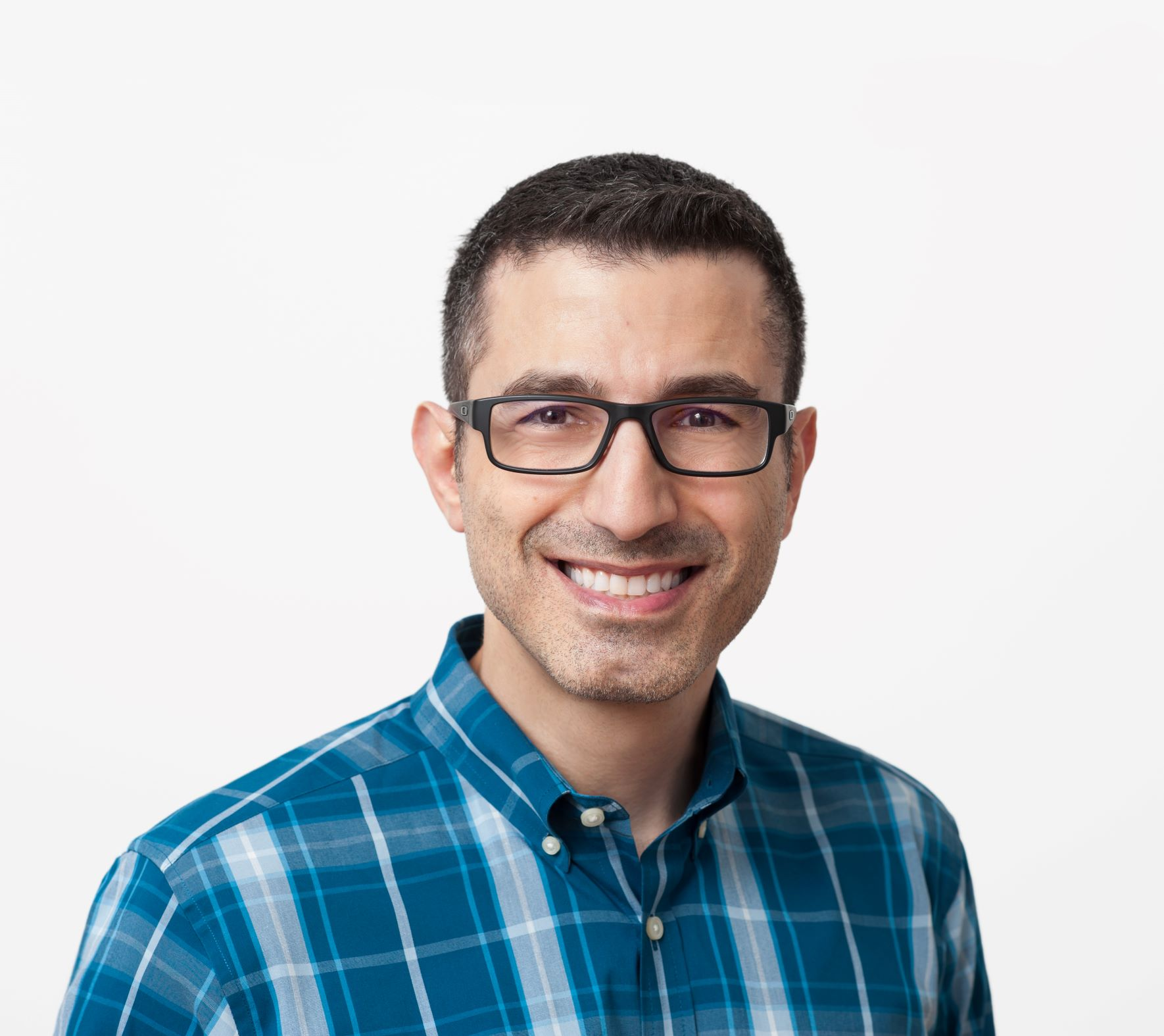
Petros Maniatis
Petros Maniatis is a Senior Staff Research Scientist at Google DeepMind, in the Learning for Code Team. Prior to that, he was a Senior Research Scientist at Intel Labs, working in Intel's Berkeley Research Lab and then at the Intel Science and Technology Center on Secure Computing at UC Berkeley. He received his MSc and Ph.D. from the Computer Science Department at Stanford University. Before Stanford, he obtained his BSc with honors at the Department of Informatics of the University of Athens in Greece. His current research interests lie primarily in the confluence of machine learning and software engineering.
