Publications
Our teams aspire to make discoveries that impact everyone, and core to our approach is sharing our research and tools to fuel progress in the field.
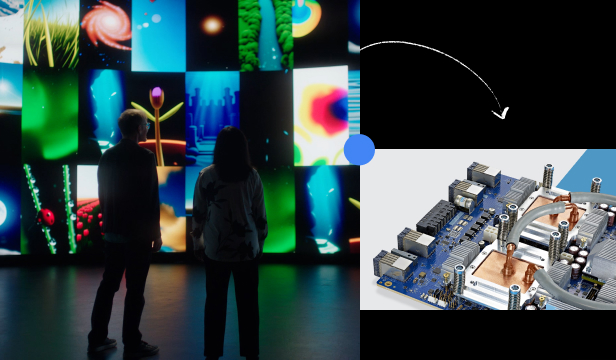
Our teams aspire to make discoveries that impact everyone, and core to our approach is sharing our research and tools to fuel progress in the field.
Sort By
1 - 15 of 19 publications
Preview abstract
Floods are one of the most common natural disasters, with a disproportionate impact in developing countries that often lack dense streamflow gauge networks. Accurate and timely warnings are critical for mitigating flood risks, but hydrological simulation models typically must be calibrated to long data records in each watershed. Here we show that AI-based forecasting achieves reliability in predicting extreme riverine events in ungauged watersheds at up to a 5-day lead time that is similar to or better than the reliability of nowcasts (0-day lead time) from a current state of the art global modeling system (the Copernicus Emergency Management Service Global Flood Awareness System). Additionally, we achieve accuracies over 5-year return period events that are similar to or better than current accuracies over 1-year return period events. This means that AI can provide flood warnings earlier and over larger and more impactful events in ungauged basins. The model developed in this paper was incorporated into an operational early warning system that produces publicly available (free and open) forecasts in real time in over 80 countries. This work highlights a need for increasing the availability of hydrological data to continue to improve global access to reliable flood warnings.
View details
An artificial neural network to estimate the foliar and ground cover input variables of the Rangeland Hydrology and Erosion Model
Mahmoud Saeedimoghaddam
David Goodrich
Mariano Hernandez
David Phillip Guertin
Loretta J. Metz
Guillermo Ponce-Campos
Haiyan Wei
Shea Burns
Sarah E. McCord
Mark A. Nearing
C. Jason Williams
Carrie-Ann Houdeshell
Mashrekur Rahman
Menberu B. Meles
Steve Barker
Journal of Hydrology (2024)
Preview abstract
Models like the Rangeland Hydrology and Erosion Model (RHEM) are useful for estimating soil erosion, however, they rely on input parameters that are sometimes difficult or expensive to measure. Specifically, RHEM requires information about foliar and ground cover fractions that generally must be measured in situ, which makes it difficult to use models like RHEM to produce erosion or soil risk maps for areas exceeding the size of a hillslope such as a large watershed. We previously developed a deep learning emulator of RHEM that has low computational expense and can, in principle, be run over large areas (e.g., over the continental US). In this paper, we develop a deep learning model to estimate the RHEM ground cover inputs from remote sensing time series, reducing the need for extensive field surveys to produce erosion maps. We achieve a prediction accuracy on hillslope runoff of r2=0.9, and on soil loss and sediment yield of r2 = 0.4 at 66,643 field locations within the US. We demonstrate how this approach can be used for mapping by developing runoff, soil loss, and sediment yield maps over a 1356 km2 region of interest in Nebraska.
View details
On the predictability of turbulent fluxes from land: PLUMBER2 MIP experimental description and preliminary results
Gab Abramowitz
Anna Ukkola
Sanaa Hobeichi
Jon Cranko Page
Mathew Lipson
Martin De Kauwe
Sam Green
Claire Brenner
Jonathan Frame
Martyn Clark
Martin Best
Peter Anthoni
Gabriele Arduini
Souhail Boussetta
Silvia Caldararu
Kyeungwoo Cho
Matthias Cuntz
David Fairbairn
Craig Ferguson
Hyungjun Kim
Yeonjoo Kim
Jürgen Knauer
David Lawrence
Xiangzhong Luo
Sergey Malyshev
Tomoko Nitta
Jerome Ogee
Keith Oleson
Catherine Ottlé
Phillipe Peylin
Patricia de Rosnay
Heather Rumbold
Bob Su
Nicolas Vuichard
Anthony Walker
Xiaoni Wang-Faivre
Yunfei Wang
Yijian Zeng
Hydrology and Earth Systems Sciences Discussions (2024)
Preview abstract
Accurate representation of the turbulent exchange of carbon, water, and heat between the land surface and the atmosphere is critical for modelling global energy, water, and carbon cycles, both in future climate projections and weather forecasts. We describe a Model Intercomparison Project (MIP) that compares the surface turbulent heat flux predictions of around 20 different land models provided with in-situ meteorological forcing, evaluated with measured surface fluxes using quality-controlled data from 170 eddy-covariance based flux tower sites.
