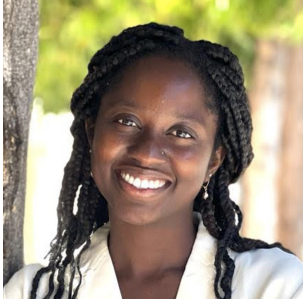
Mercy Nyamewaa Asiedu
I am a senior research scientist at Google Research, and my research interests lie in developing robust machine learning algorithms and Large Language Models for health. Before Google, I was a Schmidt Science Fellow at MIT, where I worked on different machine learning areas in medical imaging and clinical NLP. I obtained my PhD in Biomedical Engineering from Duke University with my thesis focused on developing devices and algorithms for cervical cancer screening. I obtained my BSc. in Biomedical Engineering and Business from the University of Rochester.
Research Areas
Authored Publications
Sort By
AfriMed-QA: A Pan-African Multi-Specialty Medical Question-Answering Benchmark Dataset
Tobi Olatunji
Abraham Toluwase Owodunni
Charles Nimo
Jennifer Orisakwe
Henok Biadglign Ademtew
Chris Fourie
Foutse Yuehgoh
Stephen Moore
Mardhiyah Sanni
Emmanuel Ayodele
Timothy Faniran
Bonaventure F. P. Dossou
Fola Omofoye
Wendy Kinara
Tassallah Abdullahi
Michael Best
2025
Preview abstract
Recent advancements in large language model (LLM) performance on medical multiple-choice question (MCQ) benchmarks have stimulated significant interest from patients and healthcare providers globally. Particularly in low- and middle-income countries (LMICs) facing acute physician shortages and lack of specialists, LLMs offer a potentially scalable pathway to enhance healthcare access and reduce costs. However, LLM training data is sourced from predominantly Western text, existing benchmarks are predominantly Western-centric, limited to MCQs, and focused on a narrow range of clinical specialties, raising concerns about their applicability in the Global South, particularly across Africa where localized medical knowledge and linguistic diversity are often underrepresented. In this work, we introduce AfriMed-QA, the first large-scale multi-specialty Pan-African medical Question-Answer (QA) dataset designed to evaluate and develop equitable and effective LLMs for African healthcare. It contains 3,000 multiple-choice professional medical exam questions with answers and rationale, 1,500 short answer questions (SAQ) with long-from answers, and 5,500 consumer queries, sourced from over 60 medical schools across 15 countries, covering 32 medical specialties. We further rigorously evaluate multiple open, closed, general, and biomedical LLMs across multiple axes including accuracy, consistency, factuality, bias, potential for harm, local geographic relevance, medical reasoning, and recall. We believe this dataset provides a valuable resource for practical application of large language models in African healthcare and enhances the geographical diversity of health-LLM benchmark datasets.
View details
Unprecedented Insights into Maternal Sleep: A Large-scale Longitudinal Analysis of Real-world Wearable Device Data Before, During, and After Pregnancy
Nichole Young-Lin
Conor Heneghan
Logan Schneider
Logan Niehaus
Ariel Haney
Karla Gleichauf
Jacqueline Shreibati
Belen Lafon
Lancet eBioMedicine (2025)
Preview abstract
Introduction: Current understanding of pregnancy and postpartum sleep is driven by limited lab or self-reported data. Consumer wearable devices may help reveal longitudinal, real-world sleep patterns.
Methods: We analyzed de-identified wearable device data from 2,540 users in the United States and Canada who met strict wear-time requirements (≥80% daily usage for ≥80% of the time periods of interest [12 weeks prepregnancy, throughout pregnancy, and 20 weeks immediately postpartum]). We tracked sleep time and staging using Fitbit devices.
Results: Compared to prepregnancy, total sleep time (TST) increased from an average of 425.3±43.5 min to a peak of 447.6±47.6 min at gestational week 10 with ongoing declines throughout pregnancy. Time in bed (TIB) followed a similar pattern. Increased light sleep drove the initial TST rise. Deep and REM sleep decreased significantly throughout pregnancy, with maximum reductions of 19.2±13.8 min (p<0.01) and 9.0±19.2 min (p<0.01) respectively by pregnancy end. Sleep efficiency also declined slightly during pregnancy (median drop from 88.3% to 86.8%). After delivery, TIB remained below the prepregnancy baseline by 14.7±45.7 min at one year postpartum and 15.2±47.7 min at 1.5 years postpartum.
Conclusion: This unprecedented look at large-scale, real-world sleep and pregnancy patterns revealed a previously unquantified initial increase in sleep followed by decreases in both quantity and quality as pregnancy progresses. Sleep deficits persist for at least 1.5 years postpartum. These quantified trends can assist clinicians and patients in understanding what to expect.