Authored Publications
Sort By
AI-assisted Assessment of Coding Practices in Industrial Code Review
Malgorzata (Gosia) Salawa
Ivan Budiselic
Marko Ivanković
Juanjo Carin
Mateusz Lewko
Jovan Andonov
Rene Just
2024
Preview abstract
Modern code review is a process in which incremental code contributions made by one software developer are reviewed by one or more peers before it is committed to the version control system. An important element of modern code review is verifying that the code under review adheres to style guidelines and best practices of the corresponding programming language. Some of these rules are universal and can be checked automatically or enforced via code formatters. Other rules, however, are context-dependent and the corresponding checks are commonly left to developers who are experts in the given programming language and whose time is expensive. Many automated systems have been developed that attempt to detect various rule violations without any human intervention. Historically, such systems implement targeted analyses and were themselves expensive to develop. This paper presents AutoCommenter, a system that uses a state of the art large language model to automatically learn and enforce programming language best practices. We implemented AutoCommenter for four programming languages: C++, Java, Python and Go. We evaluated its performance and adoption in a large industrial setting. Our evaluation shows that a model that automatically learns language best practices is feasible and has a measurable positive impact on the developer workflow. Additionally, we present the challenges we faced when deploying such a model to tens of thousands of developers and provide lessons we learned for any practitioners that would like to replicate the work or build on top of it.
View details
CodeQueries: A Dataset of Semantic Queries over Code
Surya Prakash Sahu
Madhurima Mandal
Shikhar Bharadwaj
Aditya Kanade
Shirish Shevade
Innovations in Software Engineering (ISEC), ACM, Bangalore, India (2024)
Preview abstract
Developers often have questions about semantic aspects of code
they are working on, e.g., “Is there a class whose parent classes
declare a conflicting attribute?”. Answering them requires understanding code semantics such as attributes and inheritance relation
of classes. An answer to such a question should identify code spans
constituting the answer (e.g., the declaration of the subclass) as well
as supporting facts (e.g., the definitions of the conflicting attributes).
The existing work on question-answering over code has considered
yes/no questions or method-level context. We contribute a labeled
dataset, called CodeQueries, of semantic queries over Python code.
Compared to the existing datasets, in CodeQueries, the queries
are about code semantics, the context is file level and the answers
are code spans. We curate the dataset based on queries supported
by a widely-used static analysis tool, CodeQL, and include both
positive and negative examples, and queries requiring single-hop
and multi-hop reasoning.
To assess the value of our dataset, we evaluate baseline neural
approaches. We study a large language model (GPT3.5-Turbo) in
zero-shot and few-shot settings on a subset of CodeQueries. We
also evaluate a BERT style model (CuBERT) with fine-tuning. We
find that these models achieve limited success on CodeQueries.
CodeQueries is thus a challenging dataset to test the ability of
neural models, to understand code semantics, in the extractive
question-answering setting
View details
Resolving Code Review Comments with Machine Learning
Alexander Frömmgen
Peter Choy
Elena Khrapko
Marcus Revaj
2024 IEEE/ACM 46th International Conference on Software Engineering: Software Engineering in Practice (ICSE-SEIP) (to appear)
Preview abstract
Code reviews are a critical part of the software development process, taking a significant amount of the code authors’ and the code reviewers’ time. As part of this process, the reviewer inspects the proposed code and asks the author for code changes through comments written in natural language. At Google, we see millions of reviewer comments per year, and authors require an average of ∼60 minutes active shepherding time between sending changes for review and finally submitting the change. In our measurements, the required active work time that the code author must devote to address reviewer comments grows almost linearly with the number of comments. However, with machine learning (ML), we have an opportunity to automate and streamline the code-review process, e.g., by proposing code changes based on a comment’s text.
