Publications
Our teams aspire to make discoveries that impact everyone, and core to our approach is sharing our research and tools to fuel progress in the field.
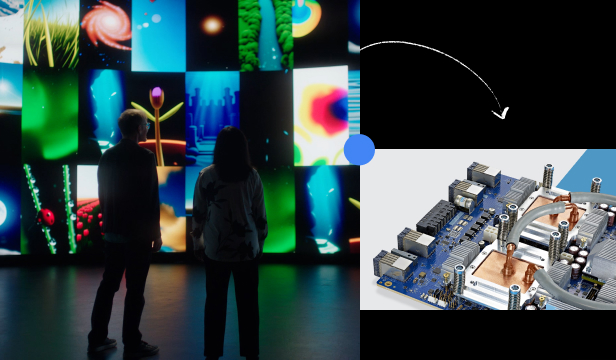
Our teams aspire to make discoveries that impact everyone, and core to our approach is sharing our research and tools to fuel progress in the field.
Sort By
1 - 15 of 10233 publications
Beyond Touchscreens: Designing for Co-Occurring Accessibility Needs
Melissa Barnhart Wantland
Mai Kobori
Universal Access in Human-Computer Interaction, Springer-Verlag (2025) (to appear)
Preview abstract
Today’s smartphone interactions are typically designed with one primary preset, accompanied by customization settings that can be manually adjusted. To promote the creation of contextually aware experiences, researchers have highlighted the factors that influence mobile device usage in the ability-based design framework. This paper expands upon existing frameworks and contributes to an empirical understanding of smartphone accessibility. Through a 10-day longitudinal diary study and video interview with 24 individuals who do and do not identify as having a disability, the research also illustrates the reactions of reattempt, adaptation, and avoidance, which were used in response to a lack of smartphone accessibility. Despite experiencing scenarios where accessibility settings could be leveraged, 20 out of 24 participants did not use accessibility settings on their smartphone. A total of 12 out of 24 participants tried accessibility settings on their smartphones, however identifying accessibility was not for them. This work highlights the need to shift current design practices to better serve the accessibility community.
View details
Preview abstract
Multimodal AI Agents are AI models that have the capability of interactively and cooperatively assisting human users to solve day-to-day tasks. Augmented Reality (AR) head worn devices can uniquely improve the user experience of solving procedural day-to-day tasks by providing egocentric multimodal (audio and video) observational capabilities to AI Agents. Such AR capabilities can help the AI Agents see and listen to actions that users take which can relate to multimodal capabilities of human users. Existing AI Agents, either Large Language Models (LLMs) or Multimodal Vision-Language Models (VLMs) are reactive in nature, which means that models cannot take an action without reading or listening to the human user's prompts. Proactivity of AI Agents, on the other hand, can help the human user detect and correct any mistakes in agent observed tasks, encourage users when they do tasks correctly, or simply engage in conversation with the user - akin to a human teaching or assisting a user. Our proposed YET to Intervene (YETI) multimodal Agent focuses on the research question of identifying circumstances that may require the Agent to intervene proactively. This allows the Agent to understand when it can intervene in a conversation with human users that can help the user correct mistakes on tasks, like cooking, using Augmented Reality. Our YETI Agent learns scene understanding signals based on interpretable notions of Structural Similarity (SSIM) on consecutive video frames. We also define the alignment signal which the AI Agent can learn to identify if the video frames corresponding to the user's actions on the task are consistent with expected actions. These signals are used by our AI Agent to determine when it should proactively intervene. We compare our results on the instances of proactive intervention in the HoloAssist multimodal benchmark for an expert agent guiding an user agent to complete procedural tasks.