Several out-of-sample empirical model predictions of site fluxes are used as benchmarks to quantify the degree to which land model performance could improve across a broad range of metrics. The performance discrepancy between empirical and physically-based model predictions also provides a potential pathway to understand sources of model error. Sites with unusual behaviour, complicated processes, poor data quality or uncommon flux magnitude will be more difficult to predict for both mechanistic and empirical models.
Results suggest that latent heat flux and net ecosystem exchange of CO2 are better predicted by land models than sensible heat flux, which at least conceptually would appear to have fewer physical processes controlling it. Land models that are implemented in Earth System Models also appear to perform notably better than stand alone ecosystem (including demographic) models, at least in terms of the fluxes examined here.
Flux tower data quality is also explored as an uncertainty source, with the difference between energy-balance corrected versus raw fluxes examined, as well as filtering for low wind speed periods. Land model performance does not appear to improve with energy-balance corrected data, and indeed some results raised questions about whether the correction process itself was appropriate. In both cases results were broadly consistent, with simple out-of-sample empirical models, including linear regression, comfortably outperforming mechanistic land models. The PLUMBER2 approach, and its openly-available data, enable precise isolation of the locations and conditions in which model developers can know that a given land model can improve, allowing information pathways and discrete parametrisations in models to be identified and targeted for model development.
View details
Uncertainty quantification in coupled wildfire-atmosphere simulations at scale
Paul Schwerdtner
Frederick Law
Cenk Gazen
Yi-fan Chen
Matthias Ihme
Benjamin Peherstorfer
PNAS Nexus (2024)
Preview abstract
Uncertainties in wildfire simulations pose a major challenge for making decisions about fire management, mitigation, and evacuations. However, ensemble calculations to quantify uncertainties are prohibitively expensive with high-fidelity models that are needed to capture today’s ever more intense and severe wildfires. This work shows that surrogate models trained on related data enable scaling multi-fidelity uncertainty quantification to high-fidelity wildfire simulations of unprecedented scale with billions of degrees of freedom. The key insight is that correlation is all that matters while bias is irrelevant for speeding up uncertainty quantification when surrogate models are combined with high-fidelity models in multi-fidelity approaches. This allows the surrogate models to be trained on abundantly available or cheaply generated related data samples that can be strongly biased as long as they are correlated to predictions of high-fidelity simulations. Numerical results with scenarios of the Tubbs 2017 wildfire demonstrate that surrogate models trained on related data make multi-fidelity uncertainty quantification in large-scale wildfire simulations practical by reducing the training time by several orders of magnitude from three months to under three hours and predicting the burned area at least twice as accurately compared to using high-fidelity simulations alone for a fixed computational budget. More generally, the results suggest that leveraging related data can greatly extend the scope of surrogate modeling, potentially benefiting other fields that require uncertainty quantification in computationally expensive high-fidelity simulations.
View details
QUANTITATIVE APPROACH FOR COORDINATION, AT SCALE, OF SIGNALIZED 2 INTERSECTION PAIRS
Jack Haddad
Nitzan Tur
Danny Veikherman
Eliav Buchnik
Shai Ferster
Tom Kalvari
Dan Karliner
Omer Litov
2024
Preview abstract
The coordination of signalized intersections in urban cities improves both traffic operations and environmental aspects. Traffic signal coordination has a long history, where the impact of offset on delays and emissions at signalized intersections have been investigated through simulations and a limited number of experimental findings. Coordinating intersections is often justified by specific engineering requirements and judgment. However, as a consequence, many intersections in cities remain uncoordinated.
In this paper, we examine the potential benefits of coordinating signalized intersections at scale. Unlike previous studies, our analysis is based on aggregated anonymized probe data analysis and does not need to explicitly model traffic-oriented issues such as queue spillback and platoon dispersion. We follow a decentralized approach by considering intersection pairs, i.e. a system of two signalized intersections which can be spatially coupled, but have different cycle lengths. We introduce a new method for coordinating those signalized intersections. The method first evaluates the effect of different offsets on vehicle travel times and emissions. Then, it coordinates the two intersections by setting a common cycle and finding the optimal offset that minimizes emissions and travel times. We present the analysis for several case studies from real intersections at Jakarta, Rio de Janeiro, Kolkata, and Haifa. Finally, we evaluated our method by implementing it in a real experimental study at Jakarta. We collaborated with the city to implement the optimal offset that we had determined, and we compared the results before and after coordination.