View details
Development and Evaluation of ML Models for Cardiotocography Interpretation
Nicole Chiou
Nichole Young-Lin
Abdoulaye Diack
Christopher Kelly
Sanmi Koyejo
NPJ Women's Health (2025)
Preview abstract
The inherent variability in the visual interpretation of cardiotocograms (CTGs) by obstetric clinical experts, both intra- and inter-observer, presents a substantial challenge in obstetric care. In response, we investigate automated CTG interpretation as a potential solution to enhance the early detection of fetal hypoxia during labor, thereby reducing unnecessary operative interventions and improving overall maternal and neonatal care. This study employs deep learning techniques to reduce the subjectivity associated with visual CTG interpretation. Our results demonstrate that employing objective cord blood pH measurements, rather than clinician-defined Apgar scores, yields more consistent and robust model performance. Additionally, through a series of ablation studies, we investigate the impact of temporal distribution shifts on the performance of these deep learning models. We examine tradeoffs between performance and fairness, specifically evaluating performance across demographic and clinical subgroups. Finally, we discuss the practical implications of our findings for the real-world deployment of such systems, emphasizing their potential utility in medical settings with limited resources.
View details
The Case for Globalizing Fairness: A Mixed Methods Study on the Perceptions of Colonialism, AI and Health in Africa
Iskandar Haykel
Chirag Nagpal
Aisha Walcott-Bryant
Sanmi Koyejo
2024
Preview abstract
With growing machine learning (ML) and large language model applications in healthcare, there have been calls for fairness in ML to understand and mitigate ethical concerns these systems may pose. Fairness has implications for health in Africa, which already has inequitable power imbalances between the Global North and South. This paper seeks to explore fairness for global health, with Africa as a case study.
We conduct a scoping review to propose fairness attributes for consideration in the African context and delineate where they may come into play in different ML-enabled medical modalities. We then conduct qualitative research studies with 625 general population study participants in 5 countries in Africa and 28 experts in ML, Health, and/or policy focussed on Africa to obtain feedback on the proposed attributes. We delve specifically into understanding the interplay between AI, health and colonialism.
Our findings demonstrate that among experts there is a general mistrust that technologies that are solely developed by former colonizers can benefit Africans, and that associated resource constraints due to pre-existing economic and infrastructure inequities can be linked to colonialism. General population survey responses found about an average of 40% of people associate an undercurrent of colonialism to AI and this was most dominant amongst participants from South Africa. However the majority of the general population participants surveyed did not think there was a direct link between AI and colonialism.Colonial history, country of origin, National income level were specific axes of disparities that participants felt would cause an AI tool to be biased
This work serves as a basis for policy development around Artificial Intelligence for health in Africa and can be expanded to other regions.
View details
TRINDs: Assessing the Diagnostic Capabilities of Large Language Models for Tropical and Infectious Diseases
Nenad Tomašev
Chintan Ghate
Steve Adudans
Oluwatosin Akande
Sylvanus Aitkins
Geoffrey Siwo
Lynda Osadebe
Eric Ndombi
2024
Preview abstract
Neglected tropical diseases (NTDs) and infectious diseases disproportionately affect the poorest regions of the world. While large language models (LLMs) have shown promise for medical question answering, there is limited work focused on tropical and infectious disease-specific explorations. We introduce TRINDs, a dataset of 52 tropical and infectious diseases with demographic and semantic clinical and consumer augmentations. We evaluate various context and counterfactual locations to understand their influence on LLM performance. Results show that LLMs perform best when provided with contextual information such as demographics, location, and symptoms. We also develop TRINDs-LM, a tool that enables users to enter symptoms and contextual information to receive a most likely diagnosis. In addition to the LLM evaluations, we also conducted a human expert baseline study to assess the accuracy of human experts in diagnosing tropical and infectious diseases with 7 medical and public health experts. This work demonstrates methods for creating and evaluating datasets for testing and optimizing LLMs, and the use of a tool that could improve digital diagnosis and tracking of NTDs.
View details
TRINDs: Assessing the Diagnostic Capabilities of Large Language Models for Tropical and Infectious Diseases
Nenad Tomašev
Chintan Ghate
Oluwatosin Akande
Geoffrey Siwo
Steve Adudans
Sylvanus Aitkins
Lynda Osadebe
Eric Ndombi
Odianosen Ehiakhamen
2024
Preview abstract
Neglected tropical diseases (NTDs) and infectious diseases disproportionately affect the poorest regions of the world. While large language models (LLMs) have shown promise for medical question answering, there is limited work focused on tropical and infectious disease-specific explorations. We introduce TRINDs, a dataset of 52 tropical and infectious diseases with demographic and semantic clinical and consumer augmentations. We evaluate various context and counterfactual locations to understand their influence on LLM performance. Results show that LLMs perform best when provided with contextual information such as demographics, location, and symptoms. We also develop TRINDs-LM, a tool that enables users to enter symptoms and contextual information to receive a most likely diagnosis. In addition to the LLM evaluations, we also conducted a human expert baseline study to assess the accuracy of human experts in diagnosing tropical and infectious diseases with 7 medical and public health experts. This work demonstrates methods for creating and evaluating datasets for testing and optimizing LLMs, and the use of a tool that could improve digital diagnosis and tracking of NTDs.