View details
Gemini & Physical World: Large Language Models Can Estimate the Intensity of Earthquake Shaking from Multi-Modal Social Media Posts
Marc Stogaitis
Tajinder Gadh
Richard Allen
Alexei Barski
Robert Bosch
Patrick Robertson
Youngmin Cho
Nivetha Thiruverahan
Aman Raj
Geophysical Journal International (2025), ggae436
Preview abstract
This paper presents a novel approach for estimating the ground shaking intensity using real-time social media data and CCTV footage. Employing the Gemini 1.5 Pro’s (Reid et al. 2024) model, a multi-modal language model, we demonstrate the ability to extract relevant information from unstructured data utilizing generative AI and natural language processing. The model’s output, in the form of Modified Mercalli Intensity (MMI) values, align well with independent observational data. Furthermore, our results suggest that beyond its advanced visual and auditory understanding abilities, Gemini appears to utilize additional sources of knowledge, including a simplified understanding of the general relationship between earthquake magnitude, distance, and MMI intensity, which it presumably acquired during its training, in its reasoning and decision-making processes. These findings raise intriguing questions about the extent of Gemini's general understanding of the physical world and its phenomena. Gemini’s ability to generate results consistent with established scientific knowledge highlights the potential of LLMs like Gemini in augmenting our understanding of complex physical phenomena such as earthquakes. More specifically, the results of this study highlight the potential of LLMs like Gemini to revolutionize citizen seismology by enabling rapid, effective, and flexible analysis of crowdsourced data from eyewitness accounts for assessing earthquake impact and providing crisis situational awareness. This approach holds a great promise for improving early warning systems, disaster response, and overall resilience in earthquake-prone regions. This study provides a significant step toward harnessing the power of social media and AI for earthquake disaster mitigation.
View details
Governance, Risk and Compliance (GRC) Engineering: Data, AI, Automation, and the Future of Compliance to Audits
Eric Zhang
Ruchi Khurana
Vikram Khare
2025
Preview abstract
In today's rapidly evolving business landscape, Governance, Risk, and Compliance (GRC) leaders in large, complex organizations face unprecedented challenges. The cloud has revolutionized how businesses operate, offering unprecedented scalability, flexibility, cost-efficiency, additional security and resilience. However, this transformation also presents new challenges for GRC professionals. In a cloud-native world, where applications are built and deployed in dynamic, distributed environments, traditional GRC on-prem approaches, manual processes and spreadsheets struggle to keep pace. The key to success lies in embracing a data-driven GRC strategy that leverages the power of the cloud to enhance agility, visibility, and resilience.
View details
Linear Elastic Caching via Ski Rental
Todd Lipcon
The biennial Conference on Innovative Data Systems Research (2025)
Preview abstract
In this work we study the Linear Elastic Caching problem, where the goal is to minimize the total cost of a cache inclusive of not just its misses, but also its memory footprint integrated over time. We demonstrate a theoretical connection to the classic ski rental problem and propose a practical algorithm that combines online caching algorithms with ski rental policies. We also introduce a lightweight machine learning-based algorithm for ski rental that is optimized for production workloads and is easy to integrate within existing database systems. Evaluations on both production workloads in Google Spanner and publicly available traces show that the proposed elastic caching approach can significantly reduce the total cache cost compared to traditional fixed-size cache policies.
View details
Preview abstract
We study the existence of almost fair and near-optimal solutions to a routing problem as defined in the seminal work of Rosenthal. We focus on the setting where multiple alternative routes are available for each potential request (which corresponds to a potential user of the network). This model captures a collection of diverse applications such as packet routing in communication networks, routing in road networks with multiple alternative routes, and the economics of transportation of goods.
Our recommended routes have provable guarantees in terms of both the total cost and fairness concepts such as approximate envy-freeness. We employ and appropriately combine tools from algorithmic game theory and fair division. Our results apply on two distinct models: the splittable case where the request is split among the selected paths (e.g., routing a fleet of trucks) and the unsplittable case where the request is assigned to one of its designated paths (e.g., a single user request). Finally, we conduct an empirical analysis to test the performance of our approach against simpler baselines using the real world road network of New York City.
