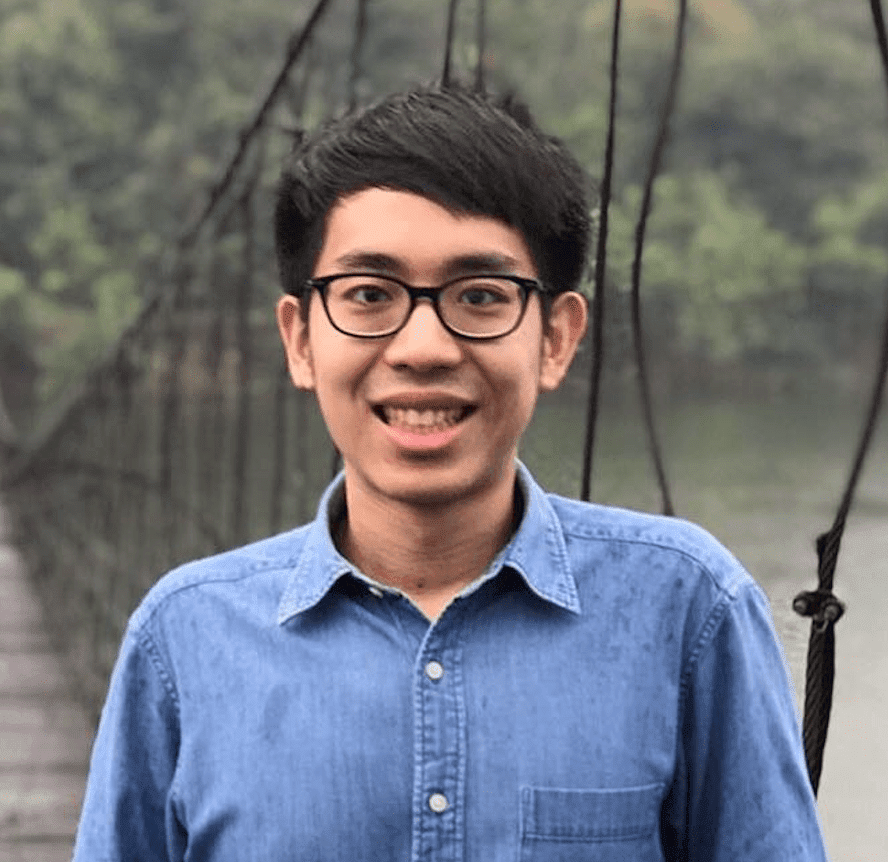
Piyawat L Kumjorn
Piyawat is currently a research scientist working with the Android Input Research team at Google. Early 2022, he obtained a Ph.D. from the Department of Computing, Imperial College London where he conducted research on the intersection of text classification, explainable AI, and human-AI interaction. He has served as an area chair for ACL and EMNLP, and also a program committee member for several top-tier AI/NLP conferences such as NeurIPS, IJCAI, AAAI, NAACL, and EACL.
Authored Publications
Sort By
Rambler: Supporting Writing With Speech via LLM-Assisted Gist Manipulation
Susan Lin
Jeremy Warner
J.D. Zamfirescu-Pereira
Matthew G Lee
Sauhard Jain
Michael Xuelin Huang
Bjoern Hartmann
Can Liu
Proceedings of the 2024 CHI Conference on Human Factors in Computing Systems, Association for Computing Machinery, New York, NY, USA
Preview abstract
Dictation enables efficient text input on mobile devices. However, writing with speech can produce disfluent, wordy, and incoherent text and thus requires heavy post-processing. This paper presents Rambler, an LLM-powered graphical user interface that supports gist-level manipulation of dictated text with two main sets of functions: gist extraction and macro revision. Gist extraction generates keywords and summaries as anchors to support the review and interaction with spoken text. LLM-assisted macro revisions allow users to respeak, split, merge, and transform dictated text without specifying precise editing locations. Together they pave the way for interactive dictation and revision that help close gaps between spontaneously spoken words and well-structured writing. In a comparative study with 12 participants performing verbal composition tasks, Rambler outperformed the baseline of a speech-to-text editor + ChatGPT, as it better facilitates iterative revisions with enhanced user control over the content while supporting surprisingly diverse user strategies.