View details
Systematic Data Driven Detection of Unintentional Changes in Traffic Light Plans
Dan Karliner
Eliav Buchnik
Shai Ferster
Tom Kalvari
Omer Litov
Nitzan Tur
Danny Veikherman
Jack Haddad
2024
Preview abstract
Abstract—Traffic light plans determine the time allocated to each movement within an intersection. The plan has high influence on vehicle travel performance such as on the average delay time or the probability to stop in the intersection. Traffic engineers of a city control its traffic lights and can make changes in their plans to improve traffic performance. As it is not always easy to predict the impact of such changes, their potential impact can also be negative. We present an experimental study of real changes in traffic plans in 12 cities with a total of over 12000 intersections within a time period of over 40 days. We focus on changes of the cycle time of plans that highly impacted performance metrics such as delay. We compare the overall impact of such changes and dive into several of them through a careful analysis. To the best of our knowledge, our study is one of the largest in its scope among experimental studies of traffic conditions in recent years.
View details
Preview abstract
We develop a deep learning based convolutional-regression model that estimates the volumetric soil moisture content in the top ~5 cm. Input predictors include Sentinel-1 (active radar), Sentinel-2 (optical imagery), and SMAP (passive radar) as well as geophysical variables from SoilGrids and modelled soil moisture fields from GLDAS. The model was trained and evaluated on data from ~1300 in-situ sensors globally over the period 2015 - 2021 and obtained an average per-sensor correlation of 0.72 and ubRMSE of 0.0546. These results are benchmarked against 13 other soil moisture estimates at different locations, and an ablation study was used to identify important predictors.
View details
AI Increases Global Access to Reliable Flood Forecasts
Asher Metzger
Dana Weitzner
Frederik Kratzert
Guy Shalev
Martin Gauch
Sella Nevo
Shlomo Shenzis
Tadele Yednkachw Tekalign
Vusumuzi Dube
arXiv (2023)
Preview abstract
Floods are one of the most common natural disasters, with a disproportionate impact in developing countries that often lack dense streamflow gauge networks. Accurate and timely warnings are critical for mitigating flood risks, but hydrological simulation models typically must be calibrated to long data records in each watershed. Here we show that AI-based forecasting achieves reliability in predicting extreme riverine events in ungauged watersheds at up to a 5-day lead time that is similar to or better than the reliability of nowcasts (0-day lead time) from a current state of the art global modeling system (the Copernicus Emergency Management Service Global Flood Awareness System). Additionally, we achieve accuracies over 5-year return period events that are similar to or better than current accuracies over 1-year return period events. This means that AI can provide flood warnings earlier and over larger and more impactful events in ungauged basins. The model developed in this paper was incorporated into an operational early warning system that produces publicly available (free and open) forecasts in real time in over 80 countries. This work highlights a need for increasing the availability of hydrological data to continue to improve global access to reliable flood warnings.
View details
In Defense of Metrics: Metrics Sufficiently Encode Typical Human Preferences Regarding Hydrological Model Performance
Martin Gauch
Frederik Kratzert
Hoshin Gupta
Juliane Mai
Bryan A. Tolson
Sepp Hochreiter
Daniel Klotz
Water Resources Research, 59, e2022WR033918 (2023)
Preview abstract
Building accurate rainfall–runoff models is an integral part of hydrological science and practice. The variety of modeling goals and applications have led to a large suite of evaluation metrics for these models. Yet, hydrologists still put considerable trust into visual judgment, although it is unclear whether such judgment agrees or disagrees with existing quantitative metrics. In this study, we tasked 622 experts to compare and judge more than 14,000 pairs of hydrographs from 13 different models. Our results show that expert opinion broadly agrees with quantitative metrics and results in a clear preference for a Machine Learning model over traditional hydrological models. The expert opinions are, however, subject to significant amounts of inconsistency. Nevertheless, where experts agree, we can predict their opinion purely from quantitative metrics, which indicates that the metrics sufficiently encode human preferences in a small set of numbers. While there remains room for improvement of quantitative metrics, we suggest that the hydrologic community should reinforce their benchmarking efforts and put more trust in these metrics.
