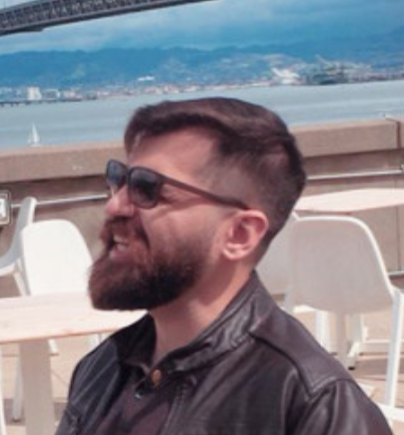
Sasan Tavakkol
Authored Publications
Sort By
Data Sampling using Locality Sensitive Hashing for Large Scale Graph Learning
Mohamed Farghal
Animesh Nandi
Sarath Shekkizhar
2023
Preview abstract
An important step in graph-based data analysis and processing is the construction of similarity graphs. Recent works have focused on the semi-supervised setting to learn an optimal similarity function for constructing a task-optimal graph. However, in many scenarios with billions of data points and trillions of potential edges, the run-time and computational requirements for training the similarity model make these approaches impractical.
In this work, we consider data sampling as a means to overcome this issue. Unlike typical sampling use-cases which only seek diversity, the similarity-learning for graph construction problem requires data samples that are both diverse and representative of highly similar data points. We present an efficient sampling approach by taking an adaptive partition view of locality sensitive hashing. Theoretically, we show that, though the samples obtained are correlated with sampling probabilities that do not sum to one, the training loss estimated for learning the graph similarity model using our approach is unbiased with a smaller variance compared to random sampling. Experiments on public datasets demonstrate the superior generalization of similarity models learned via our sampling. In a real large-scale industrial abuse-detection example, we observe ≈10× increase in identifying abusive items while having lower false positive rate compared to the baseline.
View details
Two-step Active Learning for Instance Segmentation Neural Networks
Ke Yu
Suraj Kothawade
Abdullah Rashwan
Kayhan Batmanghelich
Xiaoqi(Michael) Yin
2023
Preview abstract
Training high quality instance segmentation models re-quires an abundance of labeled images containing instancemasks and classifications. Such data is often expensive toprocure. Active learning aims to achieve high performanceat minimal labeling cost by sampling only the most infor-mative and representative images to send for labeling. Ac-tive learning has been less explored for the task of instancesegmentation, than for other, less labeling intensive taskslike image classification. In this work, we propose a sim-ple, easy-to-implement, uncertainty based sampling strat-egy for instance segmentation, and an improvement whichadditionally incorporates diversity considerations.
View details
Kartta Labs: Collaborative Time Travel
Feng Han
Mark Phillips
Cyrus Shahabi
Yao-Yi Chiang
Raimondas Kiveris
2021
Preview abstract
We introduce the modular and scalable design of Kartta Labs, an open source, open data, and scalable system for virtually reconstructing cities from historical maps and photos. Kartta Labs relies on crowdsourcing and artificial intelligence consisting of two major modules: Maps and 3D models. Each module, in turn, consists of sub-modules that enable the system to reconstruct a city from historical maps and photos. The result is a spatiotemporal reference that can be used to integrate various collected data (curated, sensed, or crowdsourced) for research, education, and entertainment purposes. The system empowers the users to experience collaborative time travel such that they work together to reconstruct the past and experience it on an open source and open data platform.
View details
Piaget: A Probabilistic Inference Approach for Geolocating Historical Buildings
Cyrus Shahabi
Feng Han
Raimondas Kiveris
IEEE International Conference on Big Data (2021)
Preview abstract
We aim to find the geographical location of (geolocate) a large number of old buildings facades extracted from historical photographs. We can acquire the geo-coordinates of some of these facades either through crowdsourcing or exploring their metadata. Using these “seed” buildings and through spatial reasoning within and across the historical pictures, in this paper, we show how we infer the geolocation of the other facades. We propose a probabilistic inference approach that first constructs a graph with facades as nodes and their spatial distances as edges, and then through probabilistic inference on this graph, geolocate the facades. Our experiments show that with 10\% of the building geolocated as seed buildings, we can quite accurately geolocate the rest of the buildings in our dataset.