View details
PreFix: Optimizing the Performance of Heap-Intensive Applications
Chaitanya Mamatha Ananda
Rajiv Gupta
Han Shen
CGO 2025: International Symposium on Code Generation and Optimization, Las Vegas, NV, USA (to appear)
Preview abstract
Analyses of heap-intensive applications show that a small fraction of heap objects account for the majority of heap accesses and data cache misses. Prior works like HDS and HALO have shown that allocating hot objects in separate memory regions can improve spatial locality leading to better application performance. However, these techniques are constrained in two primary ways, limiting their gains. First, these techniques have Imperfect Separation, polluting the hot memory region with several cold objects. Second, reordering of objects across allocations is not possible as the original object allocation order is preserved. This paper presents a novel technique that achieves near perfect separation of hot objects via a new context mechanism that efficiently identifies hot objects with high precision. This technique, named PreFix, is based upon Preallocating memory for a Fixed small number of hot objects. The program, guided by profiles, is instrumented to compute context information derived from
dynamic object identifiers, that precisely identifies hot object allocations that are then placed at predetermined locations in the preallocated memory. The preallocated memory region for hot objects provides the flexibility to reorder objects across allocations and allows colocation of objects that are part of a hot data stream (HDS), improving spatial locality. The runtime overhead of identifying hot objects is not significant as this optimization is only focused on a small number of static hot allocation sites and dynamic hot objects. While there is an increase in the program’s memory foot-print, it is manageable and can be controlled by limiting the size of the preallocated memory. In addition, PreFix incorporates an object recycling optimization that reuses the same preallocated space to store different objects whose lifetimes are not expected to overlap. Our experiments with 13 heap-intensive applications yields reductions in execution times ranging from 2.77% to 74%. On average PreFix reduces execution time by 21.7% compared to 7.3% by HDS and 14% by HALO. This is due to PreFix’s precision in hot object identification, hot object colocation, and low runtime overhead.
View details
Circadian rhythm of heart rate and activity: a cross-sectional study
Maryam Khalid
Logan Schneider
Aravind Natarajan
Conor Heneghan
Karla Gleichauf
Chronobiology International (2025)
Preview abstract
ABSTRACT
Background: Circadian rhythms are commonly observed in a number of physiological processes. Consumer wearable devices have made it possible to obtain continuous time series data from a large number of individuals. We study circadian rhythms from measurements of heart rate, movement, and sleep, from a cohort of nearly 20,000 participants over the course of 30 days.
Methods: Participation was restricted to Fitbit users of age 21 years or older residing in the United States or Canada. Participants were enrolled through a recruitment banner shown on the Fitbit App. The advertisement was shown to 531,359 Fitbit users, and 23,239 enrolled in the program. Of these, we obtained heart rate data from 19,350 participants. We obtain the underlying circadian rhythm from time series heart rate by modeling the circadian rhythm as a sum over the first two Fourier harmonics. The first Fourier harmonic accounts for the 24-hour rhythmicity, while the second harmonic accounts for non-sinusoidal perturbations.
Findings: We observe a circadian rhythm in both heart rate and acceleration. From the diurnal modulation, we obtain the following circadian parameters: (i) amplitude of modulation, (ii) bathyphase, (iii) acrophase, (iv) non-sinusoidal fraction, and (v) fraction of day when the heart rate is greater than the mean. The amplitude, bathyphase, and acrophase depend on sex, and decrease with age. The waketime on average, follows the bathyphase by 2.4 hours. In most individuals, the circadian rhythm of heart rate lags the circadian rhythm of activity.
Interpretation: Circadian metrics for heart rate and activity can be reliably obtained from commercially available wearable devices. Distributions of circadian metrics can be valuable tools for individual-level interpretation.
View details
Preview abstract
Mainstream artificial neural network models, such as Deep Neural Networks (DNNs) are computation-heavy and energy-hungry. Weightless Neural Networks (WNNs) are natively built with RAM-based neurons and represent an entirely distinct type of neural network computing compared to DNNs. WNNs are extremely low-latency, low-energy, and suitable for efficient, accurate, edge inference. The WNN approach derives an implicit inspiration from the decoding process observed in the dendritic trees of biological neurons, making neurons based on Random Access Memories (RAMs) and/or Lookup Tables (LUTs) ready-to-deploy neuromorphic digital circuits. Since FPGAs are abundant in LUTs, LUT based WNNs are a natural fit for implementing edge inference in FPGAs.
WNNs has been demonstrated to be an energetically efficient AI model, both in software, as well as in hardware. For instance, the most recent DWN – Differential Weightless Neural Network – model demonstrates up to 135× reduction in energy costs in FPGA implementations compared to other multiplication-free approaches, such as binary neural networks (BNNs) and DiffLogicNet, up to 9% higher accuracy in deployments on constrained devices, and culminate in up to 42.8× reduction in circuit area for ultra-low-cost chip implementations. This tutorial will help participants understand how WNNs work, why WNNs were underdogs for such a long time, and be introduced to the most recent members of the WNN family, such as BTHOWeN , LogicWiSARD, COIN, ULEEN and DWN, and contrast to BNNs and LogicNets.
