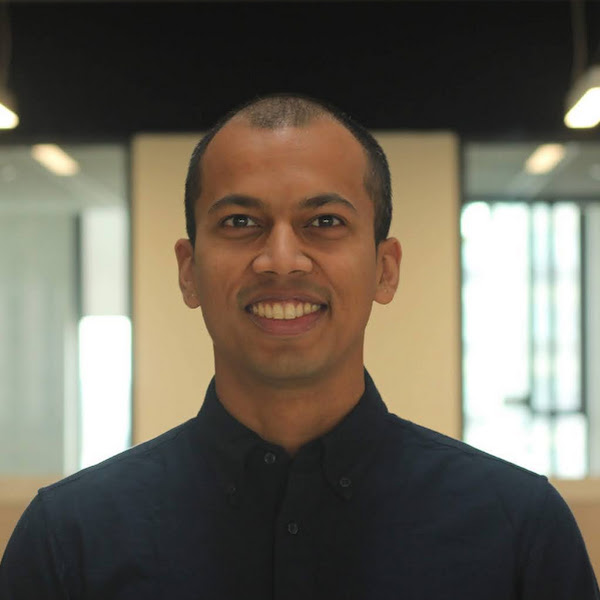
Vrushank Phadnis
Research Areas
Authored Publications
Sort By
InstructPipe: Building Visual Programming Pipelines in Visual Blocks with Human Instructions Using LLMs
Zhongyi Zhou
Jing Jin
Xiuxiu Yuan
Jun Jiang
Jingtao Zhou
Yiyi Huang
Kristen Wright
Jason Mayes
Mark Sherwood
Alex Olwal
Ram Iyengar
Na Li
Proceedings of the 2025 CHI Conference on Human Factors in Computing Systems (CHI), ACM, pp. 23
Preview abstract
Visual programming provides beginner-level programmers with a coding-free experience to build their customized pipelines. Existing systems require users to build a pipeline entirely from scratch, implying that novice users need to set up and link appropriate nodes all by themselves, starting from a blank workspace. We present InstructPipe, an AI assistant that enables users to start prototyping machine learning (ML) pipelines with text instructions. We designed two LLM modules and a code interpreter to execute our solution. LLM modules generate pseudocode of a target pipeline, and the interpreter renders a pipeline in the node-graph editor for further human-AI collaboration. Technical evaluations reveal that InstructPipe reduces user interactions by 81.1% compared to traditional methods. Our user study (N=16) showed that InstructPipe empowers novice users to streamline their workflow in creating desired ML pipelines, reduce their learning curve, and spark innovative ideas with open-ended commands.
View details
H2E: Hand, Head, Eye: A Multimodal Cascade of Natural Inputs
Khushman Patel
Ken Pfeuffer
Hans Gellersen
IEEE VR (2025)
Preview abstract
Eye-based interaction techniques for extended reality, such as gaze and pinch, are simple to use however suffer from input precision issues. We present H2E, a fine and coarse-grained pointing technique that cascades Hand, Head, and Eye inputs. As users initiate a pinch gesture, a cursor appears at the gaze point that can be dragged by head pointing before pinch confirmation. This has the potential advantage that it can add a precision component without changing the semantics of the technique. In this paper, we describe the design and implementation of the technique. Furthermore, we present an evaluation of our method in a Fitts-based user study, exploring the speed-accuracy trade-offs against a gaze and pinch interaction baseline.
View details
Experiencing InstructPipe: Building Multi-modal AI Pipelines via Prompting LLMs and Visual Programming
Zhongyi Zhou
Jing Jin
Xiuxiu Yuan
Jun Jiang
Jingtao Zhou
Yiyi Huang
Kristen Wright
Jason Mayes
Mark Sherwood
Alex Olwal
Ram Iyengar
Na Li
Extended Abstracts of the 2024 CHI Conference on Human Factors in Computing Systems, ACM, pp. 5
Preview abstract
Foundational multi-modal models have democratized AI access, yet the construction of complex, customizable machine learning pipelines by novice users remains a grand challenge. This paper demonstrates a visual programming system that allows novices to rapidly prototype multimodal AI pipelines. We first conducted a formative study with 58 contributors and collected 236 proposals of multimodal AI pipelines that served various practical needs. We then distilled our findings into a design matrix of primitive nodes for prototyping multimodal AI visual programming pipelines, and implemented a system with 65 nodes. To support users' rapid prototyping experience, we built InstructPipe, an AI assistant based on large language models (LLMs) that allows users to generate a pipeline by writing text-based instructions. We believe InstructPipe enhances novice users onboarding experience of visual programming and the controllability of LLMs by offering non-experts a platform to easily update the generation.
View details
The Work Avatar Face-Off: Knowledge Worker Preferences for Realism in Meetings
Kristin Moore
22nd IEEE International Symposium on Mixed and Augmented Reality (ISMAR) (2023) (to appear)
Preview abstract
While avatars have grown in popularity in social settings, their use in the workplace is still debatable. We conducted a large-scale survey to evaluate knowledge worker sentiment towards avatars, particularly the effects of realism on their acceptability for work meetings. Our survey of 2509 knowledge workers from multiple countries rated five avatar styles for use by managers, known colleagues and unknown colleagues.
In all scenarios, participants favored higher realism, but fully realistic avatars were sometimes perceived as uncanny. Less realistic avatars were rated worse when interacting with an unknown colleague or manager, as compared to a known colleague. Avatar acceptability varied by country, with participants from the United States and South Korea rating avatars more favorably. We supplemented our quantitative findings with a thematic analysis of open-ended responses to provide a comprehensive understanding of factors influencing work avatar choices.
In conclusion, our results show that realism had a significant positive correlation with acceptability. Non-realistic avatars were seen as fun and playful, but only suitable for occasional use.
View details