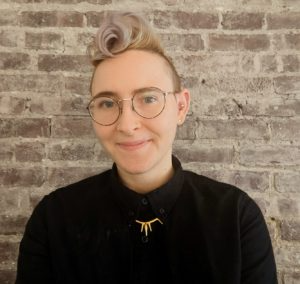
Remi Denton
Research Areas
Authored Publications
Sort By
“They only care to show us the wheelchair”: disability representation in text-to-image AI models
Avery Mack
Rida Qadri
CHI Conference on Human-Computer Interaction (2024)
Preview abstract
This paper reports on disability representation in images output from text-to-image (T2I) generative AI systems. Through eight focus groups with 25 people
with disabilities, we found that models repeatedly presented reductive archetypes for different disabilities. Often these representations reflected broader
societal stereotypes and biases, which our participants were concerned to see reproduced through T2I. Our participants discussed further challenges with
using these models including the current reliance on prompt engineering to reach satisfactorily diverse results. Finally, they offered suggestions for
how to improve disability representation with solutions like showing multiple, heterogeneous images for a single prompt and including the prompt with images
generated. Our discussion reflects on tensions and tradeoffs we found among the diverse perspectives shared to inform future research on representation-oriented
generative AI system evaluation metrics and development processes.
View details
ViSAGe: A Global-Scale Analysis of Visual Stereotypes in Text-to-Image Generation
Akshita Jha
Sarah Laszlo
Rida Qadri
Chandan Reddy
ACL (2024)
Preview abstract
Recent studies have highlighted the issue of varying degrees of stereotypical depictions for different identity group. However, these existing approaches have several key limitations, including a noticeable lack of coverage of identity groups in their evaluation, and the range of their associated stereotypes. Additionally, these studies often lack a critical distinction between inherently visual stereotypes, such as `brown' or `sombrero', and culturally influenced stereotypes like `kind' or `intelligent'. In this work, we address these limitations by grounding our evaluation of regional, geo-cultural stereotypes in the generated images from Text-to-Image models by leveraging existing textual resources. We employ existing stereotype benchmarks to evaluate stereotypes and focus exclusively on the identification of visual stereotypes within the generated images spanning 135 identity groups. We also compute the offensiveness across identity groups, and check the feasibility of identifying stereotypes automatically. Further, through a detailed case study and quantitative analysis, we reveal how the default representations of all identity groups have a more stereotypical appearance, and for historically marginalized groups, how the images across different attributes are visually more similar than other groups, even when explicitly prompted otherwise.
View details
Towards Globally Responsible Generative AI Benchmarks
Rida Qadri
ICLR Workshop : Practical ML for Developing Countries Workshop (2023)
Preview abstract
As generative AI globalizes, there is an opportunity to reorient our nascent development frameworks and evaluative practices towards a global context. This paper uses lessons from a community-centered study on the failure modes of text to Image models in the South Asian context, to give suggestions on how the AI/ML community can develop culturally and contextually situated benchmarks. We present three forms of mitigations for culturally situated- evaluations: 1) diversifying our diversity measures 2) participatory prompt dataset curation 2) multi-tiered evaluations structures for community engagement. Through these mitigations we present concrete methods to make our evaluation processes more holistic and human-centered while also engaging with demands of deployment at global scale.
View details
"I wouldn’t say offensive but...": Disability-Centered Perspectives on Large Language Models
Vinitha Gadiraju
Alex Taylor
Robin Brewer
Proceedings of FAccT 2023 (2023) (to appear)
Preview abstract
Large language models (LLMs) trained on real-world data can inadvertently reflect harmful societal biases, particularly toward historically marginalized communities. While previous work has primarily focused on harms related to age and race, emerging research has shown that biases toward disabled communities exist. This study extends prior work exploring the existence of harms by identifying categories of LLM-perpetuated harms toward the disability community. We conducted 19 focus groups, during which 56 participants with disabilities probed a dialog model about disability and discussed and annotated its responses. Participants rarely characterized model outputs as blatantly offensive or toxic. Instead, participants used nuanced language to detail how the dialog model mirrored subtle yet harmful stereotypes they encountered in their lives and dominant media, e.g., inspiration porn and able-bodied saviors. Participants often implicated training data as a cause for these stereotypes and recommended training the model on diverse identities from disability-positive resources. Our discussion further explores representative data strategies to mitigate harm related to different communities through annotation co-design with ML researchers and developers.
View details
AI’s Regimes of Representation: A Community-centered Study of Text-to-Image Models in South Asia
Rida Qadri
Proceedings of the 2023 ACM Conference on Fairness, Accountability, and Transparency, Association for Computing Machinery, 506–517
Preview abstract
This paper presents a community-centered study of cultural limitations of text-to-image (T2I) models in the South Asian context. We theorize these failures using scholarship on dominant media regimes of representations and locate them within participants’ reporting of their existing social marginalizations. We thus show how generative AI can reproduce an outsiders gaze for viewing South Asian cultures, shaped by global and regional power inequities. By centering communities as experts and soliciting their perspectives on T2I limitations, our study adds rich nuance into existing evaluative frameworks and deepens our understanding of the culturally-specific ways AI technologies can fail in non-Western and Global South settings. We distill lessons for responsible development of T2I models, recommending concrete pathways forward that can allow for recognition of structural inequalities.
