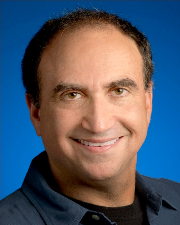
Yossi Matias
Under Yossi’s leadership, world-class global teams are leading breakthrough research on Foundational Machine Learning & Algorithms, Computing Systems & Quantum, Science, AI for Societal Impact in Health, Climate, Sustainability, Education and Socio-technical research, and advancement of Generative AI - driving real-world impact and shaping the future of technology.
Yossi was previously on Google Search leadership for over a decade, driving strategic features and technologies, and pioneered Conversational AI innovations to help transform the phone experience and help remove barriers of modality and languages. He was also the founding lead of Google center in Israel and supported other global sites. During his tenure at Google Yossi founded and spearheaded initiatives such as Google's AI for Social Good, Crisis Response, Google for Startups Accelerator, social and cultural initiatives seeding Google Arts & Culture, and programs fostering startups, sustainability, and STEM and AI literacy for youth.
Prior to Google Yossi was on the Computer Science faculty at Tel Aviv University, a visiting professor at Stanford, and a Research Scientist at Bell Labs. He’s published over 150 papers and is the inventor of over 75 patents. He pioneered some of the early technologies for internet privacy, contextual search, and the effective analysis of Big Data. He is a recipient of the Gödel Prize, an ACM Fellow, and a recipient of the ACM Kanellakis Theory and Practice Award for seminal work on streaming algorithms, data sketches, and large-scale data analytics
Yossi has a track record of impact-driven breakthrough research, innovation, advancing society-centered AI to help address global challenges and extensive product leadership. He is committed to advancing research and AI to help improve lives, transform society, and create a better future for all.
--------------------------------------------------
More about some of Yossi's work over the past years:
His team's work on Google’s Health AI is driving AI research to help transform healthcare, and help make healthcare more accessible for everyone, with multiple breakthroughs including Med-PaLM. Leadership of AI for Climate and Sustainability, work on climate crisis mitigation (e.g., Greenlight) as well as adaptation - with leadership work on Google’s Crisis Response initiative (SOS alerts, flood forecasting, wildfire detection).
Yossi's work on Generative AI includes efforts on Factuality (inc leading to Bard’s Double Check) and Efficiency (Speculative Decoding). He pioneered Conversational AI innovations towards ambient intelligence to help transforming the phone experience (Google Duplex, Call Screen, Hold for Me) and help remove barriers of modality and languages (Live Caption, Live Relay, Euphonia, Read Aloud).
He is a founding lead of Google’s AI for Social Good, Google for Startup Accelerator (with particular focus on AI & ML), Startups for Sustainable Development and Mind the Gap. He pioneered an initiative of bringing online hundreds of heritage collections (including the Dead Sea Scrolls), and helped establish Google’s Cultural Institute.
His work on Search included Autocomplete, Google Trends, Search Console, and Search experiences in weather, sports, dictionary and more. He founded and has lead Google’s center in Israel, through growth to over 2500 on staff, supported Google’s growth (4X) in Bangalore, India, and oversaw Google’s Expanding Research Center in Africa.
Yossi is an ACM Fellow for contributions to the analysis of large data sets and data streams. His foundational work on data streams, data synopses and sketches, motivated by computational challenges in what was then the world’s largest data warehouses, was recognized with the the Gödel Prize in Theoretical Computer Science, and with the ACM Kanellakis Theory and Practice Award for the instrumental role it played "in the development of the field of streaming algorithms, which is one of the most prolific and highly regarded areas of data management research" and its broad applicability to large-scale data analytics.
Research Areas
-
Algorithms and Theory
-
Data Management
-
Data Mining and Modeling
-
Distributed Systems and Parallel Computing
-
Education Innovation
-
Health & Bioscience
-
Human-Computer Interaction and Visualization
-
Information Retrieval and the Web
-
Machine Intelligence
-
Natural Language Processing
-
Security, Privacy and Abuse Prevention
-
Speech Processing