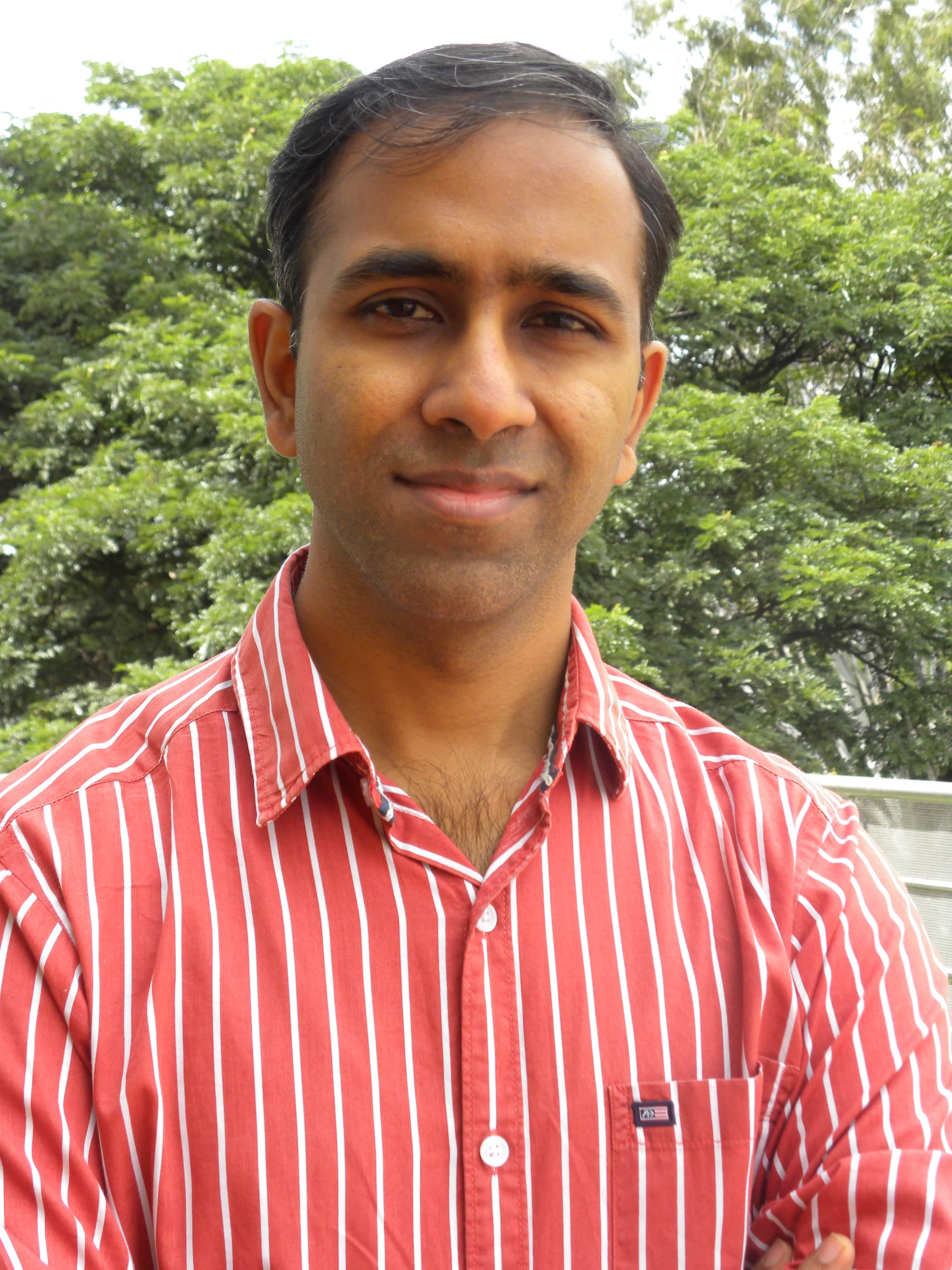
Prateek Jain
Prateek Jain is a research scientist at Google Research India and an adjunct faculty member at IIT Kanpur. Earlier, he was a Senior Principal Researcher at Microsoft Research India. He obtained his PhD degree from the Computer Science department at UT Austin and his BTech degree from IIT Kanpur. He works in the areas of large-scale and non-convex optimization, high-dimensional statistics, and ML for resource-constrained devices. He wrote a monograph on Non-convex Optimization in Machine Learning summarizing many of his results in non-convex optimization. Prateek regularly serves on the senior program committee of top ML conferences and is an action editor for JMLR, and an associate editor for SIMODS. His work has won ICML-2007, CVPR-2008 best student paper award and more recently his work on alternating minimization has been selected as the 2020 Best Paper by the IEEE Signal Processing Society.