View details
Caravan - A global community dataset for large-sample hydrology
Frederik Kratzert
Nans Addor
Tyler Erickson
Martin Gauch
Lukas Gudmundsson
Daniel Klotz
Sella Nevo
Guy Shalev
Scientific Data, 10 (2023), pp. 61
Preview abstract
High-quality datasets are essential to support hydrological science and modeling. Several CAMELS (Catchment Attributes and Meteorology for Large-sample Studies) datasets exist for specific countries or regions, however these datasets lack standardization, which makes global studies difficult. This paper introduces a dataset called Caravan (a series of CAMELS) that standardizes and aggregates seven existing large-sample hydrology datasets. Caravan includes meteorological forcing data, streamflow data, and static catchment attributes (e.g., geophysical, sociological, climatological) for 6830 catchments. Most importantly, Caravan is both a dataset and open-source software that allows members of the hydrology community to extend the dataset to new locations by extracting forcing data and catchment attributes in the cloud. Our vision is for Caravan to democratize the creation and use of globally-standardized large-sample hydrology datasets. Caravan is a truly global open-source community resource.
View details
A Neural Encoder for Earthquake Rate Forecasting
Oleg Zlydenko
Brendan Meade
Alexandra Sharon Molchanov
Sella Nevo
Yohai bar Sinai
Scientific Reports (2023)
Preview abstract
Forecasting the timing of earthquakes is a long-standing challenge. Moreover, it is still debated how to formulate this problem in a useful manner, or to compare the predictive power of different models.
Here, we develop a versatile neural encoder of earthquake catalogs, and apply it to the fundamental problem of earthquake rate prediction, in the spatio-temporal point process framework. The epidemic
type aftershock sequence model (ETAS) effectively learns a small number of parameters to constrain assumed functional forms for the space and time relationships of earthquake sequences (e.g., Omori-Utsu law). Here we introduce learned spatial and temporal embeddings for point process earthquake forecast models that capture complex correlation structures. We demonstrate the generality of this neural representation as compared with ETAS model using train-test data splits and how it enables the incorporation of additional geophysical information. In rate prediction tasks, the generalized model shows > 4% improvement in information gain per earthquake and the simultaneous learning of anisotropic spatial structures analogous to fault traces. The trained network can be also used to perform short-term prediction tasks, showing similar improvement while providing a 1,000-fold reduction in run-time.
View details
On strictly enforced mass conservation constraints for modelling the Rainfall-Runoff process
Jonathan Frame
Frederik Kratzert
Hoshin Gupta
Paul Ullrich
Hydrological Processes (2023)
Preview abstract
It has been proposed that conservation laws might not be beneficial for accurate hydrological modelling due to errors in input (precipitation) and target (streamflow) data (particularly at the event time scale), and this might explain why deep learning models (which are not based on enforcing closure) can out-perform catchment-scale conceptual and process-based models at predicting streamflow. We test this hypothesis with two forcing datasets that disagree in total, long-term precipitation. We analyse the roll of strictly enforced mass conservation for matching a long-term mass balance between precipitation input and streamflow output using physics-informed (mass conserving) machine learning and find that: (1) enforcing closure in the rainfall-runoff mass balance does appear to harm the overall skill of hydrological models; (2) deep learning models learn to account for spatiotemporally variable biases in data (3) however this ‘closure’ effect accounts for only a small fraction of the difference in predictive skill between deep learning and conceptual models.
View details
Cross Modal Distillation for Flood Extent Mapping
Shubhika Garg
Ben Feinstein
Shahar Timnat
Gideon Dror
Adi Gerzi Rosenthal
Tackling Climate Change with Machine Learning, NeurIPS 2022 Workshop
Preview abstract
The increasing intensity and frequency of floods is one of the many consequences of our changing climate. In this work, we explore ML techniques that improve the flood detection module of an operational early flood warning system. Our method exploits an unlabelled dataset of paired multi-spectral and Synthetic Aperture Radar (SAR) imagery to reduce the labeling requirements of a purely supervised learning method. Past attempts have used such unlabelled data by creating weak labels out of them, but end up learning the label mistakes in those weak labels. Motivated by knowledge distillation and semi supervised learning, we explore the use of a teacher to train a student with the help of a small hand labeled dataset and a large unlabelled dataset. Unlike the conventional self distillation setup, we propose a cross modal distillation framework that transfers supervision from a teacher trained on richer modality (multi-spectral images) to a student model trained on SAR imagery. The trained models are then tested on the Sen1Floods11 dataset. Our model outperforms the Sen1Floods11 SAR baselines by an absolute margin of 4.15% pixel wise Intersection-over-Union (IoU) on the test split.