View details
CrowdWorkSheets: Accounting for Individual and Collective Identities Underlying Crowdsourced Dataset Annotation
Ian Kivlichan
Dylan Baker
Razvan Adrian Amironesei
Association for Computing Machinery, 2342–2351
Preview abstract
Human annotated data plays a crucial role in machine learning (ML) research and development. However, the ethical considerations around the processes and decisions that go into dataset annotation have not received nearly enough attention. In this paper, we survey an array of literature that provides insights into ethical considerations around crowdsourced dataset annotation. We synthesize these insights, and lay out the challenges in this space along two layers: (1) who the annotator is, and how the annotators' lived experiences can impact their annotations, and (2) the relationship between the annotators and the crowdsourcing platforms, and what that relationship affords them. Finally, we introduce a novel framework, CrowdWorkSheets, for dataset developers to facilitate transparent documentation of key decisions points at various stages of the data annotation pipeline: task formulation, selection of annotators, platform and infrastructure choices, dataset analysis and evaluation, and dataset release and maintenance.
View details
Preview abstract
In response to growing concerns of bias, discrimination, and unfairness perpetuated by algorithmic systems, the datasets used to train and evaluate machine learning models have come under increased scrutiny. Many of these examinations have focused on the contents of machine learning datasets, finding glaring underrepresentation of minoritized groups. In contrast, relatively little work has been done to examine the norms, values, and assumptions embedded in these datasets. In this work, we conceptualize machine learning datasets as a type of informational infrastructure, and motivate a genealogy as method in examining the histories and modes of constitution at play in their creation. We present a critical history of ImageNet as an exemplar, utilizing critical discourse analysis of major texts around ImageNet’s creation and impact. We find that assumptions around ImageNet and other large computer vision datasets more generally rely on three themes: the aggregation and accumulation of more data, the computational construction of meaning, and making certain types of data labor invisible. By tracing the discourses that surround this influential benchmark, we contribute to the ongoing development of the standards and norms around data development in machine learning and artificial intelligence research.
View details
Preview abstract
Datasets form the basis for training, evaluating, and benchmarking machine learning models and have played a foundational role in the advancement of the field. Furthermore, the ways in which we collect, construct, and share these datasets inform the kinds of problems the field pursues and the methods explored in algorithm development. In this work, we survey recent issues pertaining to data in machine learning research, focusing primarily on work in computer vision and natural language processing. We summarize concerns relating to the design, collection, maintenance, distribution, and use of machine learning datasets as well as broader disciplinary norms and cultures that pervade the field. We advocate a turn in the culture toward more careful practices of development, maintenance, and distribution of datasets that are attentive to limitations and societal impact while respecting the intellectual property and privacy rights of data creators and data subjects.
View details
Art Sheets for Art Datasets
Ramya Malur Srinivasan
Jordan Jennifer Famularo
Beth Coleman
NeurIPS Dataset & Benchmark track (2021)
Preview abstract
As machine learning (ML) techniques are being employed to authenticate artworks and estimate their market value, computational tasks have expanded across a variety of creative domains and datasets drawn from the arts. With recent progress in generative modeling, ML techniques are also used for simulating artistic styles and for producing new content in various media such as music, visual arts, poetry, etc. While this progress has opened up new creative avenues, it has also paved the way for adverse downstream effects such as cultural appropriation (e.g., cultural misrepresentation, offense, and undervaluing) and amplification of gender and racial stereotypes, to name a few. Many such concerning issues stem from the training data in ways that diligent evaluation can uncover, prevent, and mitigate. In this paper, we provide a checklist of questions customized for use with art datasets, building on the questionnaire for datasets provided in Datasheets, by guiding assessment of developer motivation together with dataset provenance, composition, collection, pre-processing, cleaning, labeling, use (including data generation/synthesis), distribution, and maintenance. Case studies exemplify the value of our questionnaire. We hope our work aids ML scientists and developers by providing a framework for responsible design, development, and use of art datasets.
View details
Towards Accountability for Machine Learning Datasets
Alex Hanna
Christina Greer
Margaret Mitchell
Proceedings of FAccT 2021 (2021) (to appear)
Preview abstract
Rising concern for the societal implications of artificial intelligence systems has inspired demands for greater transparency and accountability. However the datasets which empower machine learning are often used, shared and re-used with little visibility into the processes of deliberation which led to their creation. Which stakeholder groups had their perspectives included when the dataset was conceived? Which domain experts were consulted regarding how to model subgroups and other phenomena? How were questions of representational biases measured and addressed? Who labeled the data? In this paper, we introduce a rigorous framework for dataset development transparency which supports decision-making and accountability. The framework uses the cyclical, infrastructural and engineering nature of dataset development to draw on best practices from the software development lifecycle. Each stage of the data development lifecycle yields a set of documents that facilitate improved communication and decision-making, as well as drawing attention the value and necessity of careful data work. The proposed framework is intended to contribute to closing the accountability gap in artificial intelligence systems, by making visible the often overlooked work that goes into dataset creation.
View details