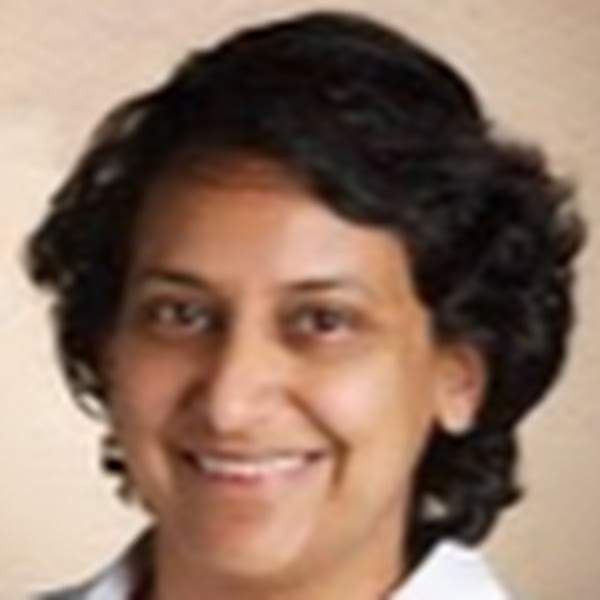
Nita Goyal
Research Areas
Authored Publications
Sort By
A scalable system to measure contrail formation on a per-flight basis
Erica Brand
Sebastian Eastham
Carl Elkin
Thomas Dean
Zebediah Engberg
Ulrike Hager
Joe Ng
Dinesh Sanekommu
Marc Shapiro
Environmental Research Communications (2024)
Preview abstract
In this work we describe a scalable, automated system to determine from satellite data whether a given flight has made a persistent contrail.
The system works by comparing flight segments to contrails detected by a computer vision algorithm running on images from the GOES-16 Advanced Baseline Imager. We develop a `flight matching' algorithm and use it to label each flight segment as a `match' or `non-match'. We perform this analysis on 1.6 million flight segments and compare these labels to existing contrail prediction methods based on weather forecast data. The result is an analysis of which flights make persistent contrails several orders of magnitude larger than any previous work. We find that current contrail prediction models fail to correctly predict whether we will match a contrail in many cases.
View details
Contrail Detection on GOES-16 ABI with the OpenContrails Dataset
Joe Ng
Jian Cui
Vincent Rudolf Meijer
Erica Brand
IEEE Transactions on Geoscience and Remote Sensing (2023)
Preview abstract
Contrails (condensation trails) are line-shaped ice clouds caused by aircraft and are a substantial contributor to aviation-induced climate change. Contrail avoidance is potentially an inexpensive way to significantly reduce the climate impact of aviation. An automated contrail detection system is an essential tool to develop and evaluate contrail avoidance systems. In this article, we present a human-labeled dataset named OpenContrails to train and evaluate contrail detection models based on GOES-16 Advanced Baseline Imager (ABI) data. We propose and evaluate a contrail detection model that incorporates temporal context for improved detection accuracy. The human labeled dataset and the contrail detection outputs are publicly available on Google Cloud Storage at gs://goes_contrails_dataset .
View details
Next Day Wildfire Spread: A Machine Learning Dataset to Predict Wildfire Spreading From Remote-Sensing Data
Fantine Huot
Lily Hu
Matthias Ihme
Yi-fan Chen
IEEE Transactions on Geoscience and Remote Sensing, 60 (2022), pp. 1-13
Preview abstract
Predicting wildfire spread is critical for land management and disaster preparedness. To this end, we present “Next Day Wildfire Spread,” a curated, large-scale, multivariate dataset of historical wildfires aggregating nearly a decade of remote-sensing data across the United States. In contrast to existing fire datasets based on Earth observation satellites, our dataset combines 2-D fire data with multiple explanatory variables (e.g., topography, vegetation, weather, drought index, and population density) aligned over 2-D regions, providing a feature-rich dataset for machine learning. To demonstrate the usefulness of this dataset, we implement a neural network that takes advantage of the spatial information of these data to predict wildfire spread. We compare the performance of the neural network with other machine learning models: logistic regression and random forest. This dataset can be used as a benchmark for developing wildfire propagation models based on remote-sensing data for a lead time of one day.
View details