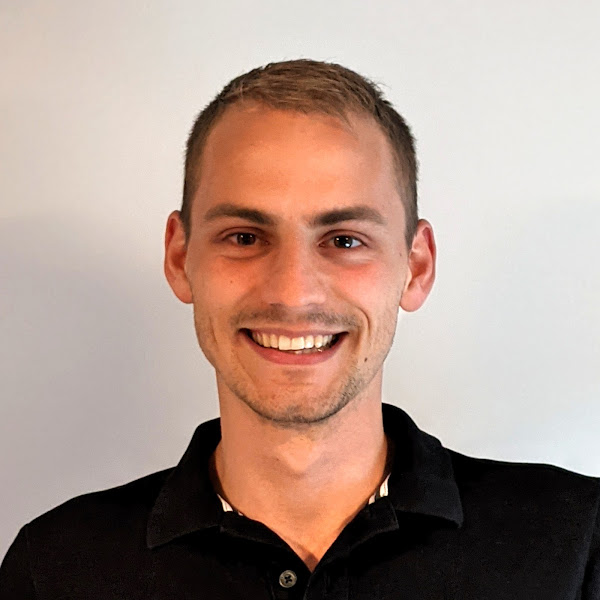
Timo Denk
Research Areas
Authored Publications
Sort By
V2Meow: Meowing to the Visual Beat via Video-to-Music Generation
Chris Donahue
Dima Kuzmin
Joonseok Lee
Judith Li
Kun Su
Mauro Verzetti
Qingqing Huang
Yu Wang
Vol. 38 No. 5: AAAI-24 Technical Tracks 5, AAAI Press (2024), pp. 4952-4960
Preview abstract
Video-to-music generation demands both a temporally localized high-quality listening experience and globally aligned video-acoustic signatures. While recent music generation models excel at the former through advanced audio codecs, the exploration of video-acoustic signatures has been confined to specific visual scenarios. In contrast, our research confronts the challenge of learning globally aligned signatures between video and music directly from paired music and videos, without explicitly modeling domain-specific rhythmic or semantic relationships. We propose V2Meow, a video-to-music generation system capable of producing high-quality music audio for a diverse range of video input types using a multi-stage autoregressive model. Trained on 5k hours of music audio clips paired with video frames mined from in-the-wild music videos, V2Meow is competitive with previous domain-specific models when evaluated in a zero-shot manner. It synthesizes high-fidelity music audio waveforms solely by conditioning on pre-trained general purpose visual features extracted from video frames, with optional style control via text prompts. Through both qualitative and quantitative evaluations, we demonstrate that our model outperforms various existing music generation systems in terms of visual-audio correspondence and audio quality. Music samples are available at tinyurl.com/v2meow.
View details
Noise2Music: Text-conditioned Music Generation with Diffusion Models
Qingqing Huang
Daniel S. Park
Tao Wang
Nanxin Chen
Zhengdong Zhang
Zhishuai Zhang
Jiahui Yu
Christian Frank
William Chan
Zhifeng Chen
Wei Han
(2023)
Preview abstract
We introduce Noise2Music, where a series of diffusion models are trained to generate high-quality 30-second music clips from text prompts. Two types of diffusion models, a generator model, which generates an intermediate representation conditioned on text, and a cascader model, which generates high-fidelity audio conditioned on the intermediate representation and possibly the text, are trained and utilized in succession to generate high-fidelity music. We explore two options for the intermediate representation, one in which it is a spectrogram and the other in which it is audio with lower fidelity. We find that the generated audio is able to faithfully reflect key elements of the text prompt such as genre, mood, tempo and instruments. Language models play a key role in this story---they are used to generate paired text for the audio of the training set and to extract embeddings of the text prompts ingested by the diffusion models.
View details
Brain2Music: Reconstructing Music from Human Brain Activity
Yu Takagi
Takuya Matsuyama
Andrea Agostinelli
Tomoya Nakai
Christian Frank
Shinji Nishimoto
(2023)
Preview abstract
The process of reconstructing experiences from human brain activity offers a unique lens into how the brain interprets and represents the world. In this paper, we introduce a method for reconstructing music from brain activity, captured using functional magnetic resonance imaging (fMRI). Our approach uses either music retrieval or the MusicLM music generation model conditioned on embeddings derived from fMRI data. The generated music resembles the musical stimuli that human subjects experienced, with respect to semantic properties like genre, instrumentation, and mood. We investigate the relationship between different components of MusicLM and brain activity through a voxel-wise encoding modeling analysis. Furthermore, we discuss which brain regions represent information derived from purely textual descriptions of music stimuli. We provide supplementary material including examples of the reconstructed music at https://google-research.github.io/seanet/brain2music
View details
MusicLM: Generating Music From Text
Andrea Agostinelli
Zalán Borsos
Mauro Verzetti
Antoine Caillon
Qingqing Huang
Marco Tagliasacchi
Matt Sharifi
Neil Zeghidour
Christian Frank
under review (2023)
Preview abstract
We introduce MusicLM, a model generating high-fidelity music from text descriptions such as "a calming violin melody backed by a distorted guitar riff". MusicLM casts the process of conditional music generation as a hierarchical sequence-to-sequence modeling task, and it generates music at 24 kHz that remains consistent over several minutes. Our experiments show that MusicLM outperforms previous systems both in audio quality and adherence to the text description. Moreover, we demonstrate that MusicLM can be conditioned on both text and a melody in that it can transform whistled and hummed melodies according to the style described in a text caption. To support future research, we publicly release MusicCaps, a dataset composed of 5.5k music-text pairs, with rich text descriptions provided by human experts.
Further links: samples, MusicCaps dataset
View details