View details
A Toolbox for Surfacing Health Equity Harms and Biases in Large Language Models
Heather Cole-Lewis
Nenad Tomašev
Liam McCoy
Leo Anthony Celi
Alanna Walton
Chirag Nagpal
Akeiylah DeWitt
Philip Mansfield
Sushant Prakash
Joelle Barral
Ivor Horn
Karan Singhal
Nature Medicine (2024)
Preview abstract
Large language models (LLMs) hold promise to serve complex health information needs but also have the potential to introduce harm and exacerbate health disparities. Reliably evaluating equity-related model failures is a critical step toward developing systems that promote health equity. We present resources and methodologies for surfacing biases with potential to precipitate equity-related harms in long-form, LLM-generated answers to medical questions and conduct a large-scale empirical case study with the Med-PaLM 2 LLM. Our contributions include a multifactorial framework for human assessment of LLM-generated answers for biases and EquityMedQA, a collection of seven datasets enriched for adversarial queries. Both our human assessment framework and our dataset design process are grounded in an iterative participatory approach and review of Med-PaLM 2 answers. Through our empirical study, we find that our approach surfaces biases that may be missed by narrower evaluation approaches. Our experience underscores the importance of using diverse assessment methodologies and involving raters of varying backgrounds and expertise. While our approach is not sufficient to holistically assess whether the deployment of an artificial intelligence (AI) system promotes equitable health outcomes, we hope that it can be leveraged and built upon toward a shared goal of LLMs that promote accessible and equitable healthcare.
View details
Nteasee: A qualitative study of expert and general population perspectives on deploying AI for health in African countries
Iskandar Haykel
Kerrie Kauer
Florence Ofori
Tousif Ahmad
2024
Preview abstract
Background: Artificial Intelligence for health has the potential to significantly change and improve healthcare. However in most African countries identifying culturally and contextually attuned approaches for deploying these solutions is not well understood. To bridge this gap, we conduct a qualitative study to investigate the best practices, fairness indicators and potential biases to mitigate when deploying AI for health in African countries, as well as explore opportunities where artificial intelligence could make a positive impact in health.
Methods: We used a mixed methods approach combining in-depth interviews (IDIs) and surveys. We conduct 1.5-2 hour long IDIs with 50 experts in health, policy and AI across 17 countries, and through an inductive approach we conduct a qualitative thematic analysis on expert IDI responses. We administer a blinded 30-minute survey with thought-cases to 672 general population participants across 5 countries in Africa (Ghana, South Africa, Rwanda, Kenya and Nigeria), and analyze responses on quantitative scales, statistically comparing responses by country, age, gender, and level of familiarity with AI. We thematically summarize open-ended responses from surveys.
Results and Conclusion: Our results find generally positive attitudes, high levels of trust, accompanied by moderate levels of concern among general population participants for AI usage for health in Africa. This contrasts with expert responses, where major themes revolved around trust/mistrust, AI ethics concerns, and systemic barriers to overcome, among others. This work presents the first-of-its-kind qualitative research study of the potential of AI for health in Africa with perspectives from both experts and the general population. We hope that this work guides policy makers and drives home the need for education and the inclusion of general population perspectives in decision-making around AI usage.
View details
The Case for Globalizing Fairness: A Mixed Methods Study on the Perceptions of Colonialism, AI and Health in Africa
Iskandar Haykel
Chirag Nagpal
Aisha Walcott-Bryant
Sanmi Koyejo
2024
Preview abstract
With growing machine learning (ML) and large language model applications in healthcare, there have been calls for fairness in ML to understand and mitigate ethical concerns these systems may pose. Fairness has implications for health in Africa, which already has inequitable power imbalances between the Global North and South. This paper seeks to explore fairness for global health, with Africa as a case study.
We conduct a scoping review to propose fairness attributes for consideration in the African context and delineate where they may come into play in different ML-enabled medical modalities. We then conduct qualitative research studies with 625 general population study participants in 5 countries in Africa and 28 experts in ML, Health, and/or policy focussed on Africa to obtain feedback on the proposed attributes. We delve specifically into understanding the interplay between AI, health and colonialism.
Our findings demonstrate that among experts there is a general mistrust that technologies that are solely developed by former colonizers can benefit Africans, and that associated resource constraints due to pre-existing economic and infrastructure inequities can be linked to colonialism. General population survey responses found about an average of 40% of people associate an undercurrent of colonialism to AI and this was most dominant amongst participants from South Africa. However the majority of the general population participants surveyed did not think there was a direct link between AI and colonialism.Colonial history, country of origin, National income level were specific axes of disparities that participants felt would cause an AI tool to be biased
This work serves as a basis for policy development around Artificial Intelligence for health in Africa and can be expanded to other regions.
View details
Preview abstract
As machine learning (ML) systems see far-reaching applications in healthcare, there have been calls for fairness in machine learning to understand and mitigate ethical concerns these systems may pose. Fairness has thus far mostly been defined from a Western lens, and has implications for global health in Africa, which already has inequitable power imbalances between the Global North and South. This paper seeks to explore fairness for global health, with Africa as a case study. We propose fairness attributes for consideration in the African context and delineate where they may come into play in different ML-enabled medical modalities. This serves as a basis and call for action for furthering research into fairness in global health.
View details