Please visit prateekjain.org for my personal website.
Research Areas
Authored Publications
Sort By
Dual-Encoders for Extreme Multi-label Classification
Nilesh Gupta
Devvrit Khatri
Inderjit Dhillon
International Conference on Learning Representations (ICLR) (2024)
Preview abstract
Dual-encoder models have demonstrated significant success in dense retrieval tasks for open-domain question answering that mostly involves zero-shot and few-shot scenarios. However, their performance in many-shot retrieval problems, such as extreme classification, remains largely unexplored. State-of-the-art extreme classification techniques like NGAME use a combination of dual-encoders and a learnable classification head for each class to excel on these tasks. Existing empirical evidence shows that, for such problems, the dual-encoder method's accuracies lag behind the performance of the SOTA extreme classification methods that grow the number of learnable parameters with the number of classes. In this work, we investigate the potential reasons behind this observed gap, such as the intrinsic capacity limit due to fixed model size for dual-encoder models that is independent of the numbers of classes, training, loss formulation, negative sampling, etc. We methodically experiment on these different axes and find that model size is not the main bottleneck, but rather the training and loss formulation. When trained correctly even small dual-encoders can outperform State-of-the-art extreme classification methods by up to 2% at Precision on million label scale extreme classification datasets, while being 20x smaller in terms of the number of trainable parameters. We further propose a differentiable top-k error-based loss function, which can be used to specifically optimize for recall@k metrics.
View details
LEARNING AN INVERTIBLE OUTPUT MAPPING CAN MITIGATE SIMPLICITY BIAS IN NEURAL NETWORKS
Anshul Nasery
Sravanti Addepalli
Will be submitted to ICLR 2023 (2023) (to appear)
Preview abstract
Deep Neural Networks (DNNs) are known to be brittle to even minor distribution
shifts compared to the training distribution . Simplicity Bias (SB) of DNNs – bias
towards learning a small number of simplest features – has been demonstrated
to be a key reason for this brittleness. Prior works have shown that the effect of
Simplicity Bias is extreme – even when the features learned are diverse, training
the classification head again selects only few of the simplest features, leading to
similarly brittle models. In this work, we introduce Feature Reconstruction Regularizer
(FRR) in the linear classification head, with the aim of reducing Simplicity
Bias, thereby improving Out-Of-Distribution (OOD) robustness. The proposed
regularizer when used during linear layer training, termed as FRR-L, enforces that
the features can be reconstructed back from the logit layer, ensuring that diverse
features participate in the classification task. We further propose to finetune the
full network by freezing the weights of the linear layer trained using FRR-L. This
approach, termed as FRR-FLFT or Fixed Linear FineTuning, improves the quality
of the learned features, making them more suitable for the classification task.
Using this simple solution, we demonstrate up to 12% gain in accuracy on the
recently introduced synthetic datasets with extreme distribution shifts. Moreover,
on the standard OOD benchmarks recommended on DomainBed, our technique
can provide up to 5% gains over the existing SOTA methods .
View details
Preview abstract
We consider the problem of latent bandits with cluster structure where there are multiple users, each with an associated multi-armed bandit problem. These users are grouped into \emph{latent} clusters such that the mean reward vectors of users within the same cluster are identical. At each round, a user, selected uniformly at random, pulls an arm and observes a corresponding noisy reward. The goal of the users is to maximize their cumulative rewards. This problem is central to practical recommendation systems and has received wide attention of late \cite{gentile2014online, maillard2014latent}. Now, if each user acts independently, then they would have to explore each arm independently and a regret of $Ω(\sqrt{MNT})$ is unavoidable, where ?,? are the number of arms and users, respectively. Instead, we propose LATTICE (Latent bAndiTs via maTrIx ComplEtion) which allows exploitation of the latent cluster structure to provide the minimax optimal regret of $\tilde{O}(\sqrt{(M+N)T})$, when the number of clusters is $\tilde{O}(1)$. This is the first algorithm to guarantee such strong regret bound. LATTICE is based on a careful exploitation of arm information within a cluster while simultaneously clustering users. Furthermore, it is computationally efficient and requires only O(log?) calls to an offline matrix completion oracle across all ? rounds.
