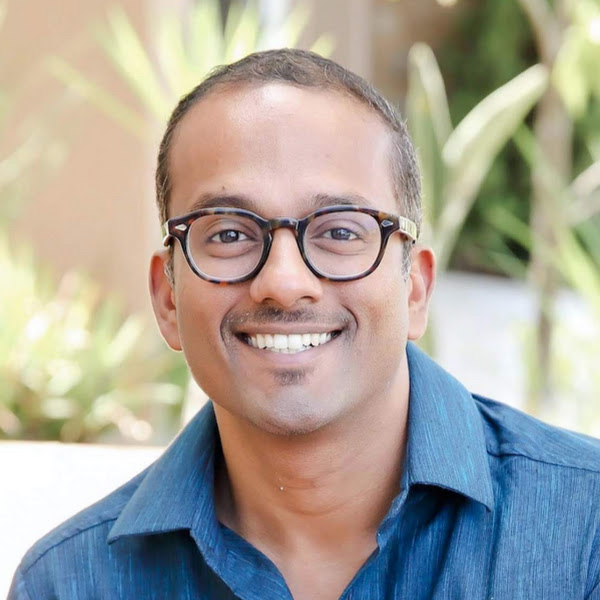
Vinodkumar Prabhakaran
Vinodkumar Prabhakaran is a Research Scientist at Google, working on issues around Ethical AI and ML Fairness. Prior to this, he was a postdoctoral researcher in the Computer Science department at Stanford University, where he worked with Prof. Dan Jurafsky and others at the Stanford NLP group, in an array of projects with a focus on applying Artificial Intelligence for Social Good. He obtained his PhD in computer science from Columbia University in 2015. His research brings together natural language processing techniques, machine learning algorithms, and social science methods to build scalable ways to identify and address large-scale societal issues such as racial disparities in policing, workplace incivility, gender bias and stereotypes, and abusive behavior online.
Authored Publications
Sort By
Beyond Aesthetics: Cultural Competence in Text-to-Image Models
Nithish Kannen
Marco Andreetto
Adji Bousso Dieng
2024
Preview abstract
Use of Text-to-Image models is expanding beyond generating generic objects, as they are increasingly being adopted by diverse global communities to create visual representations of their unique culture. Current T2I benchmarks primarily evaluate image-text alignment, aesthetics and fidelity of generations for complex prompts with generic objects, overlooking the critical dimension of cultural understanding. In this work, we address this gap by defining a framework to evaluate cultural competence of T2I models, and present a scalable approach to collect cultural artifacts unique to a particular culture from Knowledge Graphs and Large Language Models in tandem. We assess the ability of state-of-the-art T2I models to generate culturally faithful and realistic images across 8 countries and 3 cultural domains. Furthermore, we emphasize the importance of T2I models reflecting a culture's diversity and introduce cultural diversity as a novel metric for T2I evaluation, drawing inspiration from the Vendi Score. We introduce T2I-GCube, a first-of-its-kind benchmark for T2I evaluation. T2I-GCube includes cultural prompts, metrics, and cultural concept spaces, enabling comprehensive assessment of T2I models' cultural knowledge and diversity. Our evaluations reveal significant gaps in the cultural knowledge of existing models and provide valuable insights into the diversity of image outputs for under-specified prompts. By introducing a novel approach to evaluating cultural diversity and knowledge in T2I models, T2I-GCube will be instrumental in fostering the development of models with enhanced cultural competence.
View details
Preview abstract
While large, generative, multilingual models are rapidly being developed and deployed, their safety and fairness evaluations primarily hinge on resources collected in the English language and some limited translations. This has been demonstrated to be insufficient, and severely lacking in nuances of unsafe language and stereotypes prevalent in different languages and the geographical pockets they are prevalent in. Gathering these resources, at scale, in varied languages and regions also poses a challenge as it requires expansive sociolinguistic knowledge and can also be prohibitively expensive. We utilize an established methodology of coupling LLM generations with distributed annotations to overcome these gaps and create the resource SeeGULL Multilingual, spanning 20 languages across 23 regions.