View details
Security Signals: Making Web Security Posture Measurable At Scale
David Dworken
Artur Janc
Santiago (Sal) Díaz
Workshop on Measurements, Attacks, and Defenses for the Web (MADWeb)
Preview abstract
The area of security measurability is gaining increased attention, with a wide range of organizations calling for the development of scalable approaches for assessing the security of software systems and infrastructure. In this paper, we present our experience developing Security Signals, a comprehensive system providing security measurability for web services, deployed in a complex application ecosystem of thousands of web services handling traffic from billions of users. The system collects security-relevant information from production HTTP traffic at the reverse proxy layer, utilizing novel concepts such as synthetic signals augmented with additional risk information to provide a holistic view of the security posture of individual services and the broader application ecosystem. This approach to measurability has enabled large-scale security improvements to our services, including prioritized rollouts of security enhancements and the implementation of automated regression monitoring. Furthermore, it has proven valuable for security research and prioritization of defensive work. Security Signals addresses shortcomings of prior web measurability proposals by tracking a comprehensive set of security properties relevant to web applications, and by extracting insights from collected data for use by both security experts and non-experts. We believe the lessons learned from the implementation and use of Security Signals offer valuable insights for practitioners responsible for web service security, potentially inspiring new approaches to web security measurability.
View details
Triaging mammography with artificial intelligence: an implementation study
Sarah M. Friedewald
Sunny Jansen
Fereshteh Mahvar
Timo Kohlberger
David V. Schacht
Sonya Bhole
Dipti Gupta
Scott Mayer McKinney
Stacey Caron
David Melnick
Mozziyar Etemadi
Samantha Winter
Alejandra Maciel
Luca Speroni
Martha Sevenich
Arnav Agharwal
Rubin Zhang
Gavin Duggan
Shiro Kadowaki
Atilla Kiraly
Jie Yang
Basil Mustafa
Krish Eswaran
Shravya Shetty
Breast Cancer Research and Treatment (2025)
Preview abstract
Purpose
Many breast centers are unable to provide immediate results at the time of screening mammography which results in delayed patient care. Implementing artificial intelligence (AI) could identify patients who may have breast cancer and accelerate the time to diagnostic imaging and biopsy diagnosis.
Methods
In this prospective randomized, unblinded, controlled implementation study we enrolled 1000 screening participants between March 2021 and May 2022. The experimental group used an AI system to prioritize a subset of cases for same-visit radiologist evaluation, and same-visit diagnostic workup if necessary. The control group followed the standard of care. The primary operational endpoints were time to additional imaging (TA) and time to biopsy diagnosis (TB).
Results
The final cohort included 463 experimental and 392 control participants. The one-sided Mann-Whitney U test was employed for analysis of TA and TB. In the control group, the TA was 25.6 days [95% CI 22.0–29.9] and TB was 55.9 days [95% CI 45.5–69.6]. In comparison, the experimental group's mean TA was reduced by 25% (6.4 fewer days [one-sided 95% CI > 0.3], p<0.001) and mean TB was reduced by 30% (16.8 fewer days; 95% CI > 5.1], p=0.003). The time reduction was more pronounced for AI-prioritized participants in the experimental group. All participants eventually diagnosed with breast cancer were prioritized by the AI.
Conclusions
Implementing AI prioritization can accelerate care timelines for patients requiring additional workup, while maintaining the efficiency of delayed interpretation for most participants. Reducing diagnostic delays could contribute to improved patient adherence, decreased anxiety and addressing disparities in access to timely care.
View details
InstructPipe: Building Visual Programming Pipelines in Visual Blocks with Human Instructions Using LLMs
Zhongyi Zhou
Jing Jin
Xiuxiu Yuan
Jun Jiang
Jingtao Zhou
Yiyi Huang
Kristen Wright
Jason Mayes
Mark Sherwood
Alex Olwal
Ram Iyengar
Na Li
Proceedings of the 2025 CHI Conference on Human Factors in Computing Systems (CHI), ACM, pp. 23
Preview abstract
Visual programming provides beginner-level programmers with a coding-free experience to build their customized pipelines. Existing systems require users to build a pipeline entirely from scratch, implying that novice users need to set up and link appropriate nodes all by themselves, starting from a blank workspace. We present InstructPipe, an AI assistant that enables users to start prototyping machine learning (ML) pipelines with text instructions. We designed two LLM modules and a code interpreter to execute our solution. LLM modules generate pseudocode of a target pipeline, and the interpreter renders a pipeline in the node-graph editor for further human-AI collaboration. Technical evaluations reveal that InstructPipe reduces user interactions by 81.1% compared to traditional methods. Our user study (N=16) showed that InstructPipe empowers novice users to streamline their workflow in creating desired ML pipelines, reduce their learning curve, and spark innovative ideas with open-ended commands.