View details
Flood forecasting with machine learning models in an operational framework
Asher Metzger
Chen Barshai
Dana Weitzner
Frederik Kratzert
Gregory Begelman
Guy Shalev
Hila Noga
Moriah Royz
Niv Giladi
Ronnie Maor
Sella Nevo
Yotam Gigi
Zvika Ben-Haim
HESS (2022)
Preview abstract
Google’s operational flood forecasting system was developed to provide accurate real-time flood warnings to agencies and the public, with a focus on riverine floods in large, gauged rivers. It became operational in 2018 and has since expanded geographically. This forecasting system consists of four subsystems: data validation, stage forecasting, inundation modeling, and alert distribution. Machine learning is used for two of the subsystems. Stage forecasting is modeled with the Long Short-Term Memory (LSTM) networks and the Linear models. Flood inundation is computed with the Thresholding and the Manifold models, where the former computes inundation extent and the latter computes both inundation extent and depth. The Manifold model, presented here for the first time, provides a machine-learning alternative to hydraulic modeling of flood inundation. When evaluated on historical data, all models achieve sufficiently high-performance metrics for operational use. The LSTM showed higher skills than the Linear model, while the Thresholding and Manifold models achieved similar performance metrics for modeling inundation extent. During the 2021 monsoon season, the flood warning system was operational in India and Bangladesh, covering flood-prone regions around rivers with a total area of 287,000 km2, home to more than 350M people. More than 100M flood alerts were sent to affected populations, to relevant authorities, and to emergency organizations. Current and future work on the system includes extending coverage to additional flood-prone locations, as well as improving modeling capabilities and accuracy.
View details
The Great Lakes Runoff Intercomparison Project Phase 4: The Great Lakes (GRIP-GL)
Juliane Mai
Hongren Shen
Bryan A. Tolson
Étienne Gaborit
Richard Arsenault
James R. Craig
Vincent Fortin
Lauren M. Fry
Martin Gauch
Daniel Klotz
Frederik Kratzert
Nicole O’Brien
Daniel G. Princz
Sinan Rasiya Koya
Tirthankar Roy
Frank Seglenieks
Narayan K. Shrestha
André G. T. Temgoua
Vincent Vionnet
Jonathan W. Waddell
Hydrology and Earth System Sciences, 26 (2022), 3537–3572
Preview abstract
Model intercomparison studies are carried out to test and compare the simulated outputs of various model setups over the same study domain. The Great Lakes region is such a domain of high public interest as it not only resembles a challenging region to model with its transboundary location, strong lake effects, and regions of strong human impact but is also one of the most densely populated areas in the USA and Canada. This study brought together a wide range of researchers setting up their models of choice in a highly standardized experimental setup using the same geophysical datasets, forcings, common routing product, and locations of performance evaluation across the 1×106 km2 study domain. The study comprises 13 models covering a wide range of model types from machine-learning-based, basin-wise, subbasin-based, and gridded models that are either locally or globally calibrated or calibrated for one of each of the six predefined regions of the watershed. Unlike most hydrologically focused model intercomparisons, this study not only compares models regarding their capability to simulate streamflow (Q) but also evaluates the quality of simulated actual evapotranspiration (AET), surface soil moisture (SSM), and snow water equivalent (SWE). The latter three outputs are compared against gridded reference datasets. The comparisons are performed in two ways – either by aggregating model outputs and the reference to basin level or by regridding all model outputs to the reference grid and comparing the model simulations at each grid-cell.
The main results of this study are as follows:
1. The comparison of models regarding streamflow reveals the superior quality of the machine-learning-based model in the performance of all experiments; even for the most challenging spatiotemporal validation, the machine learning (ML) model outperforms any other physically based model.
2. While the locally calibrated models lead to good performance in calibration and temporal validation (even outperforming several regionally calibrated models), they lose performance when they are transferred to locations that the model has not been calibrated on. This is likely to be improved with more advanced strategies to transfer these models in space.
3. The regionally calibrated models – while losing less performance in spatial and spatiotemporal validation than locally calibrated models – exhibit low performances in highly regulated and urban areas and agricultural regions in the USA.
4. Comparisons of additional model outputs (AET, SSM, and SWE) against gridded reference datasets show that aggregating model outputs and the reference dataset to the basin scale can lead to different conclusions than a comparison at the native grid scale. The latter is deemed preferable, especially for variables with large spatial variability such as SWE.
5. A multi-objective-based analysis of the model performances across all variables (Q, AET, SSM, and SWE) reveals overall well-performing locally calibrated models (i.e., HYMOD2-lumped) and regionally calibrated models (i.e., MESH-SVS-Raven and GEM-Hydro-Watroute) due to varying reasons. The machine-learning-based model was not included here as it is not set up to simulate AET, SSM, and SWE.
6. All basin-aggregated model outputs and observations for the model variables evaluated in this study are available on an interactive website that enables users to visualize results and download the data and model outputs.
View details