We describe our application of recent advances in large sequence models in a real-world setting to automatically resolve code-review comments in the day-to-day development workflow at Google. We present the evolution of this feature from an asynchronous generation of suggested edits after the reviewer sends feedback, to an interactive experience that suggests code edits to the reviewer at review time. In deployment, code-change authors at Google address 7.5% of all reviewer comments by applying an ML-suggested edit. The impact of this will be to reduce the time spent on code reviews by hundreds of thousands of engineer hours annually at Google scale. Unsolicited, very positive feedback highlights that the impact of ML-suggested code edits increases Googlers’ productivity and allows them to focus on more creative and complex tasks.
View details
Predicting Dynamic Properties of Heap Allocations Using Neural Networks Trained on Static Code
Christian Navasca
Guoqing Harry Xu
2023 ACM SIGPLAN International Symposium on Memory Management (ISMM 2023)
Preview abstract
Memory allocators and runtime systems can leverage dynamic properties of heap allocations – such as object lifetimes, hotness or access correlations – to improve performance and resource consumption. A significant amount of work has focused on approaches that collect this information in performance profiles and then use it in new memory allocator or runtime designs, both offline (in ahead-of-time compilers) and online (in JIT compilers). This is a special instance of profile-guided optimization.
This approach has significant disadvantages: 1) The profiling oftentimes introduces substantial overheads, which are prohibitive in many production scenarios, 2) Creating a representative profiling run adds significant engineering complexity and reduces deployment velocity, and 3) Profiles gathered ahead of time or during the warm-up phase of a server are often not representative of all workload behavior and may miss important corner cases.
In this paper, we investigate a fundamentally different approach. Instead of deriving heap allocation properties from profiles, we explore the ability of neural network models to predict them from the statically available code. As an intellectual abstract, we do not offer a conclusive answer but describe the trade-off space of this approach, investigate promising directions, motivate these directions with data analysis and experiments, and highlight challenges that future work needs to overcome.
View details
Snowcat: Efficient Kernel Concurrency Testing using a Learned Coverage Predictor
Sishuai Gong
Dinglan Peng
Pedro Fonseca
Symposium on Operating Systems Principles (SOSP) (2023)
Preview abstract
Random-based approaches and heuristics are commonly
used in kernel concurrency testing due to the massive scale
of modern kernels and corresponding interleaving space.
The lack of accurate and scalable approaches to analyze concurrent
kernel executions makes existing testing approaches
heavily rely on expensive dynamic executions to measure
the effectiveness of a new test. Unfortunately, the high cost
incurred by dynamic executions limits the breadth of the
exploration and puts latency pressure on finding effective
concurrent test inputs and schedules, hindering the overall
testing effectiveness.
This paper proposes Snowcat, a kernel concurrency testing
framework that generates effective test inputs and schedules
using a learned kernel block-coverage predictor. Using a
graph neural network, the coverage predictor takes a concurrent
test input and scheduling hints and outputs a prediction
on whether certain important code blocks will be executed.
Using this predictor, Snowcat can skip concurrent tests that
are likely to be fruitless and prioritize the promising ones
for actual dynamic execution.
After testing the Linux kernel for over a week, Snowcat
finds ∼17% more potential data races, by prioritizing tests of
more fruitful schedules than existing work would have chosen.
Snowcat can also find effective test inputs that expose
new concurrency bugs with higher probability (1.4×∼2.6×),
or reproduce known bugs more quickly (15×) than state-ofart
testing tools. More importantly, Snowcat is shown to be
more efficient at reaching a desirable level of race coverage
in the continuous setting, as the Linux kernel evolves from
version to version. In total, Snowcat discovered 17 new concurrency
bugs in Linux kernel 6.1, of which 13 are confirmed
and 6 are fixed.
View details
Learning to Walk over Relational Graphs of Source Code
Pardis Pashakhanloo
Aaditya Naik
Hanjun Dai
Mayur Naik
Deep Learning for Code (DL4C) Workshop @ ICLR 2022 (2022)
Preview abstract
Information-rich relational graphs have shown great potential in designing effective representations of code for program-understanding tasks. However, the
wealth of structural and semantic information in such graphs can overwhelm models, because of their limited input size. A promising approach for overcoming this
challenge is to gather presumed-relevant but smaller context from a larger graph,
and random walks over graphs was one of the first such approaches discovered.