View details
Prompt-Based Label-Aware Framework for Few-Shot Multi-Label Text Classification
Thanakorn Thaminkaew
Peerapon Vateekul
IEEE Access, 12 (2024), pp. 28310-28322
Preview abstract
Prompt-based learning has demonstrated remarkable success in few-shot text classification, outperforming the traditional fine-tuning approach. This method transforms a text input into a masked language modeling prompt using a template, queries a fine-tuned language model to fill in the mask, and then uses a verbalizer to map the model’s output to a predicted class. Previous prompt-based text classification approaches were primarily designed for multi-class classification, taking advantage of the fact that the classes are mutually exclusive and one example belongs to only one class. However, these assumptions do not hold in the context of multi-label text classification, where labels often exhibit correlations with each other. Therefore, we propose a Prompt-based Label-Aware framework for Multi-Label text classification (PLAML) that addresses the challenges. Specifically, PLAML enhances prompt-based learning with three proposed techniques to improve the overall performance for multi-label classification. The techniques include (i) a token weighting algorithm that considers the correlations between labels, (ii) a template for augmenting training samples, making the training process label-aware, and (iii) a dynamic threshold mechanism, refining the prediction condition of each label. Extensive experiments on few-shot text classification across multiple datasets with various languages show that our PLAML outperforms other baseline methods. We also analyzed the effect of each proposed technique to better understand how it is suitable for the multi-label setting.
View details
Can Capacitive Touch Images Enhance Mobile Keyboard Decoding?
Billy Dou
Cedric Ho
Proceedings of UIST 2024 (2024)
Preview abstract
Capacitive touch sensors capture the two-dimensional spatial profile (referred to as a touch heatmap) of a finger's contact with a mobile touchscreen. However, the research and design of touchscreen mobile keyboards - one of the most speed- and accuracy-demanding touch interfaces - has focused on the location of the touch centroid derived from the touch image heatmap as the input, discarding the rest of the raw spatial signals. In this paper, we investigate whether touch heatmaps can be leveraged to further improve the tap decoding accuracy for mobile touchscreen keyboards. Specifically, we compared machine-learning models that decode user taps by using the centroids and/or the heatmaps as their input and studied the contribution due to the heatmap. The results show that adding the heatmap into the input feature set led to 21.4% relative reduction of character error rates on average, compared to using the centroid alone. Furthermore, we conducted online deployment testing of the heatmap-based decoder in a user study with 16 participants and observed lower error rate, faster typing speed, and higher self-reported satisfaction score based on the heatmap-based decoder than the centroid-based decoder. These findings underline the promise of utilizing touch heatmaps for improving typing experience in mobile keyboards.
View details
Meta Lifelong-Learning With Selective and Task-Aware Adaptation
Thanapapas Horsuwan
Kasidis Kanwatchara
Boonserm Kijsirikul
Peerapon Vateekul
IEEE Access, 12 (2024), pp. 34099-34115
Preview abstract
Meta-learning has been applied to lifelong language learning due to its ability to find an optimal model for efficient adaptation to any learned tasks. Generally, meta lifelong-learning partially stores samples from seen tasks in a memory and selects some of them to train the model, refresh the knowledge, and adapt the model for inference. However, the sample selection for these steps was usually done in a sub-optimal manner in existing work. Hence, we propose MeLSTA (Meta Lifelong-Learning with Selective and Task-Aware Adaptation) to effectively select the samples based on task identifiers and the adaptation scores reflecting the model behavior after adaptation. The results show that MeLSTA enhances the accuracy by 1.2% over the state-of-the-art while significantly shrinking the training duration by over 6 times. Additionally, our in-depth analysis reveals the strengths and limitations of MeLSTA and existing work, providing useful insights for future designs of meta lifelong-learning for NLP.
View details