View details
Preview abstract
https://www.overleaf.com/project/65ba7d45dae2bce751dba252
Hashing is a fundamental operation in various computer sci-
ence applications. Despite the prevalence of specific key
formats like social security numbers, MAC addresses, plate
numbers, and URLs, hashing libraries typically treat them as
general byte sequences. This paper introduces a technique
for synthesizing specialized hash functions tailored to par-
ticular byte formats. The proposed code generation method
leverages three prevalent patterns: (i) fixed-length keys, (ii)
keys with common subsequences, and (iii) keys ranging on
predetermined sequences of bytes. The code generation pro-
cess involves two algorithms: one identifies relevant regular
expressions within key examples, and the other generates
specialized hash functions based on these expressions. This
approach, straightforward to implement, showcases improve-
ments over highly optimized hash function implementations.
Comparative analysis demonstrates that our synthetic func-
tions outperform counterparts in the C++ Standard Template
Library and the Google Abseil Library, achieving speedups
ranging from 2% to 11%, depending on the key format.
View details
Preview abstract
The problem of contract design addresses the challenge of moral hazard in principle-agent setups. The agent exerts costly efforts that produce a random outcome with an associated reward for the principal. Moral hazard refers to the tension that the principal cannot observe the agent’s effort level hence needs to incentivize the agent only through rewarding the realized effort outcome, i.e., the contract. Bayesian contract design studies the principal’s design problem of an optimal contract when facing an unknown agent characterized by a private Bayesian type. In its most general form, the agent’s type is inherently “multi-parameter” and can arbitrarily affect both the agent’s productivity and effort costs. In contrast, a natural single-parameter setting of much recent interest simplifies the agent’s type to a single value that describes the agent’s cost per unit of effort, whereas agents’ efforts are assumed to be equally
productive.
The main result of this paper is an almost approximation-preserving polynomial-time reduction from the most general multi-parameter Bayesian contract design (BCD) to single-parameter BCD. That is, for any multi-parameter BCD instance I^M, we construct a single-parameter instance I^S such that any β-approximate contract (resp. menu of contracts) of I^S can in turn be converted to a (β − ϵ)-approximate contract (resp. menu of contracts) of I^M. The reduction is in time polynomial in the input size and log(1/ϵ); moreover, when β = 1 (i.e., the given single-parameter solution is exactly optimal), the dependence on 1/ϵ can be removed, leading to a polynomial-time exact reduction. This efficient reduction is somewhat surprising because in the closely related problem of Bayesian mechanism design, a polynomial-time reduction from multi-parameter to single-parameter setting is believed to not exist. Our result demonstrates the intrinsic difficulty of addressing moral hazard in Bayesian contract design, regardless of being single-parameter or multi-parameter.
As byproducts, our reduction answers two open questions in recent literature of algorithmic contract design: (a) it implies that optimal contract design in single-parameter BCD is not in APX unless P=NP even when the agent’s type distribution is regular, answering the open question of [3] in the negative; (b) it implies that the principal’s (order-wise) tight utility gap between using a menu of contracts and a single contract is Θ(n) where n is the number of actions, answering the major open question of [27] for the single-parameter case.
View details
H2E: Hand, Head, Eye: A Multimodal Cascade of Natural Inputs
Khushman Patel
Ken Pfeuffer
Hans Gellersen
IEEE VR (2025)
Preview abstract
Eye-based interaction techniques for extended reality, such as gaze and pinch, are simple to use however suffer from input precision issues. We present H2E, a fine and coarse-grained pointing technique that cascades Hand, Head, and Eye inputs. As users initiate a pinch gesture, a cursor appears at the gaze point that can be dragged by head pointing before pinch confirmation. This has the potential advantage that it can add a precision component without changing the semantics of the technique. In this paper, we describe the design and implementation of the technique. Furthermore, we present an evaluation of our method in a Fitts-based user study, exploring the speed-accuracy trade-offs against a gaze and pinch interaction baseline.
View details