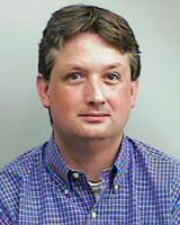
Michiel Bacchiani
Research Areas
Authored Publications
Sort By
LibriTTS-R: Restoration of a Large-Scale Multi-Speaker TTS Corpus
Yifan Ding
Kohei Yatabe
Nobuyuki Morioka
Yu Zhang
Wei Han
Interspeech 2023 (2023)
Preview abstract
This paper introduces a new speech dataset called ``LibriTTS-R'' designed for text-to-speech (TTS) use. It is derived by applying speech restoration to the LibriTTS corpus, which consists of 585 hours of speech data at 24 kHz sampling rate from 2,456 speakers and the corresponding texts. The constituent samples of LibriTTS-R are identical to those of LibriTTS, with only the sound quality improved. Experimental results show that the LibriTTS-R ground-truth samples showed significantly improved sound quality compared to those in LibriTTS. In addition, neural end-to-end TTS trained with LibriTTS-R achieved speech naturalness on par with that of the ground-truth samples. The corpus is freely available for download from [URL-HERE]
View details
Miipher: A Robust Speech Restoration Model Integrating Self-Supervised Speech Representation and Linguistic Features
Yifan Ding
Kohei Yatabe
Nobuyuki Morioka
Yu Zhang
Wei Han
WASPAA 2023 (2023) (to appear)
Preview abstract
Speech restoration (SR) is a task of converting degraded speech signals into high-quality ones. In this study, we propose a robust SR model called Miipher, and apply Miipher to a new SR application: increasing the amount of high-quality training data for speech generation by converting speech samples collected from the web to studio-quality. To make our SR model robust against various degradation, we use (i) a speech representation extracted from w2v-BERT for the input feature, and (ii) linguistic features extracted from transcripts and PnG-BERT for conditioning features. Experiments show that the proposed model (i) is robust against various audio degradation, (ii) can restore samples in the LJspeech dataset and improves the quality of text-to-speech (TTS) outputs without changing the model and hyper-parameters, and (iii) enable us to train a high-quality TTS model from restored speech samples collected from the web.
View details
WaveFit: An Iterative and Non-autoregressive Neural Vocoder based on Fixed-Point Iteration
Kohei Yatabe
Proc. IEEE Spoken Language Technology Workshop (SLT) (2022) (to appear)
Preview abstract
Denoising diffusion probabilistic models (DDPMs) and generative adversarial networks (GANs) are popular generative models for neural vocoders. The DDPMs and GANs can be characterized by the iterative denoising framework and adversarial training, respectively. This study proposes a fast and high-quality neural vocoder called WaveFit, which integrates the essence of GANs into a DDPM-like iterative framework based on fixed-point iteration. WaveFit iteratively denoises an input signal, and trains a deep neural network (DNN) for minimizing an adversarial loss calculated from intermediate outputs at all iterations. Subjective (side-by-side) listening tests showed no statistically significant differences in naturalness between human natural speech and those synthesized by WaveFit with five iterations. Furthermore, the inference speed of WaveFit was more than 240 times faster than WaveRNN. Audio demos are available at google.github.io/df-conformer/wavefit/.
View details
SNRi Target Training for Joint Speech Enhancement and Recognition
Sankaran Panchapagesan
Proc. Interspeech (2022) (to appear)
Preview abstract
Speech enhancement (SE) is used as a frontend in speech applications including automatic speech recognition (ASR) and telecommunication. A difficulty in using the SE frontend is that the appropriate noise reduction level differs depending on applications and/or noise characteristics. In this study, we propose ``{\it signal-to-noise ratio improvement (SNRi) target training}''; the SE frontend is trained to output a signal whose SNRi is controlled by an auxiliary scalar input. In joint training with a backend, the target SNRi value is estimated by an auxiliary network. By training all networks to minimize the backend task loss, we can estimate the appropriate noise reduction level for each noisy input in a data-driven scheme. Our experiments showed that the SNRi target training enables control of the output SNRi. In addition, the proposed joint training relatively reduces word error rate by 4.0\% and 5.7\% compared to a Conformer-based standard ASR model and conventional SE-ASR joint training model, respectively. Furthermore, by analyzing the predicted target SNRi, we observed the jointly trained network automatically controls the target SNRi according to noise characteristics. Audio demos are available in our demo page [google.github.io/df-conformer/snri_target/].
View details
SpecGrad: Diffusion Probabilistic Model based Neural Vocoder with Adaptive Noise Spectral Shaping
Kohei Yatabe
Nanxin Chen
Proc. Interspeech (2022) (to appear)
Preview abstract
Neural vocoder using denoising diffusion probabilistic model (DDPM) has been improved by adaptation of the diffusion noise distribution to given acoustic features. In this study, we propose SpecGrad that adapts the diffusion noise so that its time-varying spectral envelope becomes close to the conditioning log-mel spectrogram. This adaptation by time-varying filtering improves the sound quality especially in the high-frequency bands. It is processed in the time-frequency domain to keep the computational cost almost the same as the conventional DDPM-based neural vocoders. Experimental results showed that SpecGrad generates higher-fidelity speech waveform than conventional DDPM-based neural vocoders in both analysis-synthesis and speech enhancement scenarios. Audio demos are available at [wavegrad.github.io/specgrad/].
View details
Preview abstract
End-to-end speech recognition is a promising technology for enabling compact automatic speech recognition (ASR) systems since it can unify the acoustic and language model into a single neural network.
