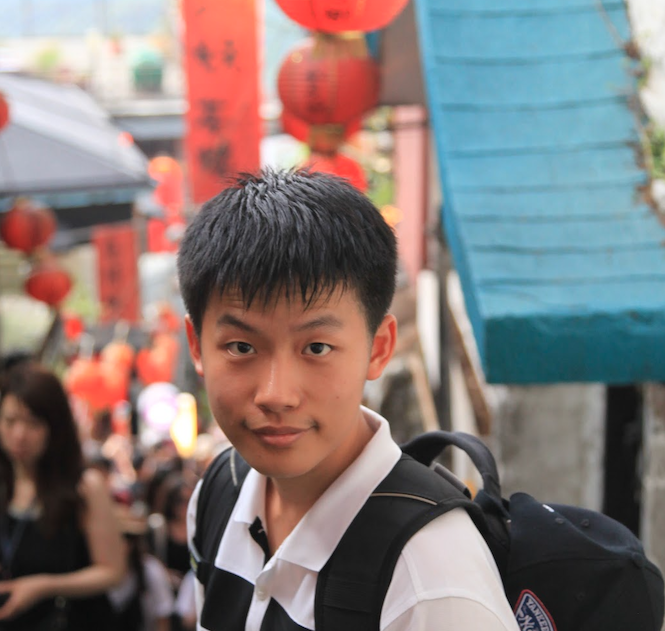
Yuan Deng
Yuan Deng is a Research Scientist at Google Research New York City. His research is broadly situated at the interface between economics and computer science (aka. algorithmic game theory), mainly including dynamic mechanism design (how to design auctions for online advertisement markets) and learning in economic environments (e.g. online pricing for strategic agents and/or learning agents).
Authored Publications
Sort By
Auto-bidding and Auctions in Online Advertising: A Survey
Ashwinkumar Badanidiyuru Varadaraja
Christopher Liaw
Haihao (Sean) Lu
Andres Perlroth
Georgios Piliouras
Ariel Schvartzman
Kelly Spendlove
Hanrui Zhang
Mingfei Zhao
ACM SIGecom Exchanges, 22 (2024)
Preview abstract
In this survey, we summarize recent developments in research fueled by the growing adoption of automated bidding strategies in online advertising. We explore the challenges and opportunities that have arisen as markets embrace this autobidding and cover a range of topics in this area, including bidding algorithms, equilibrium analysis and efficiency of common auction formats, and optimal auction design.
View details
Individual Welfare Guarantees in the Autobidding World with Machine-learned Advice
Negin Golrezaei
Patrick Jaillet
Jason Cheuk Nam Liang
Proceedings of the ACM on Web Conference 2024, 267–275
Preview abstract
Online advertising channels commonly focus on maximizing total advertiser welfare to enhance channel health, and previous literature has studied augmenting ad auctions with machine learning predictions on advertiser values (also known asmachine-learned advice ) to improve total welfare. Yet, such improvements could come at the cost of individual bidders' welfare and do not shed light on how particular advertiser bidding strategies impact welfare. Motivated by this, we present an analysis on an individual bidder's welfare loss in the autobidding world for auctions with and without machine-learned advice, and also uncover how advertiser strategies relate to such losses. In particular, we demonstrate how ad platforms can utilize ML advice to improve welfare guarantee on the aggregate and individual bidder level by setting ML advice as personalized reserve prices when the platform consists ofautobidders who maximize value while respecting a return on ad spend (ROAS) constraint. Under parallel VCG auctions with such ML advice-based reserves, we present a worst-case welfare lower-bound guarantee for an individual autobidder, and show that the lower-bound guarantee is positively correlated with ML advice quality as well as the scale of bids induced by the autobidder's bidding strategies. Further, we show that no truthful, and possibly randomized mechanism with anonymous allocations can achieve universally better individual welfare guarantees than VCG, in the presence of personalized reserves based on ML-advice of equal quality. Moreover, we extend our individual welfare guarantee results to generalized first price (GFP) and generalized second price (GSP) auctions. Finally, we present numerical studies using semi-synthetic data derived from ad auction logs of a search ad platform to showcase improvements in individual welfare when setting personalized reserve prices with ML-advice.
