Connectomics
Our goal is to leverage Google expertise and resources to advance understanding of the structure and function of the brain.
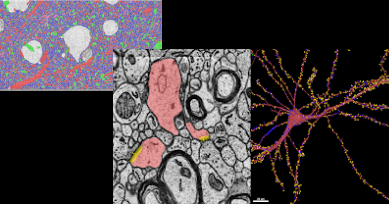
Our goal is to leverage Google expertise and resources to advance understanding of the structure and function of the brain.
About our team
A major hypothesis in modern neuroscience is that neuron-to-neuron connectivity structure in the brain can be linked to function -- how the brain encodes memories, extracts features from perceptual stimuli, and makes decisions. However, the structure of these brain networks has remained largely unknown, due to technical difficulties involved in imaging and reconstructing the brain in 3D.
New microscopy techniques have begun to address the challenge of imaging the brain in 3D at nanometer resolution, and 4D at cellular resolution, but this has led to a huge bottleneck in the subsequent step of data analysis. Our goal is to help solve some of these data analysis problems and thus enable a high-throughput approach to studying the network architecture of the brain.
In order to facilitate this work we collaborate with the Max Planck Institute, HHMI Janelia Research Campus, Harvard University, and other organizations.
Team focus summaries
We develop algorithms and software for automating the process of aligning, segmenting, and annotating petabyte-scale 3d images of brain tissue.
We develop software such as TensorStore and Neuroglancer which helps store, process, and visualize large n-dimensional images and volumes.
Featured publications
Highlighted work
-
A Browsable Petascale Reconstruction of the Human CortexIn collaboration with the Lichtman Laboratory at Harvard University, we are releasing the “H01” dataset, a 1.4 petabyte rendering of a small sample of human brain tissue, along with a companion paper, “A connectomic study of a petascale fragment of human cerebral cortex.
-
The Drosophila Hemibrain Connectome: The Largest Synapse-Resolution Map of Brain ConnectivityIn collaboration with the FlyEM team at HHMI’s Janelia Research Campus and several other research partners, we released the "hemibrain" connectome, a highly detailed map of neuronal connectivity in the fly brain, along with a suite of tools for visualization and analysis.
-
Flood-Filling Networks: Improving Connectomics by an Order of MagnitudeIn collaboration with colleagues at the Max Planck Institute of Neurobiology, we published High-Precision Automated Reconstruction of Neurons with Flood-Filling Networks in Nature Methods, which shows how a new type of recurrent neural network can improve the accuracy of automated interpretation of connectomics data by an order of magnitude over previous deep learning techniques.
-
NeuroglancerNeuroglancer is a WebGL-based viewer for petascale multidimensional data. It is capable of displaying arbitrary (non axis-aligned) cross-sectional views of volumetric data, as well as 3-D meshes and line-segment based models (skeletons).
-
TensorStoreCloud-first library for reading and writing large multi-dimensional arrays, with advanced concurrency and indexing capabilities.
Some of our locations
Some of our people
-
Tim Blakely
- General Science
- Machine Intelligence
- Robotics
-
Viren Jain
- General Science
- Machine Perception
-
Michał Januszewski
- Distributed Systems and Parallel Computing
- General Science
- Machine Intelligence
-
Laramie Leavitt
-
Peter H. Li
- General Science
- Machine Intelligence
- Machine Perception
-
Jeremy Maitin-Shepard
- Machine Intelligence
- Machine Perception
-
Art Pope
- Machine Intelligence
- Machine Perception