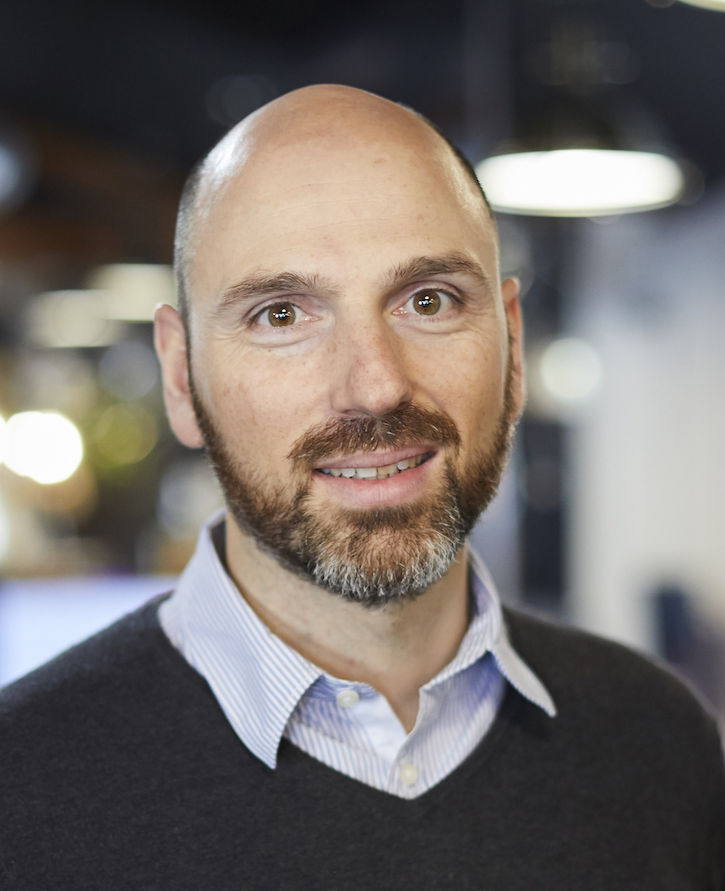
Olivier Bousquet
Olivier received his PhD in Machine Learning from Ecole Polytechnique, France in 2002. He was then a researcher at the Max Planck Institute in Tuebingen, working on Machine Learning and in particular Statistical Learning Theory and Kernel Methods. In 2004 he joined a startup company where he lead a research team and developed ML software for predicting manufacturing quality.
Olivier joined Google Zurich in 2007 and contributed to many aspects of the search engine, in particular leading an engineering team working on Language Understanding and the Knowledge Graph. In 2016, he joined the Research team and is now working on Deep Learning and Language Understanding, leading the Brain teams in Zurich and Paris.
His research interests include Learning with limited supervision (Semi-supervised or Unsupervised), AutoML (automation of Deep Learning), Learning of world representations and world knowledge.
Research Areas
Authored Publications
Sort By
Fine-Grained Distribution-Dependent Learning Curves
Jonathan Shafer
Shay Moran
Steve Hanneke
Proceedings of Thirty Sixth Conference on Learning Theory (COLT), PMLR 195:5890-5924, 2023. (2023)
Preview abstract
Learning curves plot the expected error of a learning algorithm as a function of the number of labeled input samples. They are widely used by machine learning practitioners as a measure of an algorithm's performance, but classic PAC learning theory cannot explain their behaviour. In this paper we introduce a new combinatorial characterization called the VCL dimension that improves and refines the recent results of Bousquet et al. (2021). Our characterization sheds new light on the structure of learning curves by providing fine-grained bounds, and showing that for classes with finite VCL, the rate of decay can be decomposed into a linear component that depends only on the hypothesis class and an exponential component that depends also on the target distribution. In particular, the finer nuance of the VCL dimension implies lower bounds that are quantitatively stronger than the bounds of Bousquet et al. (2021) and qualitatively stronger than classic 'no free lunch' lower bounds. The VCL characterization solves an open problem studied by Antos and Lugosi (1998), who asked in what cases such lower bounds exist. As a corollary, we recover their lower bound for half-spaces in ℝd, and we do so in a principled way that should be applicable to other cases as well. Finally, to provide another viewpoint on our work and how it compares to traditional PAC learning bounds, we also present an alternative formulation of our results in a language that is closer to the PAC setting.