View details
Preview abstract
In this work, we consider the problem of collaborative multi-user reinforcement learning. In this setting there are multiple users with the same state-action space and transition probabilities but with different rewards. Under the assumption that the reward matrix of the N users has a low-rank structure -- a standard and practically successful assumption in the offline collaborative filtering setting-- the question is can we design algorithms with significantly lower sample complexity compared to the ones that learn the MDP individually for each user. Our main contribution is an algorithm which explores rewards collaboratively with N user-specific MDPs and can learn rewards efficiently in two key settings: tabular MDPs and linear MDPs. When N is large and the rank is constant, the sample complexity per MDP depends logarithmically over the size of the state-space, which represents an exponential reduction (in the state-space size) when compared to the standard ``non-collaborative'' algorithms.
View details
Preview abstract
We consider the problem of \emph{blocked} collaborative bandits where there are multiple users, each with an associated multi-armed bandit problem. These users are grouped into \emph{latent} clusters such that the mean reward vectors of users within the same cluster are identical. Our goal is to design algorithms that maximize the cumulative reward accrued by all the users over time, under the \emph{constraint} that no arm of a user is pulled more than ? times. This problem has been originally considered by \cite{Bresler:2014}, and designing regret-optimal algorithms for it has since remained an open problem. In this work, we propose an algorithm called \texttt{B-LATTICE} (Blocked Latent bAndiTs via maTrIx ComplEtion) that collaborates across users, while simultaneously satisfying the budget constraints, to maximize their cumulative rewards. Theoretically, under certain reasonable assumptions on the latent structure, with M users, N arms, T rounds per user, and C=O(1) latent clusters, \texttt{B-LATTICE} achieves a per-user regret of $\tilde{O}(\sqrt{T(1+N/M)})$ under a budget constraint of B=Θ(log T). These are the first sub-linear regret bounds for this problem, and match the minimax regret bounds when B=T. Empirically, we demonstrate that our algorithm has superior performance over baselines even when B=1. \texttt{B-LATTICE} runs in phases where in each phase it clusters users into groups and collaborates across users within a group to quickly learn their reward models.
View details
Domain-Agnostic Contrastive Representations for Learning from Label Proportions
Jay Nandy
Jatin Chauhan
Balaraman Ravindran
Proc. CIKM 2022
Preview abstract
We study the weak supervision learning problem of Learning from
Label Proportions (LLP) where the goal is to learn an instance-level
classifier using proportions of various class labels in a bag – a
collection of input instances that often can be highly correlated. While
representation learning for weakly-supervised tasks is found to
be effective, they often require domain knowledge. To the best of
our knowledge, representation learning for tabular data
(unstructured data containing both continuous and categorical
features) are not studied. In this paper, we propose to learn diverse
representations of instances within the same bags to effectively
utilize the weak bag-level supervision. We propose a domain
agnostic LLP method, called "Self Contrastive Representation
Learning for LLP" (SelfCLR-LLP) that incorporates a novel self–
contrastive function as an auxiliary loss to learn representations on
tabular data for LLP. We show that diverse representations for
instances within the same bags aid efficient usage of the weak bag-
level LLP supervision. We evaluate the proposed method through
extensive experiments on real-world LLP datasets from e-commerce
applications to demonstrate the effectiveness of our proposed
SelfCLR-LLP.
View details
Matryoshka Representation Learning
Aditya Kusupati
Gantavya Bhatt
Aniket Rege
Matthew Wallingford
Aditya Sinha
Vivek Ramanujan
William Howard-Snyder
Sham Kakade
Ali Farhadi
NeurIPS 2022 (2022)
Preview abstract
Learned representations are a central component in modern ML systems, serving a multitude of downstream tasks. When training such representations, it is often the case that computational and statistical constraints for each downstream task are unknown. In this context rigid, fixed capacity representations can be either over or under-accommodating to the task at hand. This leads us to ask: can we design a flexible representation that can adapt to multiple downstream tasks with varying computational resources? Our main contribution is Matryoshka Representation Learning (MRL) which encodes information at different granularities and allows a single embedding to adapt to the computational constraints of downstream tasks. MRL minimally modifies existing representation learning pipelines and imposes no additional cost during inference and deployment. MRL learns coarse-to-fine representations that are at least as accurate and rich as independently trained low-dimensional representations. The flexibility within the learned Matryoshka Representations offer: (a) up to 14x smaller embedding size for ImageNet-1K classification at the same level of accuracy; (b) up to 14x real-world speed-ups for large-scale retrieval on ImageNet-1K and 4K; and (c) up to 2% accuracy improvements for long-tail few-shot classification, all while being as robust as the original representations. Finally, we show that MRL extends seamlessly to web-scale datasets (ImageNet, JFT) across various modalities -- vision (ViT, ResNet), vision + language (ALIGN) and language (BERT). MRL code and pretrained models are open-sourced at https://github.com/RAIVNLab/MRL.
