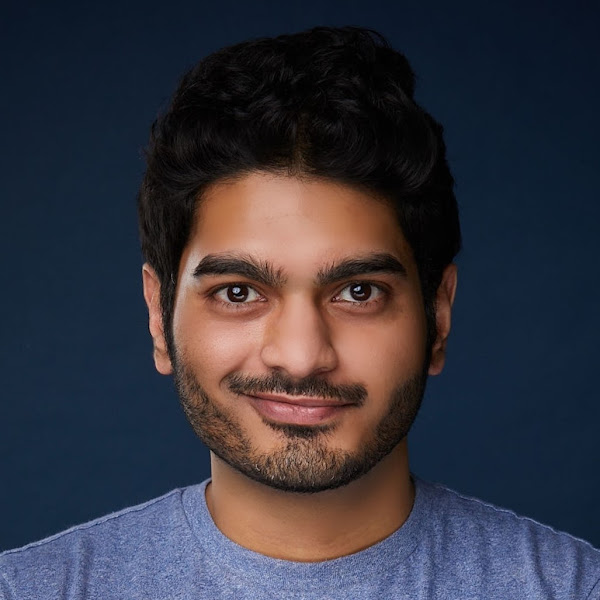
Vedant Misra
I work on studying and extending the capabilities of large neural networks with the goal of understanding the computational principles of intelligence. I previously oversaw the Algorithms and Reasoning teams at OpenAI. Earlier, I was founder and CEO of Kemvi (acquired by HubSpot), and led the ML Labs team at HubSpot, focused on developing new machine learning products with language.
Research Areas
Authored Publications
Sort By
Exploring Length Generalization in Large Language Models
Cem Anil
Yuhuai Wu
Aitor Lewkowycz
Guy Gur-Ari
NeurIPS Oral (2022)
Preview abstract
The ability to extrapolate from short problem instances to longer ones is an important form of out-of-distribution generalization in reasoning tasks, and is crucial when learning from datasets where longer problem instances are rare. These include theorem proving, solving quantitative mathematics problems, and reading/summarizing novels. In this paper, we run careful empirical studies exploring the length generalization capabilities of transformer-based language models. We first establish that naively finetuning transformers on length generalization tasks shows significant generalization deficiencies independent of model scale. We then show that combining pretrained large language models' in-context learning abilities with scratchpad prompting (asking the model to output solution steps before producing an answer) results in a dramatic improvement in length generalization. We run careful failure analyses on each of the learning modalities and identify common sources of mistakes that highlight opportunities in equipping language models with the ability to generalize to longer problems.
View details
Beyond the Imitation Game: Quantifying and extrapolating the capabilities of language models
Aitor Lewkowycz
Daniel Freeman
Guy Gur-Ari
Jaehoon Lee
Jascha Sohl-dickstein
Liam B. Fedus
TBD (2022)
Preview abstract
Language models demonstrate both quantitative improvement and new qualitative capabilities with increasing scale. Despite their potentially transformative impact, these new capabilities are as yet poorly characterized. In order to direct future research, prepare for disruptive new model capabilities, and ameliorate socially harmful effects, it is vital that we understand the present and near-future capabilities and limitations of language models.
To address this challenge, we introduce the Beyond the Imitation Game benchmark (BIG-bench). BIG-bench consists of 207 tasks, contributed by over 400 authors across 132 institutions. Task topics are diverse, drawing problems from linguistics, childhood development, math, common sense reasoning, biology, physics, social bias, software development, and beyond. BIG-bench focuses on capabilities that are believed to be beyond current language models. We evaluate the behavior of OpenAI's GPT models, Google-internal dense transformer architectures, and Switch-style sparse transformers on BIG-bench, across model sizes spanning millions to hundreds of billions of parameters. A team of human experts further performed all tasks, to provide a strong baseline. Findings include: model performance and calibration both improve with scale, but are poor in absolute terms (and when compared with human performance); model performance is remarkably similar across model classes; tasks that improve gradually and predictably commonly involve a large knowledge or memorization component, whereas tasks that exhibit ``breakthrough'' behavior at a critical scale often involve a significant reasoning or algorithmic component; social bias typically increases with scale in settings with ambiguous context, but this can be improved with prompting.
View details
PaLM: Scaling Language Modeling with Pathways
Aakanksha Chowdhery
Sharan Narang
Jacob Devlin
Maarten Bosma
Hyung Won Chung
Sebastian Gehrmann
Parker Schuh
Sasha Tsvyashchenko
Abhishek Rao
Yi Tay
Noam Shazeer
Nan Du
Reiner Pope
James Bradbury
Guy Gur-Ari
Toju Duke
Henryk Michalewski
Xavier Garcia
Liam Fedus
David Luan
Barret Zoph
Ryan Sepassi
David Dohan
Shivani Agrawal
Mark Omernick
Marie Pellat
Aitor Lewkowycz
Erica Moreira
Rewon Child
Oleksandr Polozov
Zongwei Zhou
Brennan Saeta
Michele Catasta
Jason Wei
arxiv:2204.02311 (2022)
Preview abstract
Large language models have been shown to achieve remarkable performance across a variety of natural language tasks using few-shot learning, which drastically reduces the number of task-specific training examples needed to adapt the model to a particular application. To further our understanding of the impact of scale on few-shot learning, we trained a 540-billion parameter, densely activated, Transformer language model, which we call Pathways Language Model PaLM. We trained PaLM on 6144 TPU v4 chips using Pathways, a new ML system which enables highly efficient training across multiple TPU Pods. We demonstrate continued benefits of scaling by achieving state-of-the-art few-shot learning results on hundreds of language understanding and generation benchmarks. On a number of these tasks, PaLM 540B achieves breakthrough performance, outperforming the finetuned state-of-the-art on a suite of multi-step reasoning tasks, and outperforming average human performance on the recently released BIG-bench benchmark. A significant number of BIG-bench tasks showed discontinuous improvements from model scale, meaning that performance steeply increased as we scaled to our largest model. PaLM also has strong capabilities in multilingual tasks and source code generation, which we demonstrate on a wide array of benchmarks. We additionally provide a comprehensive analysis on bias and toxicity, and study the extent of training data memorization with respect to model scale. Finally, we discuss the ethical considerations related to large language models and discuss potential mitigation strategies.
View details
Solving Quantitative Reasoning Problems with Language Models
Aitor Lewkowycz
David Martin Dohan
Henryk Michalewski
Cem Anil
Imanol Schlag
Theo Gutman-Solo
Yuhuai Wu
Guy Gur-Ari
NeurIPS (2022)
Preview abstract
Language models have achieved remarkable performance on a wide range of tasks that require natural language understanding. Nevertheless, state-of-the-art models have generally struggled with tasks that require quantitative reasoning, such as solving mathematics, science, and engineering problems at the college level. To help close this gap, we introduce Minerva, a large language model pretrained on general natural language data and further trained on technical content. The model achieves state-of-the-art performance on technical benchmarks without the use of external tools. We also evaluate our model on over two hundred undergraduate-level problems in physics, biology, chemistry, economics, and other sciences that require quantitative reasoning, and find that the model can correctly answer nearly a third of them.
View details