View details
Preview abstract
We develop the third-order adaptive Adams-Bashforth time integration and the second-order finite difference equation for variable time steps. We incorporate these schemes in the Celeris Advent software to discretize and solve the 2D extended Boussinesq equations. This software uses a hybrid finite volume – finite difference scheme and leverages the GPU to solve the equations faster than real-time while concurrently visualizing them. The newly added adaptive scheme significantly improves the robustness of the model while providing faster computational performance. We simulate several benchmarks using the adaptive time stepping scheme of Celeris Advent and demonstrate the capability of the software in modeling wave-breaking, wave runup, irregular waves, and rip currents.
View details
An Automatic Approach for Generating Rich, Linked Geo-Metadata from Historical Map Images
Zekun Li
Yao-Yi Chiang
Basel Shbita
Johannes H. Uhl
Stefan Leyk
Craig Knoblock
ACM SIGKDD International Conference on Knowledge Discovery and Data Mining (2020)
Preview abstract
Historical maps contain detailed geographic information difficult to find elsewhere covering long-periods of time (e.g., 125 years for the historical topographic maps in the US). However, these maps typically exist as scanned images without searchable metadata. Existing approaches making historical maps searchable rely on tedious manual work (including crowd-sourcing) to generate the metadata (e.g., geolocations and keywords). Optical character recognition (OCR) software could alleviate the required manual work, but the recognition results are individual words instead of location phrases (e.g., ``Black'' and ``Mountain'' vs. ``Black Mountain''). This paper presents an end-to-end approach to address the real-world problem of finding and indexing historical map images. This approach automatically processes historical map images to extract their text content and generates a set of metadata that is linked to large external geospatial knowledge bases. The linked metadata in the RDF (Resource Description Framework) format support complex queries for finding and indexing historical maps, such as retrieving all historical maps covering mountain peaks higher than 1,000 meters in California. We have implemented the approach in a system called mapKurator. We have evaluated mapKurator using historical maps from several sources with various map styles, scales, and coverage. Our results show significant improvement over the state-of-the-art methods. The code has been made publicly available as modules of the Kartta Labs project at https://github.com/kartta-labs/Project.
View details
Preview abstract
We introduce our interactive and immersive coastal wave simulation software, Celeris Base, which is the successor to Celeris Advent. Celeris Base is an open source software developed in the Unity3D game engine and in C# language. It supports an interactive environment and allows users to view the simulations in a virtual reality headset. Celeris Base solves the same equations as Celeris Advent, the extended Boussinesq equations, using our hybrid finite volume–finite difference method. These equations are solved on the GPU using compute shaders, written in HLSL. Celeris Base has several new features such as 360◦ video capturing, geographic map overlays, built-in real-time gauge plotters, etc. It also improves the implementation of the sponge layer boundary condition by introducing new damping equations. Celeris Base is designed and implemented using the best software engineering practices in the hope that it will be a base for further developments of the Celeris software series by researchers around the globe. We validate Celeris Base against experimental results in this paper.
View details
Kartta Labs: Unrendering Historical Maps
Yao-Yi Chiang
Tim Waters
Feng Han
Kisalaya Prasad
Raimondas Kiveris
Proceedings of the 3nd ACM SIGSPATIAL International Workshop on AI for Geographic Knowledge Discovery. ACM, 2019. (2019)
Preview abstract
This paper introduces the Kartta Labs, an ongoing open-source and open-data project aiming for organizing the world’s historical maps and making them universally accessible and useful. Kartta Labs’ framework is designed as a composition of multiple modules. Each module includes a crowdsourcing component and an intelligent, machine-assisted component to automate the process. The framework takes images of historical maps, registers them in space and time, generates a vector version of the map content, and allows the users to query for the vector content and recreate the historical maps in various cartographic styles. We refer to this process as unrendering. The resulting machine-readable map data support a variety of scientific studies and applications that require long-term, detailed geographic information in the past and open up opportunities in other areas such as entertainment. The paper also presents the preliminary results from one automated module to geolocalize a guven a historical map.
View details