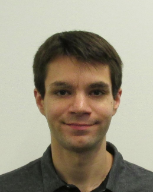
Jonathan Krause
Research Areas
Authored Publications
Sort By
Safety principles for medical summarization using generative AI
Dillon Obika
Christopher Kelly
Nicola Ding
Chris Farrance
Praney Mittal
Donny Cheung
Heather Cole-Lewis
Madeleine Elish
Nature Medicine (2024)
Preview abstract
The introduction of Generative AI, particularly large language models presents exciting opportunities for healthcare. However their novel capabilities also have the potential to introduce novel risks and hazards. This paper explores the unique safety challenges associated with LLMs in healthcare, using medical text summarization as a motivating example. Using MedLM as a case example, we propose leveraging existing standards and guidance while developing novel approaches tailored to the specific characteristics of LLMs.
View details
A deep learning model for novel systemic biomarkers in photographs of the external eye: a retrospective study
Ilana Traynis
Christina Chen
Akib Uddin
Jorge Cuadros
Lauren P. Daskivich
April Y. Maa
Ramasamy Kim
Eugene Yu-Chuan Kang
Lily Peng
Avinash Varadarajan
The Lancet Digital Health (2023)
Preview abstract
Background
Photographs of the external eye were recently shown to reveal signs of diabetic retinal disease and elevated glycated haemoglobin. This study aimed to test the hypothesis that external eye photographs contain information about additional systemic medical conditions.
Methods
We developed a deep learning system (DLS) that takes external eye photographs as input and predicts systemic parameters, such as those related to the liver (albumin, aspartate aminotransferase [AST]); kidney (estimated glomerular filtration rate [eGFR], urine albumin-to-creatinine ratio [ACR]); bone or mineral (calcium); thyroid (thyroid stimulating hormone); and blood (haemoglobin, white blood cells [WBC], platelets). This DLS was trained using 123 130 images from 38 398 patients with diabetes undergoing diabetic eye screening in 11 sites across Los Angeles county, CA, USA. Evaluation focused on nine prespecified systemic parameters and leveraged three validation sets (A, B, C) spanning 25 510 patients with and without diabetes undergoing eye screening in three independent sites in Los Angeles county, CA, and the greater Atlanta area, GA, USA. We compared performance against baseline models incorporating available clinicodemographic variables (eg, age, sex, race and ethnicity, years with diabetes).
Findings
Relative to the baseline, the DLS achieved statistically significant superior performance at detecting AST >36.0 U/L, calcium <8.6 mg/dL, eGFR <60.0 mL/min/1.73 m2, haemoglobin <11.0 g/dL, platelets <150.0 × 103/μL, ACR ≥300 mg/g, and WBC <4.0 × 103/μL on validation set A (a population resembling the development datasets), with the area under the receiver operating characteristic curve (AUC) of the DLS exceeding that of the baseline by 5.3–19.9% (absolute differences in AUC). On validation sets B and C, with substantial patient population differences compared with the development datasets, the DLS outperformed the baseline for ACR ≥300.0 mg/g and haemoglobin <11.0 g/dL by 7.3–13.2%.
Interpretation
We found further evidence that external eye photographs contain biomarkers spanning multiple organ systems. Such biomarkers could enable accessible and non-invasive screening of disease. Further work is needed to understand the translational implications.
View details
Lessons learned from translating AI from development to deployment in healthcare
Sunny Virmani
Jay Nayar
Elin Rønby Pedersen
Divleen Jeji
Lily Peng
Nature Medicine (2023)
Preview abstract
The application of an artificial intelligence (AI)-based screening tool for retinal disease in India and Thailand highlighted the myths and reality of introducing medical AI, which may form a framework for subsequent tools.
