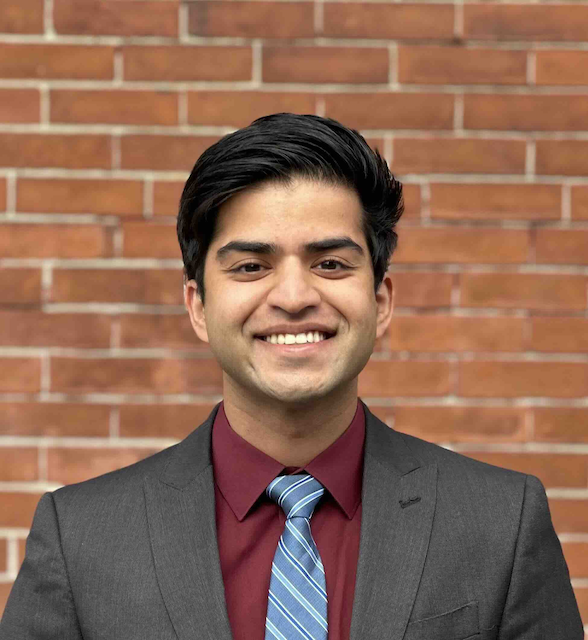
Anil Palepu
Anil Palepu is a Research Scientist at Google Research, where he works on developing LLM-based systems for medical and biomedical applications. Most notably, he is a core contributor to the AMIE project, conducting research towards a conversational, multimodal, empathic and diagnostic medical intelligence. He previously completed his PhD at Harvard-MIT Health Sciences & Technology, advised by Dr. Andrew Beam, as well as his Bachelors and Masters in biomedical engineering at Johns Hopkins University.
Research Areas
Authored Publications
Sort By
Towards Conversational AI for Disease Management
Khaled Saab
David Stutz
Kavita Kulkarni
Sara Mahdavi
Joelle Barral
James Manyika
Ryutaro Tanno
Adam Rodman
arXiv (2025)
Preview abstract
While large language models (LLMs) have shown promise in diagnostic dialogue, their capabilities for effective management reasoning - including disease progression, therapeutic response, and safe medication prescription - remain under-explored. We advance the previously demonstrated diagnostic capabilities of the Articulate Medical Intelligence Explorer (AMIE) through a new LLM-based agentic system optimised for clinical management and dialogue, incorporating reasoning over the evolution of disease and multiple patient visit encounters, response to therapy, and professional competence in medication prescription. To ground its reasoning in authoritative clinical knowledge, AMIE leverages Gemini's long-context capabilities, combining in-context retrieval with structured reasoning to align its output with relevant and up-to-date clinical practice guidelines and drug formularies. In a randomized, blinded virtual Objective Structured Clinical Examination (OSCE) study, AMIE was compared to 21 primary care physicians (PCPs) across 100 multi-visit case scenarios designed to reflect UK NICE Guidance and BMJ Best Practice guidelines. AMIE was non-inferior to PCPs in management reasoning as assessed by specialist physicians and scored better in both preciseness of treatments and investigations, and in its alignment with and grounding of management plans in clinical guidelines. To benchmark medication reasoning, we developed RxQA, a multiple-choice question benchmark derived from two national drug formularies (US, UK) and validated by board-certified pharmacists. While AMIE and PCPs both benefited from the ability to access external drug information, AMIE outperformed PCPs on higher difficulty questions. While further research would be needed before real-world translation, AMIE's strong performance across evaluations marks a significant step towards conversational AI as a tool in disease management.
View details
Towards Conversational Diagnostic AI
Khaled Saab
Jan Freyberg
Ryutaro Tanno
Amy Wang
Brenna Li
Nenad Tomašev
Karan Singhal
Le Hou
Albert Webson
Kavita Kulkarni
Sara Mahdavi
Juro Gottweis
Joelle Barral
Kat Chou
Arxiv (2024) (to appear)
Preview abstract
At the heart of medicine lies the physician-patient dialogue, where skillful history-taking paves the way for accurate diagnosis, effective management, and enduring trust. Artificial Intelligence (AI) systems capable of diagnostic dialogue could increase accessibility, consistency, and quality of care. However, approximating clinicians' expertise is an outstanding grand challenge. Here, we introduce AMIE (Articulate Medical Intelligence Explorer), a Large Language Model (LLM) based AI system optimized for diagnostic dialogue.
