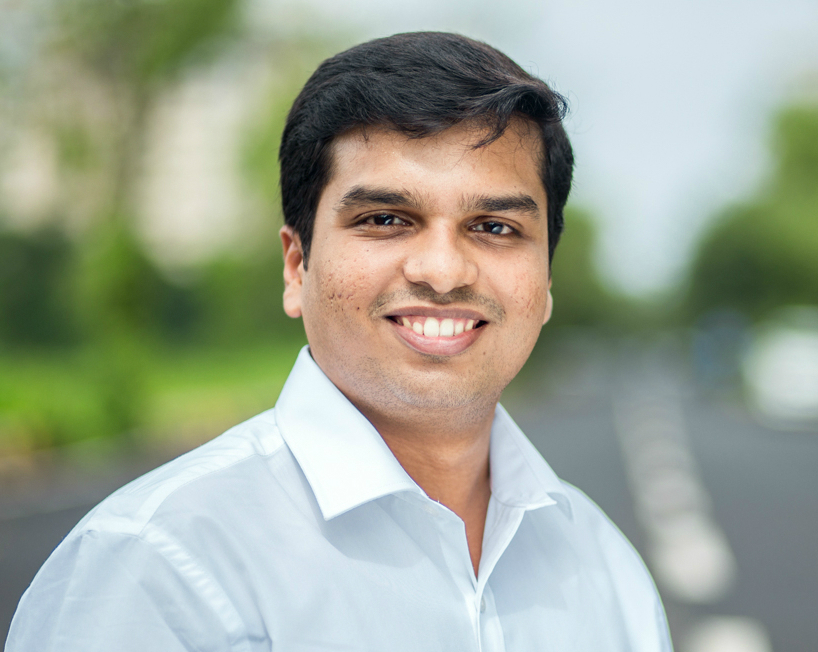
Sujay Kakarmath
Sujay Kakarmath is a Product Manager at Google Research, specializing in health AI projects. With a background as a physician and experience in global public health and digital health research, Sujay focuses on translating AI advancements into healthcare innovations. His recent work includes predicting cardiovascular risk using smartphone signals and developing a bioacoustic model to identify biomarkers for respiratory conditions such as tuberculosis and childhood pneumonia
Research Areas
Authored Publications
Sort By
LLM-based Lossless Text Simplification and its Effect on User Comprehension and Cognitive Load
Theo Guidroz
Diego Ardila
Jimmy Li
Adam Mansour
Paul Jhun
Nina Gonzalez
Xiang Ji
Mike Sanchez
Miguel Ángel Garrido
Divyansh Choudhary
Jay Hartford
Georgina Xu
Henry Serrano
Yifan Wang
Jeff Shaffer
Eric (Yifan) Cao
Sho Fujiwara
Peggy Bui
arXiv (2025)
Preview abstract
Information on the web, such as scientific publications and Wikipedia, often surpasses users' reading level. To help address this, we used a self-refinement approach to develop a LLM capability for minimally lossy text simplification. To validate our approach, we conducted a randomized study involving 4563 participants and 31 texts spanning 6 broad subject areas: PubMed (biomedical scientific articles), biology, law, finance, literature/philosophy, and aerospace/computer science. Participants were randomized to viewing original or simplified texts in a subject area, and answered multiple-choice questions (MCQs) that tested their comprehension of the text. The participants were also asked to provide qualitative feedback such as task difficulty. Our results indicate that participants who read the simplified text answered more MCQs correctly than their counterparts who read the original text (3.9% absolute increase, p<0.05). This gain was most striking with PubMed (14.6%), while more moderate gains were observed for finance (5.5%), aerospace/computer science (3.8%) domains, and legal (3.5%). Notably, the results were robust to whether participants could refer back to the text while answering MCQs. The absolute accuracy decreased by up to ~9% for both original and simplified setups where participants could not refer back to the text, but the ~4% overall improvement persisted. Finally, participants' self-reported perceived ease based on a simplified NASA Task Load Index was greater for those who read the simplified text (absolute change on a 5-point scale 0.33, p<0.05). This randomized study, involving an order of magnitude more participants than prior works, demonstrates the potential of LLMs to make complex information easier to understand. Our work aims to enable a broader audience to better learn and make use of expert knowledge available on the web, improving information accessibility.
View details
Predicting Cardiovascular Disease Risk using Photoplethysmography and Deep Learning
Sebastien Baur
Mayank Daswani
Christina Chen
Mariam Jabara
Babak Behsaz
Shravya Shetty
Goodarz Danaei
Diego Ardila
PLOS Global Public Health, 4(6) (2024), e0003204
Preview abstract
Cardiovascular diseases (CVDs) are responsible for a large proportion of premature deaths in low- and middle-income countries. Early CVD detection and intervention is critical in these populations, yet many existing CVD risk scores require a physical examination or lab measurements, which can be challenging in such health systems due to limited accessibility. We investigated the potential to use photoplethysmography (PPG), a sensing technology available on most smartphones that can potentially enable large-scale screening at low cost, for CVD risk prediction. We developed a deep learning PPG-based CVD risk score (DLS) to predict the probability of having major adverse cardiovascular events (MACE: non-fatal myocardial infarction, stroke, and cardiovascular death) within ten years, given only age, sex, smoking status and PPG as predictors. We compare the DLS with the office-based refit-WHO score, which adopts the shared predictors from WHO and Globorisk scores (age, sex, smoking status, height, weight and systolic blood pressure) but refitted on the UK Biobank (UKB) cohort. All models were trained on a development dataset (141,509 participants) and evaluated on a geographically separate test (54,856 participants) dataset, both from UKB. DLS’s C-statistic (71.1%, 95% CI 69.9–72.4) is non-inferior to office-based refit-WHO score (70.9%, 95% CI 69.7–72.2; non-inferiority margin of 2.5%, p<0.01) in the test dataset. The calibration of the DLS is satisfactory, with a 1.8% mean absolute calibration error. Adding DLS features to the office-based score increases the C-statistic by 1.0% (95% CI 0.6–1.4). DLS predicts ten-year MACE risk comparable with the office-based refit-WHO score. Interpretability analyses suggest that the DLS-extracted features are related to PPG waveform morphology and are independent of heart rate. Our study provides a proof-of-concept and suggests the potential of a PPG-based approach strategies for community-based primary prevention in resource-limited regions.
View details