However, as a drawback, training of end-to-end speech recognizers always requires transcribed utterances.
Since end-to-end models are also known to be severely data hungry, this constraint is crucial especially because obtaining transcribed utterances is costly and can possibly be impractical or impossible.
This paper proposes a method for alleviating this issue by transferring knowledge from a language model neural network that can be pretrained with text-only data.
Specifically, this paper attempts to transfer semantic knowledge acquired in embedding vectors of large-scale language models.
Since embedding vectors can be assumed as implicit representations of linguistic information such as part-of-speech, intent, and so on, those are also expected to be useful modeling cues for ASR decoders.
This paper extends two types of ASR decoders, attention-based decoders and neural transducers, by modifying training loss functions to include embedding prediction terms.
The proposed systems were shown to be effective for error rate reduction without incurring extra computational costs in the decoding phase.
View details
DF-Conformer: Integrated architecture of Conv-TasNet and Conformer using linear complexity self-attention for speech enhancement
Lion Jones
Proc. IEEE Workshop Appl. Signal Process. Audio Acoust. (WASPAA) (2021)
Preview abstract
Combinations of a trainable filterbank and a mask prediction network is a strong framework in single-channel speech enhancement (SE). Since the denoising performance and computational efficiency are mainly affected by the structure of the mask prediction network, we aim to improve this network. In this study, by focusing on a similarity between the structure of Conv-TasNet and Conformer, we integrate the Conformer into SE as a mask prediction network to benefit its powerful sequential modeling ability. To improve the computational complexity and local sequential modeling, we extend the Conformer using linear complexity attention and stacked 1-D dilated depthwise convolution layers. Experimental results show that (i) the use of linear complexity attention avoids high computational complexity, and (ii) our model achieves higher scale-invariant signal-to-noise ratio than the improved time-dilated convolution network (TDCN++), an extended version of Conv-TasNet.
View details
A Comparative Study on Neural Architectures and Training Methods for Japanese Speech Recognition
Lion Jones
Interspeech 2021 (2021) (to appear)
Preview abstract
End-to-end (E2E) modeling is advantageous for automatic speech recognition (ASR) especially for Japanese since word-based tokenization of Japanese is not trivial, and E2E modeling is able to model character sequences directly. This paper focuses on the latest E2E modeling techniques, and investigates their performances on character-based Japanese ASR by conducting comparative experiments. The results are analyzed and discussed in order to understand the relative advantages of long short-term memory (LSTM), and Conformer models in combination with connectionist temporal classification, transducer, and attention-based loss functions. Furthermore, the paper investigates on effectivity of the recent training techniques such as data augmentation (SpecAugment), variational noise injection, and exponential moving average. The best configuration found in the paper achieved the state-of-the-art character error rates of 4.1%, 3.2%, and 3.5% for Corpus of Spontaneous Japanese (CSJ) eval1, eval2, and eval3 tasks, respectively. The system is also shown to be computationally efficient thanks to the efficiency of Conformer transducers.
View details
Preview abstract
This paper proposes methods to improve a commonly used end-to-end speech recognition model, Listen-Attend-Spell (LAS).
The methods we propose use multi-task learning to improve generalization of the model by leveraging information from multiple labels.
The focus in this paper is on multi-task models for simultaneous signal-to-grapheme and signal-to-phoneme conversions while sharing the encoder parameters.
Since phonemes are designed to be a precise description of the linguistic aspects of the speech signal, using phoneme recognition as an auxiliary task can help guiding the early stages of training to be more stable.
In addition to conventional multi-task learning, we obtain further improvements by introducing a method that can exploit dependencies between labels in different tasks. Specifically, the dependencies between phonemes and grapheme sequences are considered. In conventional multi-task learning these sequences are assumed to be independent. Instead, in this paper, a joint model is proposed based on ``iterative refinement'' where dependency modeling is achieved by a multi-pass strategy.
The proposed method is evaluated on a 28000h corpus of Japanese speech data. Performance of a conventional multi-task approach is contrasted with that of the joint model with iterative refinement.
View details
State-of-the-art Speech Recognition With Sequence-to-Sequence Models
Chung-Cheng Chiu
Patrick Nguyen
Katya Gonina
Navdeep Jaitly
Jan Chorowski
ICASSP (2018) (to appear)
Preview abstract
Attention-based encoder-decoder architectures such as Listen, Attend, and Spell (LAS), subsume the acoustic, pronunciation and language model components of a traditional automatic speech recognition (ASR) system into a single neural network. In our previous work, we have shown that such architectures are comparable to state-of-the-art ASR systems on dictation tasks, but it was not clear if such architectures would be practical for more challenging tasks such as voice search. In this work, we explore a variety of structural and optimization improvements to our LAS model which significantly improve performance. On the structural side, we show that word piece models can be used instead of graphemes. We introduce a multi-head attention architecture, which offers improvements over the commonly-used single-head attention. On the optimization side, we explore techniques such as synchronous training, scheduled sampling, label smoothing, and minimum word error rate optimization, which are all shown to improve accuracy. We present results with a unidirectional LSTM encoder for streaming recognition. On a 12,500 hour voice search task, we find that the proposed changes improve the WER of the LAS system from 9.2% to 5.6%, while the best conventional system achieve 6.7% WER. We also test both models on a dictation dataset, and our model provide 4.1% WER while the conventional system provides 5% WER.
View details