View details
Non-uniform Bid-scaling and Equilibria for Different Auctions: An Empirical Study
Proceedings of the ACM on Web Conference 2024, 256–266
Preview abstract
In recent years, the growing adoption of autobidding has motivated the study of auction design with value-maximizing auto-bidders. It is known that under mild assumptions, uniform bid-scaling is an optimal bidding strategy in truthful auctions, e.g., Vickrey-Clarke-Groves auction (VCG), and the price of anarchy for VCG is 2. However, for other auction formats like First-Price Auction (FPA) and Generalized Second-Price auction (GSP), uniform bid-scaling may not be an optimal bidding strategy, and bidders have incentives to deviate to adopt strategies with non-uniform bid-scaling. Moreover, FPA can achieve optimal welfare if restricted to uniform bid-scaling, while its price of anarchy becomes 2 when non-uniform bid-scaling strategies are allowed.
All these price of anarchy results have been focused on welfare approximation in the worst-case scenarios. To complement theoretical understandings, we empirically study how different auction formats (FPA, GSP, VCG) with different levels of non-uniform bid-scaling perform in an autobidding world with a synthetic dataset for auctions. Our empirical findings include: * For both uniform bid-scaling and non-uniform bid-scaling, FPA is better than GSP and GSP is better than VCG in terms of both welfare and profit; * A higher level of non-uniform bid-scaling leads to lower welfare performance in both FPA and GSP, while different levels of non-uniform bid-scaling have no effect in VCG. Our methodology of synthetic data generation may be of independent interest.
View details
Preview abstract
The recent increasing adoption of autobidding has inspired the growing interest in analyzing the performance of classic mechanism with value-maximizing auto-bidders both theoretically and empirically. It is known that optimal welfare can be obtained in first-price auctions if auto-bidders are restricted to uniform bid-scaling and the price of anarchy is $2$ when non-uniform bid-scaling strategies are allowed.
In this paper, we provide a fine-grained price of anarchy analysis for non-uniform bid-scaling strategies in first-price auctions, demonstrating the reason why more powerful (individual) non-uniform bid-scaling strategies may lead to worse (aggregated) performance in social welfare. Our theoretical results match recent empirical findings that a higher level of non-uniform bid-scaling leads to lower welfare performance in first-price auctions.
View details
Preview abstract
We study the price of anarchy of the first-price auction in the autobidding world, where bidders can be either utility maximizers (i.e., traditional bidders) or value maximizers (i.e., autobidders). We show that with autobidders only, the price of anarchy of the first-price auction is 1/2, and with both kinds of bidders, the price of anarchy degrades to about 0.457 (the precise number is given by an optimization). These results complement the recent result by Jin and Lu [2022] showing that the price of anarchy of the first-price auction with traditional bidders only is $1−1/e^2$. We further investigate a setting where the seller can utilize machine-learned advice to improve the efficiency of the auctions. There, we show that as the accuracy of the advice increases, the price of anarchy improves smoothly from about 0.457 to 1.
View details
Optimal Mechanisms for a Value Maximizer: The Futility of Screening Targets
Proceedings of the 25th ACM Conference on Economics and Computation (EC) (2024)
Preview abstract
Motivated by the increased adoption of autobidding algorithms in internet advertising markets, we study the design of optimal mechanisms for selling items to a value-maximizing buyer with a return-on-spend constraint. The buyer's values and target ratio in the return-on-spend constraint are private. We restrict attention to deterministic sequential screening mechanisms that can be implemented as a menu of prices paid for purchasing an item or not. The main result of this paper is to provide a characterization of an optimal mechanism. Surprisingly, we show that the optimal mechanism does not require target screening, i.e., offering a single pair of prices is optimal for the seller. The optimal mechanism is a subsidized posted price that provides a subsidy to the buyer to encourage participation and then charges a fixed unit price for each item sold. The seller's problem is a challenging non-linear mechanism design problem, and a key technical contribution of our work is to provide a novel approach to analyze non-linear pricing contracts.
