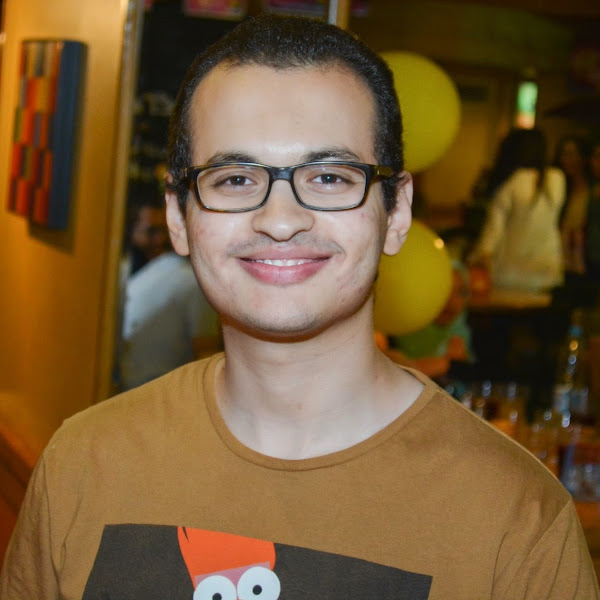
Mohamed Hammad
I am an AI researcher with a focus on recommender systems and personalization. My passion lies in developing intelligent and efficient algorithms that enhance user experiences through tailored recommendations. I am particularly interested in the intersection of LLMs, AI, personalization, and RecSys.
Research Areas
Authored Publications
Sort By
CALRec: Contrastive Alignment of Generative LLMs For Sequential Recommendation
Keyi Yu
18th ACM Conference on Recommender Systems (RecSys 2024) (2024) (to appear)
Preview abstract
Personalized recommendation requires understanding both the candidate items and user preferences. Traditional collaborative filtering approaches rely on embedding users and items in the same representation space while recent efforts formulate the problem into sequential user activity modeling and future activity prediction tasks. Some of the most recent efforts leverage autoregressive large language models to directly generate the recommendation. This work proposes CALRec, a sequential recommendation framework aligning the generative task based on PaLM-2 LLM with contrastive learning tasks for user/item understanding. To leverage the strong generalization capabilities of the state-of-the-art pretrained LLMs, our input consists of pure texts following differentiable text templates for user inputs and item inputs. We propose novel ways of combining generative loss and contrastive losses in multi-category joint continuous pretraining, followed by domain-specific finetuning. During training, the LLM backbone trains in a two-tower fashion to comprehend users’ consecutive behaviors and descriptions of individual items. Our model outperforms many state-of-the-art baselines significantly especially in ranking tasks. Our systematic ablation study reveals that (i) multi-category pretraining and domain-adaptation finetuning are both important and deliver better performance when combined, and (ii) contrastive alignment further improves the quality among many categories of the Amazon review dataset.
View details