View details
Beyond Aesthetics: Cultural Competence in Text-to-Image Models
Nithish Kannen
Marco Andreetto
Adji Bousso Dieng
2024
Preview abstract
Use of Text-to-Image models is expanding beyond generating generic objects, as they are increasingly being adopted by diverse global communities to create visual representations of their unique culture. Current T2I benchmarks primarily evaluate image-text alignment, aesthetics and fidelity of generations for complex prompts with generic objects, overlooking the critical dimension of cultural understanding. In this work, we address this gap by defining a framework to evaluate cultural competence of T2I models, and present a scalable approach to collect cultural artifacts unique to a particular culture from Knowledge Graphs and Large Language Models in tandem. We assess the ability of state-of-the-art T2I models to generate culturally faithful and realistic images across 8 countries and 3 cultural domains. Furthermore, we emphasize the importance of T2I models reflecting a culture's diversity and introduce cultural diversity as a novel metric for T2I evaluation, drawing inspiration from the Vendi Score. We introduce T2I-GCube, a first-of-its-kind benchmark for T2I evaluation. T2I-GCube includes cultural prompts, metrics, and cultural concept spaces, enabling comprehensive assessment of T2I models' cultural knowledge and diversity. Our evaluations reveal significant gaps in the cultural knowledge of existing models and provide valuable insights into the diversity of image outputs for under-specified prompts. By introducing a novel approach to evaluating cultural diversity and knowledge in T2I models, T2I-GCube will be instrumental in fostering the development of models with enhanced cultural competence.
View details
ViSAGe: A Global-Scale Analysis of Visual Stereotypes in Text-to-Image Generation
Akshita Jha
Sarah Laszlo
Rida Qadri
Chandan Reddy
ACL (2024)
Preview abstract
Recent studies have highlighted the issue of varying degrees of stereotypical depictions for different identity group. However, these existing approaches have several key limitations, including a noticeable lack of coverage of identity groups in their evaluation, and the range of their associated stereotypes. Additionally, these studies often lack a critical distinction between inherently visual stereotypes, such as `brown' or `sombrero', and culturally influenced stereotypes like `kind' or `intelligent'. In this work, we address these limitations by grounding our evaluation of regional, geo-cultural stereotypes in the generated images from Text-to-Image models by leveraging existing textual resources. We employ existing stereotype benchmarks to evaluate stereotypes and focus exclusively on the identification of visual stereotypes within the generated images spanning 135 identity groups. We also compute the offensiveness across identity groups, and check the feasibility of identifying stereotypes automatically. Further, through a detailed case study and quantitative analysis, we reveal how the default representations of all identity groups have a more stereotypical appearance, and for historically marginalized groups, how the images across different attributes are visually more similar than other groups, even when explicitly prompted otherwise.
View details
Preview abstract
Stereotypes are oversimplified beliefs and ideas about particular groups of people. These cognitive biases are omnipresent in our language, reflected in human-generated dataset and potentially learned and perpetuated by language technologies. Although mitigating stereotypes in language technologies is necessary for preventing harms, stereotypes can impose varying levels of risks for targeted individuals and social groups by appearing in various contexts. Technical challenges in detecting stereotypes are rooted in the societal nuances of stereotyping, making it impossible to capture all intertwined interactions of social groups in diverse cultural context in one generic benchmark. This paper delves into the nuances of detecting stereotypes in an annotation task with humans from various regions of the world. We iteratively disambiguate our definition of the task, refining it as detecting ``generalizing language'' and contribute a multilingual, annotated dataset consisting of sentences mentioning a wide range of social identities in 9 languages and labeled on whether they make broad statements and assumptions about those groups. We experiment with training generalizing language detection models, which provide insight about the linguistic context in which stereotypes can appear, facilitating future research in addressing the dynamic, social aspects of stereotypes.
View details
Beyond Aesthetics: Cultural Competence in Text-to-Image Models
Nithish Kannen
Marco Andreetto
Adji Bousso Dieng
2024
Preview abstract
Use of Text-to-Image models is expanding beyond generating generic objects, as they are increasingly being adopted by diverse global communities to create visual representations of their unique culture. Current T2I benchmarks primarily evaluate image-text alignment, aesthetics and fidelity of generations for complex prompts with generic objects, overlooking the critical dimension of cultural understanding. In this work, we address this gap by defining a framework to evaluate cultural competence of T2I models, and present a scalable approach to collect cultural artifacts unique to a particular culture from Knowledge Graphs and Large Language Models in tandem. We assess the ability of state-of-the-art T2I models to generate culturally faithful and realistic images across 8 countries and 3 cultural domains. Furthermore, we emphasize the importance of T2I models reflecting a culture's diversity and introduce cultural diversity as a novel metric for T2I evaluation, drawing inspiration from the Vendi Score. We introduce T2I-GCube, a first-of-its-kind benchmark for T2I evaluation. T2I-GCube includes cultural prompts, metrics, and cultural concept spaces, enabling comprehensive assessment of T2I models' cultural knowledge and diversity. Our evaluations reveal significant gaps in the cultural knowledge of existing models and provide valuable insights into the diversity of image outputs for under-specified prompts. By introducing a novel approach to evaluating cultural diversity and knowledge in T2I models, T2I-GCube will be instrumental in fostering the development of models with enhanced cultural competence.
