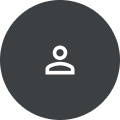
Sofia Erell
Sofia Erell is a SWE in Israel's Google Research group, focusing on medical NLP.
Research Areas
Authored Publications
Sort By
LLMs Accelerate Annotation for Medical Information Extraction
Akshay Goel
Almog Gueta
Omry Gilon
Chang Liu
Xiaohong Hao
Bolous Jaber
Shashir Reddy
Rupesh Kartha
Jean Steiner
Machine Learning for Health (ML4H), PMLR (2023)
Preview abstract
The unstructured nature of clinical notes within electronic health records often conceals vital patient-related information, making it challenging to access or interpret. To uncover this hidden information, specialized Natural Language Processing (NLP) models are required. However, training these models necessitates large amounts of labeled data, a process that is both time-consuming and costly when relying solely on human experts for annotation. In this paper, we propose an approach that combines Large Language Models (LLMs) with human expertise to create an efficient method for generating ground truth labels for medical text annotation. By utilizing LLMs in conjunction with human annotators, we significantly reduce the human annotation burden, enabling the rapid creation of labeled datasets. We rigorously evaluate our method on a medical information extraction task, demonstrating that our approach not only substantially cuts down on human intervention but also maintains high accuracy. The results highlight the potential of using LLMs to improve the utilization of unstructured clinical data, allowing for the swift deployment of tailored NLP solutions in healthcare.
View details
Learning and Evaluating a Differentially Private Pre-trained Language Model
Shlomo Hoory
Avichai Tendler
Ayelet Benjamini
Findings of the Association for Computational Linguistics: EMNLP 2021, Association for Computational Linguistics, Punta Cana, Dominican Republic, pp. 1178-1189
Preview abstract
Contextual language models have led to significantly better results on a plethora of language understanding tasks, especially when pre-trained on the same data as the downstream task. While this additional pre-training usually improves performance, it often leads to information leakage and therefore risks the privacy of individuals mentioned in the training data. One method to guarantee the privacy of such individuals is to train a differentially private model, but this usually comes at the expense of model performance. Moreover, it is hard to tell given a privacy parameter $\epsilon$ what was the effect on the trained representation and whether it maintained relevant information while improving privacy. To improve privacy and guide future practitioners and researchers, we demonstrate here how to train a differentially private pre-trained language model (i.e., BERT) with a privacy guarantee of $\epsilon=0.5$ with only a small degradation in performance. We experiment on a dataset of clinical notes with a model trained on an entity extraction (EE) task on and compare it to a similar model trained without differential privacy. Finally, we present a series of experiments showing how to interpret the differentially private representation and understand the information lost and maintained in this process.
View details