View details
Efficiency of the Generalized Second-Price Auction for Value Maximizers
Hanrui Zhang
Proceedings of the ACM on Web Conference 2024, 46–56
Preview abstract
We study the price of anarchy of the generalized second-price auction where bidders are value maximizers (i.e., autobidders). We show that in general the price of anarchy can be as bad as 0. For comparison, the price of anarchy of running VCG is 1/2 in the autobidding world. We further show a fined-grained price of anarchy with respect to the discount factors (i.e., the ratios of click probabilities between lower slots and the highest slot in each auction) in the generalized second-price auction, which highlights the qualitative relation between the smoothness of the discount factors and the efficiency of the generalized second-price auction.
View details
Autobidding Auctions in the Presence of User Costs
Hanrui Zhang
Proceedings of the ACM Web Conference 2023, pp. 3428-3435
Preview abstract
Although costs are prevalent in ad auctions, not many auction theory works study auction design in the presence of cost in the classic settings. One reason is that most auctions design in the setting without cost directly generalize to the setting with cost when the bidders maximizing quasi-linear utility.
However, as auto-bidding becomes a major choice of advertisers in online advertising, the distinction from the underlying behavior model often leads to different solutions of many well-studied auctions. In the context of ad auctions with cost, VCG achieves optimal welfare with quasi-linear utility maximizing bidders, while has 0 welfare approximation guarantee with value maximizing bidders who follow the optimization behind common auto-bidding algorithms.
In this paper, we prove that the approximation welfare guarantee of VCG auction can be significantly improved by a minimal change --- introducing cost multipliers. We note that one can use either one multiplier per auction or one multiplier per bidder, but one global multiplier across all auctions and bidders does not work. Finally, to echo with our theoretical results, we conduct empirical evaluations using semi-synthetic data derived from real auction data of a major advertising platform.
View details
Optimal Pricing Schemes for an Impatient Buyer
Kangning Wang
Proceedings of the Annual ACM-SIAM Symposium on Discrete Algorithms (2023), pp. 382-398
Preview abstract
A patient seller aims to sell a good to an impatient buyer (i.e., one who discounts utility over time).The buyer will remain in the market for a period of time T , and her private value is drawn from a publicly known distribution. What is the revenue-optimal pricing-curve (sequence of (price, time) pairs) for the seller? Is randomization of help here? Is the revenue-optimal pricing-curve computable in polynomial time? We answer these questions in this paper. We give an efficient algorithm for computing the revenue-optimal pricing curve. We show that pricing curves, that post a price at each point of time and let the buyer pick her utility maximizing time to buy, are revenue-optimal among a much broader class of sequential lottery mechanisms: namely, mechanisms that allow the seller to post a menu of lotteries at each point of time cannot get any higher revenue than pricing curves. We also show that the even broader class of mechanisms that allow the menu of lotteries to be adaptively set, can earn strictly higher revenue than that of pricing curves, and the revenue gap can be as big as the support size of the buyer’s value distribution.
View details
Multi-channel Autobidding with Budget and ROI Constraints
Negin Golrezaei
Patrick Jaillet
Jason Cheuk Nam Liang
Proceedings of the 40th International Conference on Machine Learning (2023), 7617–7644
Preview abstract
In digital online advertising, advertisers procure ad impressions simultaneously on multiple platforms, or so-called channels, such as Google Ads, Meta Ads Manager, etc., each of which consists of numerous ad auctions. We study how an advertiser maximizes total conversion (e.g. ad clicks) while satisfying aggregate return-on-investment (ROI) and budget constraints across all channels. In practice, an advertiser does not have control over, and thus cannot globally optimize, which individual ad auctions she participates in for each channel, and instead authorizes a channel to procure impressions on her behalf: the advertiser can only utilize two levers on each channel, namely setting a per-channel budget and per-channel target ROI. In this work, we first analyze the effectiveness of each of these levers for solving the advertiser's global multi-channel problem. We show that when an advertiser only optimizes over per-channel ROIs, her total conversion can be arbitrarily worse than what she could have obtained in the global problem. Further, we show that the advertiser can achieve the global optimal conversion when she only optimizes over per-channel budgets. In light of this finding, under a bandit feedback setting that mimics real-world scenarios where advertisers have limited information on ad auctions in each channels and how channels procure ads, we present an efficient learning algorithm that produces per-channel budgets whose resulting conversion approximates that of the global optimal problem. Finally, we argue that all our results hold for both single-item and multi-item auctions from which channels procure impressions on advertisers' behalf.
View details