View details
Preview abstract
Detecting offensive content in text is an increasingly central challenge for both social-media platforms and AI-driven technologies. However offensiveness remains a subjective phenomenon as perspectives differ across sociodemographic characteristics, as well as cultural norms and moral values. This intricacy is largely ignored in the current AI-focused approaches for detecting offensiveness or related concepts such as hate speech and toxicity detection. We frame the task of determining offensiveness as essentially a matter of moral judgment --- deciding the boundaries of ethically wrong vs. right language to be used or generated within an implied set of sociocultural norms. In this paper, we investigate how judgment of offensiveness varies across diverse global cultural regions, and the crucial role of moral values in shaping these variations. Our findings highlight substantial cross-cultural differences in perceiving offensiveness, with moral concerns about Caring and Purity as the mediating factor driving these differences. These insights are of importance as AI safety protocols, shaped by human annotators' inputs and perspectives, embed their moral values which do not align with the notions of right and wrong in all contexts, and for all individuals.
View details
Intersecting Demographics: Bayesian Multilevel Models Reveal Age, Gender, and Racial Differences in Safety Perception of Chatbot Conversations
Chris Homan
Greg Serapio-García
Alex Taylor
(2023)
Preview abstract
Chatbots based on large language models (LLM) exhibit a level of human-like behavior that promises to have profound impacts on how people access information, create content, and seek social support. Yet these models have also shown a propensity toward biases and hallucinations, i.e., make up entirely false information and convey it as truthful. Consequently, understanding and moderating safety risks in these models is a critical technical and social challenge. We use Bayesian multilevel models to explore the connection between rater demographics and their perception of safety in chatbot dialogues. We study a sample of 252 human raters stratified by gender, age, race/ethnicity, and location. Raters were asked to annotate the safety risks of 1,340 chatbot conversations. We show that raters from certain demographic groups are more likely to report safety risks than raters from other groups. We discuss the implications of these differences in safety perception and suggest measures to ameliorate these differences.
View details
Building Stereotype Repositories with Complementary Approaches
Akshita Jha
Jaya Goyal
Dinesh Tewari
C3NLP workshop at EACL 2023 (2023)
Preview abstract
Measurements of fairness in NLP have been critiqued for lacking concrete definitions of biases or harms measured, and for perpetuating a singular, Western narrative of fairness globally. To combat some of these pivotal issues, methods for curating datasets and benchmarks that target specific harms are rapidly emerging. However, these methods still face the significant challenge of achieving coverage over global cultures and perspectives at scale. To address this, in this paper, we highlight the utility and importance of complementary approaches in these curation strategies, which leverage both community engagement as well as large generative models. We specifically target the harm of stereotyping and demonstrate a pathway to build a benchmark that covers stereotypes about diverse, and intersectional identities.
View details
Preview abstract
Along with the recent advances in large language modeling, there is growing concern that language technologies may reflect, propagate, and amplify various social stereotypes about groups of people. Publicly available stereotype benchmarks play a crucial role in detecting and mitigating this issue in language technologies to prevent both representational and allocational harms in downstream applications. However, existing stereotype benchmarks are limited in their size and coverage, largely restricted to stereotypes prevalent in the Western society. This is especially problematic as language technologies are gaining hold across the globe. To address this gap, we present SeeGULL, a broad-coverage stereotype dataset, expanding the coverage by utilizing the generative capabilities of large language models such as PaLM and GPT-3, and leveraging a globally diverse rater pool to validate prevalence of those stereotypes in society. SeeGULL is an order of magnitude larger in terms of size, and contains stereotypes for 179 identity groups spanning 6 continents, 8 different regions, 178 countries, 50 US states, and 31 Indian states and union territories. We also get fine-grained offensiveness scores for different stereotypes and demonstrate how stereotype perceptions for the same identity group differs across in-region vs out-region annotators.
View details