We propose a deep-learning approach that improves upon random walks by learning task-specific walk policies that guide the traversal of the graph towards the
most relevant context. In the setting of relational graphs representing programs
and their semantic properties, we observe that models that employ learned policies for guiding walks are 6--36% points more accurate than models that employ
uniform random walks, and 0.2--3.5% points more accurate than models that employ expert knowledge for guiding the walks.
View details
Preview abstract
Designing a suitable representation for code-reasoning tasks is challenging in
aspects such as the kinds of program information to model, how to combine them,
and how much context to consider. We propose CodeTrek, a deep learning approach
that addresses these challenges by representing codebases as databases that conform
to rich relational schemas. The relational representation not only allows CodeTrek
to uniformly represent diverse kinds of program information, but also to leverage
program-analysis queries to derive new semantic relations, which can be readily
incorporated without further architectural engineering. CodeTrek embeds this
relational representation using a set of walks that can traverse different relations
in an unconstrained fashion, and incorporates all relevant attributes along the way.
We evaluate CodeTrek on four diverse and challenging Python tasks: variable
misuse, exception prediction, unused definition, and variable shadowing. CodeTrek
achieves an accuracy of 91%, 63%, 98%, and 94% on these tasks respectively, and
outperforms state-of-the-art neural models by 2--19% points.
View details
Learning to Answer Semantic Queries over Code
Surya Prakash Sahu
Madhurima Mandal
Shikhar Bharadwaj
Aditya Kanade
Shirish Shevade
Google Research (2022)
Preview abstract
During software development, developers need answers to queries about semantic
aspects of code. Even though extractive question-answering using neural approaches has been studied widely in natural languages, the problem of answering
semantic queries over code using neural networks has not yet been explored. This
is mainly because there is no existing dataset with extractive question and answer pairs over code involving complex concepts and long chains of reasoning.
We bridge this gap by building a new, curated dataset called CodeQueries, and
proposing a neural question-answering methodology over code.
We build upon state-of-the-art pre-trained models of code to predict answer and
supporting-fact spans. Given a query and code, only some of the code may be
relevant to answer the query. We first experiment under an ideal setting where
only the relevant code is given to the model and show that our models do well. We
then experiment under three pragmatic considerations: (1) scaling to large-size
code, (2) learning from a limited number of examples and (3) robustness to minor
syntax errors in code. Our results show that while a neural model can be resilient
to minor syntax errors in code, increasing size of code, presence of code that is not
relevant to the query, and reduced number of training examples limit the model
performance. We are releasing our data and models
to facilitate future work on
the proposed problem of answering semantic queries over code.
View details
Graph Representations of Python Programs via Source-level Static Analysis
Daniel Johnson
David Bieber
Vincent Josua Hellendoorn
Arxiv (2022)
Preview abstract
Graph representations of programs are commonly a central element of machine learning for code research. We introduce an open source Python library python_graphs that applies static analysis to construct graph representations of Python programs suitable for training machine learning models. Our library admits the construction of control-flow graphs, data-flow graphs, and composite "program graphs" that combine control-flow, data-flow, syntactic, and lexical information about a program. We present the capabilities and limitations of the library, perform a case-study applying the library to millions of competitive programming submissions, and showcase the library's utility for machine learning research.
View details
PLUR: A Unifying, Graph-Based View of Program Learning, Understanding, and Repair
Zimin Chen
Vincent J Hellendoorn
Subhodeep Moitra
Thirty-fifth Conference on Neural Information Processing Systems (NeurIPS 2021) (2021)
Preview abstract
Machine learning for understanding and editing source code has recently attracted significant interest, with many developments in new models,
new code representations, and new tasks.
This proliferation can appear disparate and disconnected, making each approach seemingly unique and incompatible, thus obscuring the core
machine learning challenges and contributions.
In this work, we demonstrate that the landscape can be significantly simplified by taking a general approach of mapping a graph to a sequence of tokens and pointers.
Our main result is to show that 16 recently published tasks of different shapes can be cast in this form, based on which a single model architecture achieves near or above state-of-the-art results on nearly all tasks, outperforming custom models like code2seq and alternative generic models like Transformers.
This unification further enables multi-task learning and a series of cross-cutting experiments about the importance of different modeling choices for code understanding and repair tasks.
The full framework, called PLUR, is easily extensible to more tasks, and will be open-sourced (link).
View details