View details
Domain-specific optimization and diverse evaluation of self-supervised models for histopathology
Jeremy Lai
Supriya Vijay
Jessica Loo
Saurabh Vyawahare
Saloni Agarwal
Fayaz Jamil
Cameron Chen
arXiv (2023)
Preview abstract
Task-specific deep learning models in histopathology offer promising opportunities for improving diagnosis, clinical research, and precision medicine. However, development of such models is often limited by availability of high-quality data. Foundation models in histopathology that learn general representations across a wide range of tissue types, diagnoses, and magnifications offer the potential to reduce the data, compute, and technical expertise necessary to develop task-specific deep learning models with the required level of model performance. In this work, we describe the development and evaluation of foundation models for histopathology via self-supervised learning (SSL). We first establish a diverse set of benchmark tasks involving 17 unique tissue types and 12 unique cancer types and spanning different optimal magnifications and task types. Next, we use this benchmark to explore and evaluate histopathology-specific SSL methods followed by further evaluation on held out patch-level and weakly supervised tasks. We found that standard SSL methods thoughtfully applied to histopathology images are performant across our benchmark tasks and that domain-specific methodological improvements can further increase performance. Our findings reinforce the value of using domain-specific SSL methods in pathology, and establish a set of high quality foundation models to enable further research across diverse applications.
View details
Performance of a Diabetic Retinopathy Artificial Intelligence Algorithm for Ultra-widefield Imaging
Tunde Peto
Lloyd Aiello
Srinivas R Sadda
Drew Lewis
Anne Marie Cairns
Dana Keane
Sunny Virmani
Jerry Cavallerano
Barba Hamill
Lily Peng
Sara Ellen Godek
Lu Yang
Naho Kitade
Kira Whitehouse
ARVO (2022)
Preview abstract
Purpose: To evaluate the performance of a deep learning model for diabetic retinopathy (DR) and diabetic macular edema screening when using ultra-widefield (UWF) imaging.
Methods: For model development, 67,200 UWF images were collected from DR programs and ophthalmology clinics worldwide. 30,836 images were double graded and adjudicated at 8 grading centres by 125 certified graders using ETDRS extension of the Modified Airlie House Classification of Diabetic Retinopathy following the JVN Clinical Trial Ultrawide Field Grading Manual v1.0. The grading system used traditional ETDRS 7-SF field definition as well as extended fields 3-7 to evaluate the retinal periphery. A further 36,364 UWF images were graded using a grading protocol based on the ICDR classification. The dataset was split into training, tuning and testing. The final DR model is an ensemble of 10 EfficientNet-b0 neural networks, independently trained with standard image augmentation techniques. For model validation, two independent sets of images were collected. Model performance was evaluated by comparing its predictions to the adjudicated ground truth for both sets of images.
Results: Prior to clinical validation, the model performance was internally evaluated on an independent set of 1967 images, of which 1050 were graded via adjudication as negative for more than mild diabetic retinopathy (mtmDR negative), and 917 as having referable diabetic retinopathy (mtmDR positive). The overall performance (Table 1) was weighted by target DR distribution. Clinical validation evaluated an independent data set of 420 images selected to achieve a target distribution that enabled appropriate confidence intervals for mtmDR sensitivity and specificity A panel of three graders adjudicated these 420 images and assessed 241 as mtmDR negative, 179 as mtmDR positive and 135 as vtDR positive. Model’s performance on the clinical validation set is shown in Table 2.
Conclusions: The deep learning model was developed with high quality graded UWF images and performed at a level that highly suggests usefulness in a clinical screening setting. A large, prospective multi-center clinical trial is currently evaluating the performance of a similar model in a real-world clinical setting.
This abstract was presented at the 2022 ARVO Annual Meeting, held in Denver, CO, May 1-4, 2022, and virtually.
View details
Longitudinal Screening for Diabetic Retinopathy in a Nationwide Screening Program: Comparing Deep Learning and Human Graders
Jirawut Limwattanayingyong
Variya Nganthavee
Kasem Seresirikachorn
Tassapol Singalavanija
Ngamphol Soonthornworasiri
Varis Ruamviboonsuk
Chetan Rao
Rajiv Raman
Andrzej Grzybowski
Lily Hao Yi Peng
Fred Hersch
Richa Tiwari, PhD
Dr. Paisan Raumviboonsuk
Journal of Diabetes Research (2020)
Preview abstract
Objective.
To evaluate diabetic retinopathy (DR) screening via deep learning (DL) and trained human graders (HG) in a longitudinal cohort, as case spectrum shifts based on treatment referral and new-onset DR.