View details
Preview abstract
Q-learning is a popular Reinforcement Learning (RL) algorithm which is widely used in practice with function approximation \citep{mnih2015human}. In contrast, existing theoretical results are pessimistic about Q-learning. For example, \citep{baird1995residual} shows that Q-learning does not converge even with linear function approximation for linear MDPs. Furthermore, even for tabular MDPs with synchronous updates, Q-learning was shown to have sub-optimal sample complexity \citep{li2021q,azar2013minimax}. The goal of this work is to bridge the gap between practical success of Q-learning and the relatively pessimistic theoretical results. The starting point of our work is the observation that in practice, Q-learning is used with two important modifications: (i) training with two networks, called online network and target network simultaneously (online target learning, or OTL) , and (ii) experience replay (ER) \citep{mnih2015human}. While they have been observed to play a significant role in the practical success of Q-learning, a thorough theoretical understanding of how these two modifications improve the convergence behavior of Q-learning has been missing in literature. By carefully combining the Q-learning with OTL and \emph{reverse} experience replay (RER) (a form of experience replay), we present novel methods \qrex~and \qrexdr~(\qrex + data reuse). We show that \qrex~efficiently finds the optimal policy for linear MDPs and provide non-asymptotic bounds on sample complexity. Furthermore, we demonstrate that \qrexdr~in fact achieves near optimal sample complexity in the tabular setting, improving upon the existing results for vanilla Q-learning.
View details
Private Alternating Least Squares: (Nearly) OptimalPrivacy/Utility Trade-off for Matrix Completion
Abhradeep Guha Thakurta
Li Zhang
Walid Krichene
NA, NA (2021), NA
Preview abstract
We study the problem of differentially private (DP) matrix completion under user-level privacy. We design an $(\epsilon,\delta)$-joint differentially private variant of the popular Alternating-Least-Squares (ALS) method that achieves: i) (nearly) optimal sample complexity for matrix completion (in terms of number of items, users), and ii) best known privacy/utility trade-off both theoretically, as well as on benchmark data sets.
In particular, despite non-convexity of low-rank matrix completion and ALS, we provide the first global convergence analysis of ALS with {\em noise} introduced to ensure DP. For $n$ being the number of users and $m$ being the number of items in the rating matrix, our analysis requires only about $\log (n+m)$ samples per user (ignoring rank, condition number factors) and obtains a sample complexity of $n=\tilde\Omega(m/(\sqrt{\zeta}\cdot \epsilon))$ to ensure relative Frobenius norm error of $\zeta$. This improves significantly on the previous state of the result of $n=\tilde\Omega\left(m^{5/4}/(\zeta^{5}\epsilon)\right)$ for the private-FW method by ~\citet{jain2018differentially}.
Furthermore, we extensively validate our method on synthetic and benchmark data sets (MovieLens 10mi, MovieLens 20mi), and observe that private ALS only suffers a 6 percentage drop in accuracy when compared to the non-private baseline for $\epsilon\leq 10$. Furthermore, compared to prior work of~\cite{jain2018differentially}, it is at least better by 10 percentage for all choice of the privacy parameters.
View details
Preview abstract
We study personalization of supervised learning with user-level differential privacy. Consider a setting with many users, each of whom has a training data set drawn from their own distribution $P_i$. Assuming some shared structure among the problems $P_i$, can users collectively learn the shared structure---and solve their tasks better than they could individually---while preserving the privacy of their data? We formulate this question using joint, \textit{user-level} differential privacy---that is, we control what is leaked about each user's entire data set.
We provide algorithms that exploit popular non-private approaches in this domain like the Almost-No-Inner-Loop (ANIL) method, and give strong user-level privacy guarantees for our general approach. When the problems $P_i$ are linear regression problems with each user's regression vector lying in a common, unknown low-dimensional subspace, we show that our efficient algorithms satisfy nearly optimal estimation error guarantees. We also establish a general, information-theoretic upper bound via an exponential mechanism-based algorithm.
Finally, we demonstrate empirically (through experiments on synthetic data sets) that our framework not only performs well in the studied linear regression setting, but also extends to other settings like logistic regression that are not captured by our estimation error analysis.
View details