View details
Preview abstract
The amount of training-data is one of the key factors which determines the generalization capacity of learning algorithms. Intuitively, one expects the error rate to decrease as the amount of training-data increases. Perhaps surprisingly, natural attempts to formalize this intuition give rise to interesting and challenging mathematical questions. For example, in their classical book on pattern recognition, Devroye, Gyorfi, and Lugosi (1996) ask whether there exists a monotone Bayes-consistent algorithm. This question remained open for over 25 years, until recently Pestov (2021) resolved it for binary classification, using an intricate construction of a monotone Bayes-consistent algorithm.
We derive a general result in multiclass classification, showing that every learning algorithm A can be transformed to a monotone one with similar performance. Further, the transformation is efficient and only uses a black-box oracle access to A. This demonstrates that one can provably avoid non-monotonic behaviour without compromising performance, thus answering questions asked by Devroye, Gyorfi, and Lugosi (1996), Viering, Mey, and Loog (2019), Viering and Loog (2021), and by Mhammedi (2021).
Our general transformation readily implies monotone learners in a variety of contexts: for example, Pestov’s result follows by applying it on any Bayes-consistent algorithm (e.g., k-Nearest-Neighbours). In fact, our transformation extends Pestov’s result to classification tasks with an arbitrary number of labels. This is in contrast with Pestov’s work which is tailored to binary classification.
In addition, we provide uniform bounds on the error of the monotone algorithm. This makes our transformation applicable in distribution-free settings. For example, in PAC learning it implies that every learnable class admits a monotone PAC learner. This resolves questions asked by Viering, Mey, and Loog (2019); Viering and Loog (2021); Mhammedi (2021).
View details
Differentially-Private Bayes Consistency
Aryeh Kontorovich
Shay Moran
Menachem Sadigurschi
Archive, Archive, Archive
Preview abstract
We construct a universally Bayes consistent learning rule
which satisfies differential privacy (DP).
We first handle the setting of binary classification
and then extend our rule to the more
general setting of density estimation (with respect to the total variation metric).
The existence of a universally consistent DP learner
reveals a stark difference with the distribution-free PAC model.
Indeed, in the latter DP learning is extremely limited:
even one-dimensional linear classifiers
are not privately learnable in this stringent model.
Our result thus demonstrates that by allowing
the learning rate to depend on the target distribution,
one can circumvent the above-mentioned impossibility result
and in fact learn \emph{arbitrary} distributions by a single DP algorithm.
As an application, we prove that any VC class can be privately learned in a semi-supervised
setting with a near-optimal \emph{labelled} sample complexity of $\tilde O(d/\eps)$ labeled examples
(and with an unlabeled sample complexity that can depend on the target distribution).
View details
Evaluating Generative Models using Divergence Frontiers
Josip Djolonga
Marco Cuturi
Sylvain Gelly
International Conference on Artificial Intelligence and Statistics (2020)
Preview abstract
Despite the tremendous progress in the estimation of generative models, the development of tools for diagnosing their failures and assessing their performance has advanced at a much slower pace. Very recent developments have investigated metrics that quantify which parts of the true distribution is well modeled, and, on the contrary, what the model fails to capture, akin to precision and recall in information retrieval. In this paper we present a general evaluation framework for generative models that measures the trade-off between precision and recall using R\'enyi divergences. Our framework provides a novel perspective on existing techniques and extends them to more general domains.
As a key advantage, it allows for efficient algorithms that are directly applicable to continuous distributions directly without discretization. We further showcase the proposed techniques on a set of image synthesis models.
View details
Measuring Compositional Generalization: A Comprehensive Method on Realistic Data
Nathanael Schärli
Nathan Scales
Hylke Buisman
Daniel Furrer
Nikola Momchev
Danila Sinopalnikov
Lukasz Stafiniak
Tibor Tihon
Dmitry Tsarkov
Marc van Zee
ICLR (2020)
Preview abstract
State-of-the-art machine learning methods exhibit limited compositional generalization. At the same time, there is a lack of realistic benchmarks that comprehensively measure this ability, which makes it challenging to find and evaluate improvements. We introduce a novel method to systematically construct such benchmarks by maximizing compound divergence while guaranteeing a small atom divergence between train and test sets, and we quantitatively compare this method to other approaches for creating compositional generalization benchmarks. We present a large and realistic natural language question answering dataset that is constructed according to this method, and we use it to analyze the compositional generalization ability of three machine learning architectures. We find that they fail to generalize compositionally and that there is a surprisingly strong negative correlation between compound divergence and accuracy. We also demonstrate how our method can be used to create new compositionality benchmarks on top of the existing SCAN dataset, which confirms these findings.