Methods.
We randomly selected patients with diabetes screened twice, two years apart within a nationwide screening program. The reference standard was established via adjudication by retina specialists. Each patient’s color fundus photographs were graded, and a patient was considered as having sight-threatening DR (STDR) if the worse eye had severe nonproliferative DR, proliferative DR, or diabetic macular edema. We compared DR screening via two modalities: DL and HG. For each modality, we simulated treatment referral by excluding patients with detected STDR from the second screening using that modality.
Results.
There were 5,738 patients (12.3% STDR) in the first screening. DL and HG captured different numbers of STDR cases, and after simulated referral and excluding ungradable cases, 4,148 and 4,263 patients remained in the second screening, respectively. The STDR prevalence at the second screening was 5.1% and 6.8% for DL- and HG-based screening, respectively. Along with the prevalence decrease, the sensitivity for both modalities decreased from the first to the second screening (DL: from 95% to 90%, p=0.008; HG: from 74% to 57%, p<0.001). At both the first and second screenings, the rate of false negatives for the DL was a fifth that of HG (0.5-0.6% vs. 2.9-3.2%).
Conclusion.
On 2-year longitudinal follow-up of a DR screening cohort, STDR prevalence decreased for both DL- and HG-based screening. Follow-up screenings in longitudinal DR screening can be more difficult and induce lower sensitivity for both DL and HG, though the false negative rate was substantially lower for DL. Our data may be useful for health-economics analyses of longitudinal screening settings.
View details
Preview abstract
In recent years, many new clinical diagnostic tools have been developed using complicated machine learning methods. Irrespective of how a diagnostic tool is derived, it must be evaluated using a 3-step process of deriving, validating, and establishing the clinical effectiveness of the tool. Machine learning–based tools should also be assessed for the type of machine learning model used and its appropriateness for the input data type and data set size. Machine learning models also generally have additional prespecified settings called hyperparameters, which must be tuned on a data set independent of the validation set. On the validation set, the outcome against which the model is evaluated is termed the reference standard. The rigor of the reference standard must be assessed, such as against a universally accepted gold standard or expert grading.
View details
Deep learning versus human graders for classifying diabetic retinopathy severity in a nationwide screening program
Dr. Paisan Raumviboonsuk
Dr. Peranut Chotcomwongse
Rajiv Raman
Sonia Phene
Kornwipa Hemarat
Mongkol Tadarati
Sukhum Silpa-Archa
Jirawut Limwattanayingyong
Chetan Rao
Oscar Kuruvilla
Jesse Jung
Jeffrey Tan
Surapong Orprayoon
Chawawat Kangwanwongpaisan
Ramase Sukumalpaiboon
Chainarong Luengchaichawang
Jitumporn Fuangkaew
Pipat Kongsap
Lamyong Chualinpha
Sarawuth Saree
Srirut Kawinpanitan
Korntip Mitvongsa
Siriporn Lawanasakol
Chaiyasit Thepchatri
Lalita Wongpichedchai
Lily Peng
Nature Partner Journal (npj) Digital Medicine (2019)
Preview abstract
Deep learning algorithms have been used to detect diabetic retinopathy (DR) with specialist-level accuracy. This study aims to validate one such algorithm on a large-scale clinical population, and compare the algorithm performance with that of human graders. A total of 25,326 gradable retinal images of patients with diabetes from the community-based, nationwide screening program of DR in Thailand were analyzed for DR severity and referable diabetic macular edema (DME). Grades adjudicated by a panel of international retinal specialists served as the reference standard. Relative to human graders, for detecting referable DR (moderate NPDR or worse), the deep learning algorithm had significantly higher sensitivity (0.97 vs. 0.74, p < 0.001), and a slightly lower specificity (0.96 vs. 0.98, p < 0.001). Higher sensitivity of the algorithm was also observed for each of the categories of severe or worse NPDR, PDR, and DME (p < 0.001 for all comparisons). The quadratic-weighted kappa for determination of DR severity levels by the algorithm and human graders was 0.85 and 0.78 respectively (p < 0.001 for the difference). Across different severity levels of DR for determining referable disease, deep learning significantly reduced the false negative rate (by 23%) at the cost of slightly higher false positive rates (2%). Deep learning algorithms may serve as a valuable tool for DR screening.