AMIE uses a novel self-play based simulated environment with automated feedback mechanisms for scaling learning across diverse disease conditions, specialties, and contexts. We designed a framework for evaluating clinically-meaningful axes of performance including history-taking, diagnostic accuracy, management reasoning, communication skills, and empathy. We compared AMIE's performance to that of primary care physicians (PCPs) in a randomized, double-blind crossover study of text-based consultations with validated patient actors in the style of an Objective Structured Clinical Examination (OSCE). The study included 149 case scenarios from clinical providers in Canada, the UK, and India, 20 PCPs for comparison with AMIE, and evaluations by specialist physicians and patient actors. AMIE demonstrated greater diagnostic accuracy and superior performance on 28 of 32 axes according to specialist physicians and 24 of 26 axes according to patient actors. Our research has several limitations and should be interpreted with appropriate caution. Clinicians were limited to unfamiliar synchronous text-chat which permits large-scale LLM-patient interactions but is not representative of usual clinical practice. While further research is required before AMIE could be translated to real-world settings, the results represent a milestone towards conversational diagnostic AI.
View details
Towards Generalist Biomedical AI
Danny Driess
Andrew Carroll
Chuck Lau
Ryutaro Tanno
Ira Ktena
Basil Mustafa
Aakanksha Chowdhery
Simon Kornblith
Philip Mansfield
Sushant Prakash
Renee Wong
Sunny Virmani
Sara Mahdavi
Bradley Green
Ewa Dominowska
Joelle Barral
Karan Singhal
Pete Florence
NEJM AI (2024)
Preview abstract
BACKGROUND: Medicine is inherently multimodal, requiring the simultaneous interpretation and integration of insights between many data modalities spanning text, imaging, genomics, and more. Generalist biomedical artificial intelligence systems that flexibly encode, integrate, and interpret these data might better enable impactful applications ranging from scientific discovery to care delivery.
METHODS: To catalyze development of these models, we curated MultiMedBench, a new multimodal biomedical benchmark. MultiMedBench encompasses 14 diverse tasks, such as medical question answering, mammography and dermatology image interpretation, radiology report generation and summarization, and genomic variant calling. We then introduced Med-PaLM Multimodal (Med-PaLM M), our proof of concept for a generalist biomedical AI system that flexibly encodes and interprets biomedical data including clinical language, imaging, and genomics with the same set of model weights. To further probe the capabilities and limitations of Med-PaLM M, we conducted a radiologist evaluation of model-generated (and human) chest x-ray reports.
RESULTS: We observed encouraging performance across model scales. Med-PaLM M reached performance competitive with or exceeding the state of the art on all MultiMedBench tasks, often surpassing specialist models by a wide margin. In a side-by-side ranking on 246 retrospective chest x-rays, clinicians expressed a pairwise preference for Med-PaLM Multimodal reports over those produced by radiologists in up to 40.50% of cases, suggesting potential clinical utility.
CONCLUSIONS: Although considerable work is needed to validate these models in real-world cases and understand if cross-modality generalization is possible, our results represent a milestone toward the development of generalist biomedical artificial intelligence systems.
View details
Towards Accurate Differential Diagnosis with Large Language Models
Daniel McDuff
Amy Wang
Karan Singhal
Yash Sharma
Kavita Kulkarni
Le Hou
Sara Mahdavi
Sushant Prakash
Anupam Pathak
Shwetak Patel
Ewa Dominowska
Juro Gottweis
Joelle Barral
Kat Chou
Jake Sunshine
Arxiv (2023)
Preview abstract
An accurate differential diagnosis (DDx) is a cornerstone of medical care, often reached through an iterative process of interpretation that combines clinical history, physical examination, investigations and procedures. Interactive interfaces powered by Large Language Models (LLMs) present new opportunities to both assist and automate aspects of this process. In this study, we introduce an LLM optimized for diagnostic reasoning, and evaluate its ability to generate a DDx alone or as an aid to clinicians. 20 clinicians evaluated 302 challenging, real-world medical cases sourced from the New England Journal of Medicine (NEJM) case reports. Each case report was read by two clinicians, who were randomized to one of two assistive conditions: either assistance from search engines and standard medical resources, or LLM assistance in addition to these tools. All clinicians provided a baseline, unassisted DDx prior to using the respective assistive tools. Our LLM for DDx exhibited standalone performance that exceeded that of unassisted clinicians (top-10 accuracy 59.1% vs 33.6%, [p = 0.04]). Comparing the two assisted study arms, the DDx quality score was higher for clinicians assisted by our LLM (top-10 accuracy 51.7%) compared to clinicians without its assistance (36.1%) (McNemar's Test: 45.7, p < 0.01) and clinicians with search (44.4%) (4.75, p = 0.03). Further, clinicians assisted by our LLM arrived at more comprehensive differential lists than those without its assistance. Our study suggests that our LLM for DDx has potential to improve clinicians' diagnostic reasoning and accuracy in challenging cases, meriting further real-world evaluation for its ability to empower physicians and widen patients' access to specialist-level expertise.
View details