View details
What Do Neural Networks Learn When Trained With Random Labels?
Hartmut Maennel
Robert J. N. Baldock
Sylvain Gelly
NeurIPS 2020
Preview abstract
We study deep neural networks (DNNs) trained on natural image data with entirely random labels. Despite its popularity in the literature, where it is often used to study memorization, generalization, and other phenomena, little is known about what DNNs learn in this setting. In this paper, we show analytically for convolutional and fully connected networks that an alignment between the principal components of network parameters and data takes place when training with random labels. We study this alignment effect by investigating neural networks pre-trained on randomly labelled image data and subsequently fine-tuned on disjoint datasets with random or real labels. We show how this alignment produces a positive transfer: networks pre-trained with random labels train faster downstream compared to training from scratch even after accounting for simple effects, such as weight scaling. We analyze how competing effects, such as specialization at later layers, may hide the positive transfer. These effects are studied in several network architectures, including VGG16 and ResNet18, on CIFAR10 and ImageNet.
View details
Preview abstract
The classical PAC sample complexity bounds are stated for any Empirical Risk Minimizer (ERM) and contain an extra multiplicative logarithmic is known to be necessary for ERM in general. It has been recently shown by Hanneke, 2016 that the optimal sample complexity of PAC learning for any VC class C is achieved by a particular improper learning algorithm, which outputs a specific majority-vote of hypotheses in C. This leaves the question of when this bound can be achieved by proper learning algorithms, which are restricted to always output a hypothesis from C. In this paper we aim to characterize the classes for which the optimal sample complexity can be achieved by a proper learning algorithm. We identify that these classes can be characterized by the dual Helly number, which is a combinatorial parameter that arises in discrete geometry and abstract convexity. In particular, under general conditions on C, we show that the dual Helly number is bounded if and only if there is a proper learner that obtains the optimal joint dependence on ε and δ. As further implications of our techniques we resolve a long-standing open problem posed by Vapnik and Chervonenkis, 1974 on the performance of Support Vector Machine by proving that the sample complexity of SVM in the realizable case is Θ(n/ε+1/ε log1/δ). This gives the first optimal PAC bound for Halfspaces achieved by a proper and computationally efficient learning algorithm.
View details
Preview abstract
We consider the variance of a function of $n$ independent random variables and provide inequalities that generalize previous results obtained for i.i.d. random variables. In particular we obtain upper and lower bounds on the variance based on iterated Jackknife statistics that can be considered as higher-order Efron-Stein inequalities.
View details
Practical and Consistent Estimation of f-Divergences
Paul Rubenstein
Josip Djolonga
Carlos Riquelme
Submission to Neurips 2019. (2019) (to appear)
Preview abstract
The estimation of an f-divergence between two probability distributions based on samples is a fundamental problem in statistics and machine learning. Most works study this problem under very weak assumptions, in which case it is provably hard. We consider the case of stronger structural assumptions that are commonly satisfied in modern machine learning, including representation learning and generative modelling with autoencoder architectures. Under these assumptions we propose and study an estimator that can be easily implemented, works well in high dimensions, and enjoys faster rates of convergence. We verify the behavior of our estimator empirically in both synthetic and real-data experiments.
View details
When can unlabeled data improve the learning rate?
Christina Göpfert
Shai Ben-David
Sylvain Gelly
Ruth Urner
COLT 2019
Preview abstract
In semi-supervised classification, one is given access both to labeled and unlabeled data. As unlabeled data is typically cheaper to acquire than labeled data, this setup becomes advantageous as soon as one can exploit the unlabeled data in order to produce a better classifier than with labeled data alone. However, the conditions under which such an improvement is possible are not fully understood yet. Our analysis focuses on improvements in the {\em minimax} learning rate in terms of the number of labeled examples (with the number of unlabeled examples being allowed to depend on the number of labeled ones).
We argue that for such improvements to be realistic and indisputable, certain specific conditions should be satisfied and previous analyses have failed to meet those conditions. We then demonstrate simple toy examples where these conditions can be met, in particular showing rate changes from $1/\sqrt{\l}$ to $e^{-c\l}$ and $1/\sqrt{\l}$ to $1/\l$. These results allow us to better understand what is and isn't possible in semi-supervised learning.
View details