View details
Using a deep learning algorithm and integrated gradient explanation to assist grading for diabetic retinopathy
Ankur Taly
Anthony Joseph
Arjun Sood
Arun Narayanaswamy
Derek Wu
Ehsan Rahimy
Jesse Smith
Katy Blumer
Lily Peng
Michael Shumski
Scott Barb
Zahra Rastegar
Ophthalmology (2019)
Preview abstract
Background Deep learning methods have recently produced algorithms that can detect disease such as diabetic retinopathy (DR) with doctor-level accuracy. We sought to understand the impact of these models on physician graders in assisted-read settings.
Methods We surfaced model predictions and explanation maps ("masks") to 9 ophthalmologists with varying levels of experience to read 1,804 images each for DR severity based on the International Clinical Diabetic Retinopathy (ICDR) disease severity scale. The image sample was representative of the diabetic screening population, and was adjudicated by 3 retina specialists for a reference standard. Doctors read each image in one of 3 conditions: Unassisted, Grades Only, or Grades+Masks.
Findings Readers graded DR more accurately with model assistance than without (p < 0.001, logistic regression). Compared to the adjudicated reference standard, for cases with disease, 5-class accuracy was 57.5% for the model. For graders, 5-class accuracy for cases with disease was 47.5 ± 5.6% unassisted, 56.9 ± 5.5% with Grades Only, and 61.5 ± 5.5% with Grades+Mask. Reader performance improved with assistance across all levels of DR, including for severe and proliferative DR. Model assistance increased the accuracy of retina fellows and trainees above that of the unassisted grader or model alone. Doctors’ grading confidence scores and read times both increased overall with assistance. For most cases, Grades + Masks was as only effective as Grades Only, though masks provided additional benefit over grades alone in cases with: some DR and low model certainty; low image quality; and proliferative diabetic retinopathy (PDR) with features that were frequently missed, such as panretinal photocoagulation (PRP) scars.
Interpretation Taken together, these results show that deep learning models can improve the accuracy of, and confidence in, DR diagnosis in an assisted read setting.
View details
Remote Tool-based Adjudication for Grading Diabetic Retinopathy
Tayyeba Ali
Brian Basham
Will Chen
Xiang Ji
Lily Peng
Edith Law
Translational Vision Science & Technology (TVST) (2019)
Preview abstract
Purpose: To present and evaluate a remote, tool-based system and structured grading rubric for adjudicating image-based diabetic retinopathy (DR) grades.
Methods: We compared three different procedures for adjudicating DR severity assessments among retina specialist panels, including (1) in-person adjudication based on a previously described procedure (Baseline), (2) remote, tool-based adjudication for assessing DR severity alone (TA), and (3) remote, tool-based adjudication using a feature-based rubric (TA-F). We developed a system allowing graders to review images remotely and asynchronously. For both TA and TA-F approaches, images with disagreement were reviewed by all graders in a round-robin fashion until disagreements were resolved. Five panels of three retina specialists each adjudicated a set of 499 retinal fundus images (1 panel using Baseline, 2 using TA, and 2 using TA-F adjudication). Reliability was measured as grade agreement among the panels using Cohen's quadratically weighted kappa. Efficiency was measured as the number of rounds needed to reach a consensus for tool-based adjudication.
Results: The grades from remote, tool-based adjudication showed high agreement with the Baseline procedure, with Cohen's kappa scores of 0.948 and 0.943 for the two TA panels, and 0.921 and 0.963 for the two TA-F panels. Cases adjudicated using TA-F were resolved in fewer rounds compared with TA (P < 0.001; standard permutation test).
Conclusions: Remote, tool-based adjudication presents a flexible and reliable alternative to in-person adjudication for DR diagnosis. Feature-based rubrics can help accelerate consensus for tool-based adjudication of DR without compromising label quality.
Translational Relevance: This approach can generate reference standards to validate automated methods, and resolve ambiguous diagnoses by integrating into existing telemedical workflows.
View details