics and tool support to capture the discussions and outcomes of the argumentation process that leads to a goal model. We also define the RationalGRL development process to create a RationalGRL model.
View details
Compositional Generalization in Semantic Parsing: Pre-training vs. Specialized Architectures
Daniel Peter Furrer
Nathan Scales
Nathanael Schärli
arXiv e-prints (2020), arXiv:2007.08970
Preview abstract
While mainstream machine learning methods are known to have limited ability to compositionally generalize, new architectures and techniques continue to be proposed to address this limitation. We investigate state-of-the-art techniques and architectures in order to assess their effectiveness in improving compositional generalization in semantic parsing tasks based on the SCAN and CFQ datasets.
We show that masked language model (MLM) pre-training rivals SCAN-inspired architectures on primitive holdout splits. On a more complex compositional task, we show that pre-training leads to significant improvements in performance vs. comparable non-pre-trained models, whereas architectures proposed to encourage compositional generalization on SCAN or in the area of algorithm learning fail to lead to significant improvements.
We establish a new state of the art on the CFQ compositional generalization benchmark using MLM pre-training together with an intermediate representation.
View details
Intention as Commitment toward Time
Dragan Doder
Eric Pacuit
Leon van der Torre
Mehdi Dastani
Thomas Icard III
Artificial Intelligence Journal, 283 (2020)
Preview abstract
In this paper we address the interplay among intention, time, and belief in dynamic environments. The first contribution is a logic for reasoning about intention, time and belief, in which assumptions of intentions are represented by preconditions of intended actions. Intentions and beliefs are coherent as long as these assumptions are not violated, i.e. as long as intended actions can be performed such that their preconditions hold as well. The second contribution is the formalization of what-if scenarios: what happens with intentions and beliefs if a new intention is adopted, or a new fact is learned? An agent is committed to its intended actions as long as its belief-intention database is coherent. We conceptualize intention as commitment toward time and we develop AGM-based postulates for the iterated revision of belief-intention databases, and we prove a Katsuno-Mendelzon-style representation theorem.
View details